Global Site
Displaying present location in the site.
How AI Is Transforming Financial Services
The era of artificial intelligence (AI) is upon us. The impact of AI and automation technologies on our work and daily lives is more pervasive than many of us realize. The financial industry is no exception. In fact, financial institutions are pioneering the application of AI in business, with utilization of AI expanding at an exponential rate. This paper describes the financial systems to which AI can be applied and shows how powerful AI systems can be built with NEC the WISE - a suite of AI technologies developed by NEC.
1.Introduction
The 21st century has seen accelerating growth in digitized financial services in the Japanese financial industry, with Internet transactions being the most prominent. Driven by the explosive popularization of the Internet and the trend towards financial deregulation, this trend has helped reshape customer relations as customer contact shifts from traditional face-to-face contact to interactive contact using web-based systems where no human intervention is involved. This period has also seen the evolution of Internet-only financial institutions. Today, even advanced loan services such as housing loans - from initial application to conclusion of contract - can be processed entirely on the Internet with no need at all for the customer to show up at a brick-and-mortar office. Similarly, life insurance and other types of insurance can now be applied for and purchased from a smartphone.
This digitization of financial transactions has led to the steady accumulation of massive amounts of financial and personal data. Today, the financial industry is actively seeking ways to leverage this data to deliver new and improved services. This, in turn, requires that data processing efficiency be improved in order to process all of this data quickly and accurately. This is a task ideal for AI. Already, AI is becoming indispensable - for example, data-based customer analysis is now usually conducted by AI, whereas in the past such scrutiny was usually carried out face-to-face and depended largely on the representative’s intuition and perceptions. Generally, it is becoming more and more common to submit any time-consuming analysis task to AI for high-speed analysis.
1.1 Areas Where AI is Applicable
In fiscal 2016 alone, installed AI applications at our financial institution clients, together with prior validation experiments and studies, totaled more than 100 cases. Although AI is being applied in more and more fi elds, the areas where we have worked on AI can be roughly classified into the following five categories.
(1) Screening
Creation of credit models for screening business loan applications, credit card loan applications, and housing loan applications, as well as reduction of clerical workload.
(2) Fraud detection
Detection of fraud such as fraudulent use of credit cards and cash cards, fraudulent insurance claims, illegal transactions, and transfer scams.
(3) Marketing and forecasting
Numerical value prediction to achieve maximum impact at minimum cost, including promotion prediction, demand prediction, and stock price prediction.
(4) Matching and recommendation
Creation of opportunities such as aptitude assessment for human resource management and recruitment, M&A recommendations, investment advice (robo-advisors), and product purchase recommendations.
(5) Collection and analysis of large volumes of data
Visualization such as analysis of customer comments directed to contact centers, automation of help desks, social data on social media, and analysis of news articles.
AI is capable of rapidly absorbing know-how and knowledge that takes humans many years to accumulate. It is precisely for this reason that AI is being increasingly applied in financial institutions.
2.AI Technology
What are the AI technologies that we use to support and develop the applications outlined above? In this section, we will take a closer look at NEC the WISE - a suite of AI technologies developed by NEC, three of which are now being applied in financial institution systems.
2.1 NEC’s AI Technology Suite: NEC the WISE
NEC the WISE (Fig. 1) is a suite of cutting-edge AI technologies that maximize human intelligence and creative activities. NEC the WISE represents our commitment to harnessing the wisdom of humans and AI working together to resolve the increasingly complex and intertwined issues society is facing today.
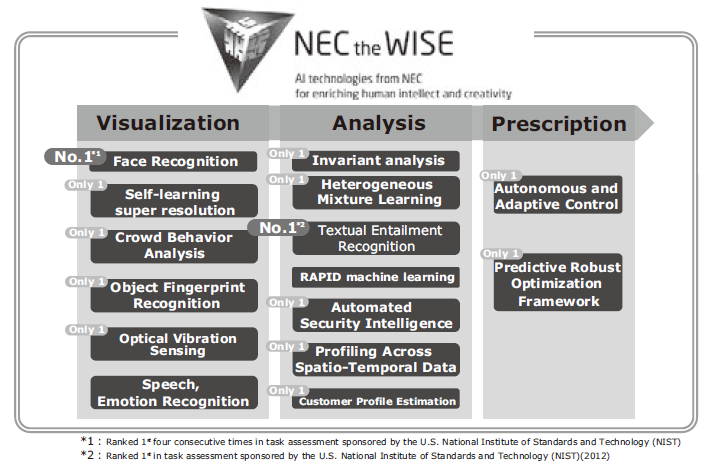
NEC the WISE includes technologies that include the world’s number-one and only-one technologies in image and voice recognition, data analysis, and system control. For example, in the area of visualization, practical applications have already been deployed in areas such as personal identification using face recognition at airports and theme parks, as well as authentication at external terminals at financial institutions also using face recognition. In the area of analysis, forecast and judgment, Heterogeneous Mixture Learning, and RAPID Machine Learning (described below), as well as risk control and marketing using Textual Entailment recognition, have begun to be deployed in real-world applications. In the area of prescription - that is, a system where AI posits a solution based on data analysis and prediction - development towards practical use is rapidly underway.
2.2 Features of NEC’s AI Technologies
In the financial industry, rapid progress in data analysis is anticipated. Here we look at three NEC the WISE technologies that have an especially wide range of potential applications.
(1) RAPID Machine Learning
One of the most promising and best-known AI technologies is RAPID Machine Learning (Fig. 2), a type of software equipped with deep learning technology. When data - whether images, text, and numerical values - are fed into RAPID Machine Learning, it learns while automatically extracting certain characteristics - for example, regularities and patterns - present in the data. Typically, the sheer scale of the computing effort required for deep learning means that it consumes a massive amount of system resources. Compared to other deep learning systems, however, NEC’s RAPID Machine Learning is fast and light weight, which is why we named it “RAPID.”
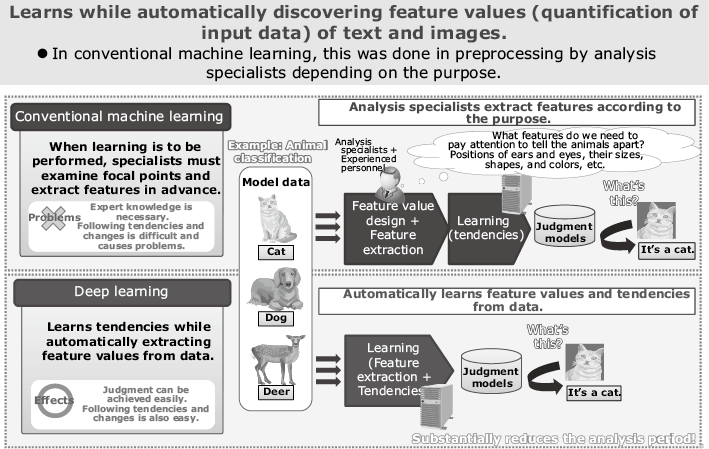
The speed and light weight of this technology means that users can start small with a small-scale system and scale up as required. Moreover, because this software has undergone quality assurance and data is not transmitted to the cloud during installation, it can be safely introduced into an organization’s machine environment even when highly confidential financial data is involved.
(2) Heterogeneous Mixture Learning
Heterogeneous Mixture Learning (Fig. 3) is also a technology that generates prediction models based on the data fed into it. However, there is a big difference. Unlike other machine learning technologies, it not only predicts possible results but also can show the basis of that prediction - something only AI can do. Moreover, by using “data partitioning,” it can even generate prediction models when different irregularities coexist in the data.
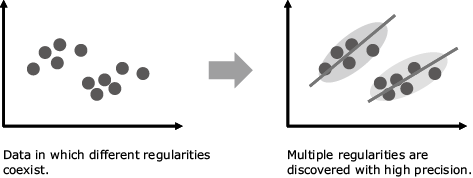
Fig. 3 Heterogeneous Mixture Learning.
For instance, in product sales prediction, different prediction models are used for weekdays and holidays. Heterogeneous Mixture Learning automatically partitions data and derives a prediction model from each “partition.” In addition to prediction, it can also be used to uncover new regularities that would escape human detection.
(3) Textual Entailment
Textual Entailment is a technology that can recognize when two sentences have the same meaning. Using this technology for text search, you can search for sentences that not only have matching keywords but also matching meanings. In the examples shown in Fig. 4, the original sentence says, “I like apples.” Meanwhile, the sentence saying, “He likes apples, but I don’t,” also includes the words “I,” “apples,” and “like” but has a different meaning. Textual Entailment can correctly adjudicate this kind of difference.
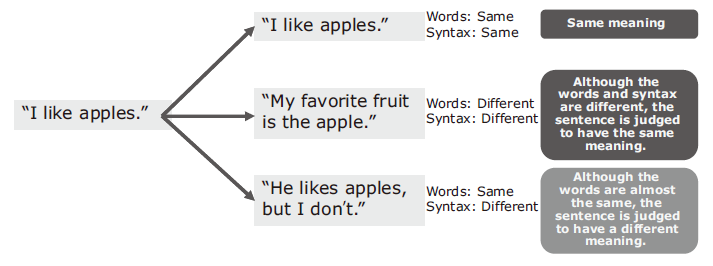
It can also be used to extract the meaning of “liking apples” from a tremendous number of sentences and to classify preferences.
3.Application Examples of AI Technology in Financial Institutions
In this section, we discuss examples of AI technology application in three of the five areas listed in 1.1 above.
(1) Screening
First, let’s look at a case where loan applications are screened using Heterogeneous Mixture Learning. First attribute data is collected on cases where payments were delinquent or the loan fell into default.
Next, the data is fed into the Heterogeneous Mixture Learning system so that it can learn from it. Then, the system automatically generates prediction formulas showing what screening items were defaulted on and under what conditions. Subsequently, when a screening target’s data is fed into these prediction formulas which have been developed using the previously partitioned data, the system can determine whether or not to accept the loan application and provide you with the basis for that judgment. Heterogeneous Mixture Learning can help improve competitiveness by reducing costs and screening time, while its most distinctive aspect - that the basis for its judgment can be known - provides the loan manager with useful reference material when it comes to making a final decision. The foundational trends for these judgments also make it possible to understand where improvements need to be made and can also lead to new product development.
(2) Fraud detection
Now, let’s look at a case where RAPID Machine Learning is used to detect fraudulent financial transactions. RAPID uses the same learning process as Heterogeneous Mixture Learning; it analyzes previous data in order to build a model that will allow it to predict the future. In this case, data on transactions that were found to be fraudulent in the past needs to be collected and analyzed first. When there is not enough learning data - that is, data on transactions that were definitively concluded to be fraudulent - it can be supplemented with data on transactions deemed suspicious by investigators and systems. All of this data is then fed into the RAPID Machine Learning system, which uses multi-layer neural networks to generate prediction models while automatically extracting the patterns and characteristics of fraudulent transactions at a level of precision only deep learning is capable of. When you feed the transaction data that you want the system to adjudicate into these prediction models, it outputs the degree - with score values - to which the data matches the characteristics of fraudulent transactions. The higher the score value the system outputs, the higher the likelihood that the transaction is fraudulent. Compared to the binary judgment using fixed threshold values, this makes it possible to prioritize investigation targets by turning degrees of suspicion into scores. Moreover, when this is combined with unstructured data such as images and text, it also becomes possible to discover new tendencies that have been thus far overlooked. By providing investigator with a powerful and reliable tool for assessing the likelihood of fraud, we anticipate that this system will significantly reduce the difficulty of fraud investigations.
(3) Text analysis
Finally, let’s take a look at a case where comments from customers recorded in contact center histories and entered in questionnaires are to be analyzed. Assuming that words expressing gratitude such as “thank,” “grateful,” and “appreciate” are used as keywords for searching, for example, it is possible that we could find sentences with opposite meanings like, “you didn’t say you were grateful to me.” Excluding hits like this one at a time would be extremely time consuming. However, because Textual Entailment technology is capable of recognizing meanings, it can distinguish a sentence like this from sentences containing the meaning of gratitude and drop it from the results. In this way, sentences with the desired meaning specified can be correctly extracted. The extracted sentences can also be classified into groups such as acknowledgments, claims, and opinions to facilitate analysis.
For example, when Textual Entailment is applied to news sites or social media sites, it can judge whether articles and postings about a certain product have positive or negative connotations and classify and aggregate them accordingly. This makes it possible to achieve almost real-time understanding of customer comments about products and services, helping hasten feedback into services.
4.Evolving AI Technology
While AI technology is increasingly being applied in a wide range of fields, many tasks remain to be addressed. Data analysis with AI requires repeated preparation and processing of data until the system has completed learning. The majority of data in business systems is stored in relational databases. It usually takes experienced experts called data scientists a few months to select data required to obtain the relevant prediction results and to associate relationships between databases.
NEC has solved this problem by developing a new AI technology called Predictive Analytics Automation Technology that automates these advanced and complex pre-processing procedures. It automatically creates combinations of data items required for prediction and also generates queries that take those combinations out of the databases. When we tested the data analysis procedure, we confirmed that a procedure that would have taken two months now took only one day. This automation technology will soon be ready for practical usage.
5.Conclusion
In this paper, we have discussed tasks where AI is applicable in the financial industry and how NEC’s AI technologies achieve those applications. As AI technology continues to evolve at an ever more rapid rate, NEC is committed to shaping and directing that evolution to ensure that society benefits from advanced, easy-to-use technology that makes life simpler and more convenient for everyone.
Reference
Authors' Profiles
FUKUDA Kenji
Project Director
Financial Systems Development Division