Global Site
Displaying present location in the site.
What is Brain-Morphic AI?
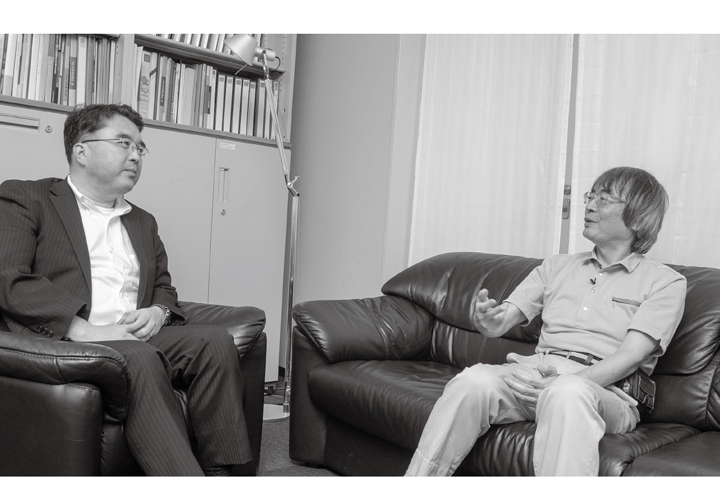
Brain-Morphic AI is a revolutionary new concept in artificial intelligence (AI) that challenges the conventional approach to AI development. Instead of using digital technology, Brain-Morphic AI seeks to more closely approximate the actual human brain by using analog circuits. Advanced trials of this new technology have now been implemented under the direction of Kazuyuki Aihara, one of Japan’s leading scientists in the field of mathematical engineering. Here, Dr. Aihara chats with Yuichi Nakamura, General Manager of NEC’s System Platform Research Laboratories, about the various elements required for AI to reproduce the brain.
Kazuyuki Aihara
PhD in Engineering
Professor, Institute of Industrial Science, University of Tokyo
Specializing in the fundamental mathematical problems related to the brain, chaos theory, complex systems, and cancer, Dr. Aihara focuses his research on mathematical analysis of complex phenomena, brain information systems theory, and developing mathematical models of diseases that can be applied to treatment. He also serves as professor at the Graduate School of Information Science and Technology and the Graduate School of Engineering at the University of Tokyo. Since April 2016, he has been heading the program for solving social issues using Brain-Morphic AI through community-university cooperation, established jointly by the University of Tokyo and NEC.
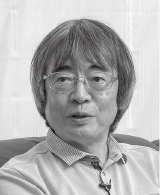
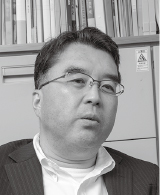
Yuichi Nakamura
PhD in Engineering
General Manager, System Platform Research
Laboratories
NEC Central Research Laboratories
Currently serving as General Manager of the NEC’s System Platform Research Laboratories, which develops basic technology that integrates computing technology and networking technology, Dr. Nakumura is engaged in research into system LSI, signal processing for ultrahigh-speed optical communication, and many-core systems.
Analysis of the brain and nervous system based on mathematical engineering
Nakamura: Dr. Aihara is the leading authority in mathematical engineering - a discipline that seeks to solve various problems by developing mathematical models to represent a wide range of natural phenomena. As for the brain, what kind of research have you conducted so far?
Aihara: My mentors in brain research are brain scientist Dr. Gen Matsumoto and applied mathematician Dr. Shun’ichi Amari. While I was working with Dr. Matsumoto in experiments of squid giant axons, he taught me about the complexity and dynamics of nervous systems. As for Dr. Amari, because I was the associate professor of the Amari Laboratory, I had a chance to learn from him just how much fun and exciting theoretical research can be. In that sense, the basis of my research was built on my studies of the brain and nervous systems from both the experimental and theoretical aspects.
Nakamura: Are you saying that the brain and nervous systems are based on mathematical engineering?
Aihara: I’m particularly interested in the dynamics of organisms. For a mathematical way to describe dynamics, dynamical systems theory is the most powerful of all. So the basis of my research is mathematical modeling using dynamical systems theory.
Nakamura: I think there are multiple patterns for the neural models of the brain. Isn’t it difficult to cover all of them using a mathematical method?
Aihara: The human brain is composed of an enormous number of neurons — about 100 billion — and each neuron has its own characteristics. So it is impossible to reproduce all of the characteristics, and that’s precisely what makes mathematical engineering important.
There has been significant progress recently in research into neurons — especially in the higher-level cerebral cortex. It has been discovered that there are two classes of neurons in the cerebral cortex - class 1 and class 2. As a stimulus to the neurons is gradually intensified, they generate electrical pulses. This is called “firing”. It is known that there are two types of characteristics in change of firing frequencies. One is a pattern in which the frequency continuously increases from almost zero, and this is called class 1. The other is a pattern in which the firing of some non-zero frequency suddenly starts at a certain threshold, and this is called class 2. When these are looked at from a mathematical point of view, they can be clearly classified using bifurcation theory — the saddle-node bifurcation for class 1 and the Hopf bifurcation for class 2. This is an important point. When creating a hardware model of a neuron for example, all that’s needed to reproduce class 1 and class 2 neurons is to design the model so that these bifurcations will occur. So what we are trying to do is to create a neuron model that generates these two types of bifurcations using the device characteristics of electronic circuits.
Nakamura: Does it mean even if there are multiple brain models, the cerebral cortex that controls thinking and remembering can be reproduced as long as a template neuron model is created?
Aihara: Yes. All you need to do to create both classes is to change the parameters of the same model in our framework.
Nakamura: Are there any examples of applications that use such a mathematical model of the brain?
Aihara: One of the most important brain functions is attention. When a living creature pays attention to something it is looking at, that is a high-level function of its brain. So we tried to determine what mechanism is at work in the brain when the creature pays attention to something. Then we came up with a mathematical model that approximates what happens when the actual brain pays attention. When this is applied in practice, it will be possible to make a robot, for example, turn its attention to different things.
One of our recent studies that has been widely praised is a study on the attention of a bat. A bat emits ultrasonic waves and uses the reflected signals to determine the location of targets. So we studied the flying routes of bats and the directions in which they emit ultrasonic waves when they prey on insects in the dark at night. Our analysis combined both experimental work and mathematical models. We found that the bat paid attention not only to the insect immediately ahead of it, but even to the target next to it. In other words, the bat was flying while searching for the optimum route that would connect these two points while simultaneously keeping an “eye” on the insect immediately ahead and the food next to it. This result could be applicable to the design of an auto driving car, for example. You can make it pay attention not only to the car right in front of it, but also to the cars ahead of them. This is just one example of the many things we can learn from living creatures.
Learning from each other - conducting joint research with NEC
Nakamura: When NEC became aware of your research, NEC proposed a joint study in AI using hardware. How did you feel about our proposal when you first heard it?
Aihara: Frankly, I thought it was a good theme. That’s because although research into AI is now actively underway in Japan, Japan is far behind in the area of neuromorphic hardware compared to Europe and North America. Personally, I was quite worried about this so it was happy to take up the challenge when NEC approached me.
Nakamura: Is there any chance for us, starting so late, to compete with Europe and North America, when they are already so far ahead of us?
Aihara: I don’t think there is any problem. That’s because the hardware research in this field has the origin in Japan. The world’s first electronic neuron model was created by Dr. Jin-ichi Nagumo, who was in the generation of researchers preceding Dr. Amari. I think that was in 1962. He used the negative resistance of the tunnel diode which was the most advanced technology at that time to implement an electrical circuit with the nonlinear characteristics of neurons. This circuit is so important historically that it deserves to be in a museum. But it is stored in my laboratory now. So even though there aren’t many scientists researching neuromorphic hardware today in Japan, this country is the wellspring of such research. So it’s fair to say that we have a lot of potential.
Additionally, even if we’re dealing with hardware, the first thing to do is to create a neuron model. So originality in that point is called into question. Because we are simultaneously conducting mathematical analysis of neurons and research into the theoretical clarification of the property, we believe that we can achieve the world’s top-level research by combining mathematical research with the hardware research.
Nakamura: What do you expect from our researchers at NEC when conducting the research?
Aihara: We are of course confident in mathematical models of neurons. However, we don’t have the rich technical expertise in electronic circuits needed when our models are actually implemented. So we expect to learn the technology pertaining to electronic circuits from NEC while we offer theoretical insights to NEC.
Nakamura: At NEC, we have accumulated knowledge in the characteristics and efficiency of electronic circuits. We hope to make useful products by taking advantage of this know-how when our joint project works to actualize brain-like computers.
Simulating the human brain using analog circuits
Nakamura: What should we do with the results of our joint research?
Aihara: The work we are doing is not just a simplified neural network, which is a fad right now. Our research is longer term. Our goal should be to create AI based on a model that mimics the actual human brain. We can do this by implementing in hardware the essential dynamics of the brain such as what the brain’s neurons do and what the brain as a network does.
Nakamura: What exactly do you have in mind? Can you be more specific?
Aihara: The point is that it’s not a digital simulation. Instead, it will replicate neurons with analog circuits. Existing digital computers cannot handle real numbers with an infinite sequence of digits. For example, even if you try to digitally implement a chaotic phenomenon in which the complexity of real numbers surfaces, all you can do is to follow its shadow. But if you do this with analog electronic circuits that can express real numbers, chaos emerges naturally as dynamical behavior of electronic circuits. We have years of experience in this research, so we are confident that we can perform a massive amount of processing by applying the analog property to neurons.
Nakamura: Talking about the difference between analog and digital, is it like the difference between digital and analog music — where an MP3, for example, eliminates components inaudible to human ears while a phonograph record reproduces detailed sound?
Aihara: Although analog and digital can approximate each other, there is a major difference between a digital computer that performs brain-like processing and a brain that performs analog calculations using the nonlinear dynamics of parallel distributed processing because there is a clear difference between principles that underlie their calculations.
So what we want to do is to make an AI using current technology that acts in a way that more closely resembles the way the real brains of animals and humans work. We call it “Brain-Morphic AI.”
Nakamura: What does “Brain-Morphic” mean?
Aihara: It means reproduction of the dynamical behavior of real neurons and the brain using spatio-temporal dynamics of parallel distributed processing based on mathematical models of the neurons and brain.
Nakamura: NEC is also working on the development of computers that don’t use von Neumann architecture. Is Brain-Morphic AI something beyond that?
Aihara: I think we have to approach this from many different directions. In the first place, the origin of current computers is the Turing machine. Turing himself was trying to put the brain’s information processing into a model. That turned out to be successful as technology, eventually leading to the development of today’s computers. But that’s only part of the information processing the brain performs. In other words, although the brain is capable of logical thinking, in reality it often performs illogical thinking. Just think about how you think when you get drunk (laughs). So the brain can do things that are not logical. There are intuitive things that cannot be handled by today’s digital computers. However, the most interesting part of this research for us is to derive the calculation principles that underlie the intuitive aspects of the brain and then to use those principles as the basis for the creation of a brain-like computer.
Nakamura: The models created through this research will be efficiently implemented in cooperation with NEC. Is that correct?
Aihara: Yes, that’s exactly right. I hope that, by implementing new calculation principles, we can expand this technology into new fields of application. For example, robots with improved attention capability will be fabricated and more advanced autonomous driving will be made possible.
Nakamura: Even today, it may be possible to do these things if we use dozens of supercomputers. But there are limits on power consumption and processing time if we want to implement them in robots and cars.
Aihara: To implement AI in various things, it is critical that the AI processor is compact and lightweight, with low power consumption. We are living in a fast-changing world, so we won’t be able to respond in time if we have supercomputers in distant locations and transfer the processed data from there.
Nakamura: Today humans can do these things using our intuition, experience, and instinct. I have a feeling that a world where AI can do these things will be a very, very different world.
Finding the right answers quickly
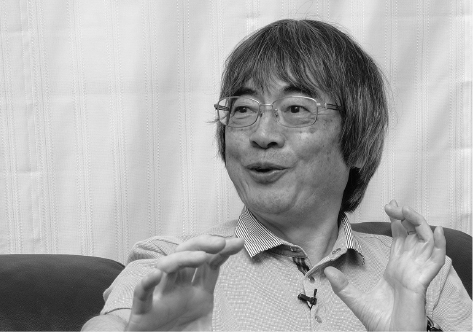
Nakamura: When we are dealing with social issues, what we may require are optimized, efficient solutions that can be achieved quickly - like what’s called approximate computing - rather than absolutely precise answers down to the last decimal point, as is done when calculating the trajectory of a bullet shell, for example. In this regard, is the neuron model of the brain effective?
Aihara: Well, our brain always keeps finding “so-so” answers in a limited amount of time while interacting with changes in the environment and situations. By reproducing this using hardware, we should be able to make a computer flexible enough to cope with dynamic changes in real time. That is one of our most important goals.
Nakamura: When we write a program, we can’t take the effects of any disturbance or interference into consideration. But a new AI like the one we are trying to make would be able to also take the effects of any disturbance into consideration and adjust its program accordingly.
Aihara: That’s right. First of all, a program is something that’s written based on past experience using existing information and data. A problem, on the other hand, is something that needs to be solved - organically, so to speak - and as such it is something that belongs to the future. For this very reason, it’s important that we change the mechanism of information processing so that it can always cope with future changes and create hardware that can cope with those changes.
I like martial arts. In the martial arts, you face your opponent without knowing how the opponent will attack. So if we were to make a martial arts robot using the current technology, despite its powerfulness it would not be able to react to its opponent’s dynamic, unpredictable movements. To create a genuine martial arts robot, it’s necessary to create a completely new mechanism for information processing based on the assumption that it is impossible to predict what will happen.
Nakamura: That’s exactly where the social issues NEC is trying to solve reside. We hope that together we can make dream systems able to handle any problem by combining hardware and mathematical models. Can you tell us what you expect from NEC in this regard?
Aihara: As I pointed out earlier, NEC has a lot of hardware expertise. So I would want to start by taking advantage of that aspect of the company. As for possible applications, we don’t know what kinds of needs are out there. NEC has a lot of experience in dealing with real-world applications and assessing existing and potential social and commercial needs.
Nakamura: The global population is expected to continue growing, and most of those people will live in cities. Of course, new technology will continue to be introduced, but energy and food could become scarce. The Japanese population, on the other hand, is shrinking, and its work force is steadily declining. To help solve these issues, NEC is committed to making contributions in the fields of information and communications. So I hope we can make good use of your research in this regard. Thank you very much.
- This article is edited based on an interview conducted in July 2016.