Global Site
Displaying present location in the site.
Combining AI with simulation technology facilitates decision-making even under conditions where data is limited
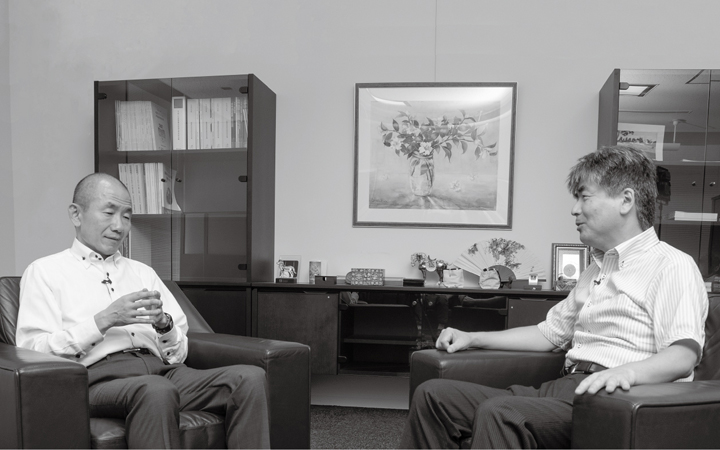
NEC and the National Institute of Advanced Industrial Science and Technology (AIST) have teamed up to establish the NEC-AIST AI Cooperative Research Laboratory in Artificial Intelligence, which conducts joint research and development relating to AI. Here, Akio Yamada, General Manager of NEC’s Data Science Research Laboratories talks to Takashi Washio, who heads the cooperative research laboratory about the applications of AI in social systems.
Takashi Washio
PhD in Engineering
General Manager, NEC-AIST AI Cooperative Research Laboratory in Artificial Intelligence, Artificial Intelligence Research Center, National Institute of Advanced Industrial Science and Technology
Professor, The Institute of Scientific and Industrial Research, Osaka University
In addition to fundamental research into the principles of data mining and machine learning as well as algorithms, Professor Washio is also engaged in development of data analysis technology and applied studies in industrial and social areas. He has headed the NEC-AIST AI Cooperative Research Laboratory in Artificial Intelligence since July 2016.
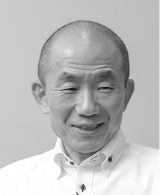
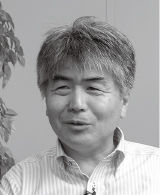
Akio Yamada
PhD in Engineering
General Manager, Data Science Research Laboratories,
NEC Central Research Laboratories
In addition to undertaking research in image information compression encoding, as well as in the areas of image analysis and recognition. As head of NEC’s Data Science Research Laboratories, Mr. Yamada currently supervises research and development in data collection technology and AI-related technology.
Using data mining to discover the relationships between phenomena
Yamada: How long have you been involved in AI research?
Washio: I started working on AI in the early 1990s. At that time the research was focusing on deduction by feeding limited data into computers. By 1995, which was the first year of Japan’s Internet age, it was possible to gather a large amount of data. So I started research in machine learning and data mining which was a promising field at the time. I thought I could make a contribution to the field of graph mining by finding a way to discover hidden relationships between graph structures. In 2000, our group announced the results of our research and introduced the term “graph mining” to the world.
Yamada: The early 1990s was when the fad for fifth-generation computers and expert systems centering around AI had started to subside. Considering this, why did you think it was a good idea to start studying graph mining?
Washio: I have a background in physics originally. When you try to turn a phenomenon that exists in the real world into a model, it is important that you give computers the relationship between each item and look for patterns. Maybe that naturally made me orient towards the concept of the graph structure.
Yamada: What fields is correlation analysis like graph mining applied to today?
Washio: When I started the research, graphs were still small. I was analyzing the relationship between structures and chemical compounds in the pharmaceutical field. Recently, large-scale graph analysis techniques have become more sophisticated, and they are now used in network analysis and structural analysis of macromolecules. They are also used in various areas such as marketing to analyze relations between individuals, for example.
Yamada: What problems stood out to you in the 1990s?
Washio: We had a hard time collecting data and knowledge. The network infrastructure then were not very well developed. So basically all the data had to be entered manually. Also the kind of data that we could collect was so limited that we had to make deductions and find answers based on very little information.
Yamada: What kind of data were you handling? Can you be specific?
Washio: Because machine learning at that time was still at the stage of looking for patterns in small amounts of data, one of the toughest tasks for us was to collect that scarce data. Even in analysis of structures and public opinion, going into the field and conducting questionnaires and surveys was the only way to gather the data.
Yamada: Gathering a large amount of data is a breeze now. So today the process only starts after that. I imagine what was important at that time was designing and planning as well as determining what kind of data would be required.
Washio: In traditional statistical analysis, it’s important to collect clean data and data that’s easy to pre-process. It was a time in which we had control over precision designing in advance and could choose respondents from unbiased population when a survey was going to be conducted. So the quality of the collected data was relatively high, and the key was how to analyze it effectively.
Yamada: You mean it was a time that still had interpretability. Putting the interpretability aside for now, we are in the midst of a trend in which we first analyze a large amount of data. Do you think this trend of laborious data analysis will continue?
Washio: The answer to that is both yes and no. For applications like autonomous driving where a machine has to understand the situation and respond in real time, maybe what’s we need is the ability to make an accurate judgment, not interpretability. However, in many applications, people and AI have to work together to execute a task. In that case, interpretability as well as accuracy becomes important, since the people and the machine have to mutually understand for their collaborations. I have a feeling that these types of applications will become more and more common.
Yamada: I think you brought up an interesting point. To sum up, are you saying that effective communication between humans and machines is required in order for humans to fully use machines and let them take active roles?
Washio: In some situations humans will need to adapt to communication with machines. When machines try to communicate with humans, they will have to present their solutions in a way that people can understand, rather than simply spitting out an incomprehensible list of numbers.
Yamada: Are there any other points you haven’t mentioned?
Washio: For people to interpret the solutions generated by AI will require a new kind of literacy. Ultimately this will be a problem of education at the basic level. In engineering it means mastering the methods of machine learning and data mining and increasing the literacy needed to make full use of them.
Applying simulation to social science to solve social issues
Yamada: You said earlier that in the time when data was limited you solved problems by using clean data. But there are many areas in the world where data is intrinsically scarce. When we try to expand the capabilities of white collar workers including researchers, it seems to me that the amount of usable data available is surprisingly limited. What do you think?
Washio: You are absolutely right. When there is a difference between the amounts of data, you have to divide it into three cases - a case with a massive amount of data, a case with some amount of data, and a case with very little data. When we have a massive amount of data, we can apply it to learning such as deep learning. This would make it possible to utilize machine learning and data mining technology to obtain high discrimination capability.
On the other hand, if the data is focused on a specific area, the amount of data that can be collected will be limited. However, as long as there is a sufficient amount of data - on the level of a few hundred to a few thousand items - current machine learning systems will be able to work with it. While deep learning cannot achieve high precision unless there is a vast amount of data, there are types of deep learning that have been researched in the past that feature quick start-up time and high precision. I think that the application of current machine learning technology such as this will play a key role from now on.
Now, as for the cases where there is virtually no data, there are many research fields in which it is not possible to extract sufficient data experimentally - such as large-scale natural disasters that happened a long time ago, accidents and events that happen very infrequently, development of rare material, and research into physics phenomena represented by the discovery of the Higgs boson particle. Analyzing this kind of data is not possible with current machine learning and data mining technology, so you have to supplement them with other technologies.
I’d say one of the possibilities is simulation technology. You have to have knowledge of the subject and modeling technology to create and use a simulation. So what you do is make a model that’s suitable for a small amount of data and analyze the subject while running the simulation. Or maybe you will need to apply machine learning and data mining to the result of the simulation to understand the phenomenon that’s taking place in that simulation.
Yamada: When we think about simulation, the first thing that comes to mind is a physical wind tunnel test or a weather experiment. What kinds of phenomena do you think you can model at this time?
Washio: We’re now expanding into modelling social science and economics. At our laboratory, we put human movement into models and apply the technology of machine learning and data mining. And then we analyze what mechanism is at work when crowding occurs in a certain space and what measures we should take to alleviate it.
Yamada: That’s very interesting. If we can perform simulation even in psychological areas that have had no room for AI until now - such as human movement, how do you think our society will change?
Washio: Something that is being studied globally right now is evacuation simulations using human movement. There is also research that analyzes human flow and movement in shopping malls. In city planning for example, this study has advanced to the level where the movement of people in rush hour can be reproduced qualitatively. Once we can to analyze the movement of people, we can apply the same techniques to the simulation of cars, trucks, and transit systems. So this analysis will be able to be applied to a fairly wide range of phenomena such as distribution and transportation.
Yamada: When I think about this in relation to NEC, the keywords that pop into my mind are “safety,” “investigation/surveillance,” and the like. Do you think it’s possible to contribute to security guarding, terrorism countermeasures, and disaster prevention, for example?
Washio: Yes, it’s possible. For instance, it’s possible to detect threats in advance by simulating possible scenarios offline. New technology like pedestrian flow sensing has been developed. This can be used for online monitoring in combination with other sensor technology. Or it can be used to manage a specific zone while predicting risk. I think this area has enormous potential.
Yamada: Going a little further, will it be possible to manipulate people’s thoughts and emotions?
Washio: Something that is now becoming popular in marketing is to infer people’s thoughts by running a simulation to see what items people are interested in based on their mental characteristics and personality types and then comparing the simulation results and actual sales results. I think there is a lot of opportunity here to take advantage of machine learning and data mining.
Yamada: Right now, reward cards and POS systems are used to collect data that can be used to analyze customer preferences. Maybe there will be a time in the future when merely observing the targets will enable us to understand what they want and to offer the appropriate services at the appropriate time - with no need to build such large-scale systems.
Washio: If we continue with the current personalization principles, there may come a time when we can actually sift through people’s thoughts and personalities and use that information for marketing.
Solving social issues through joint research aimed at social implementation and industrial application
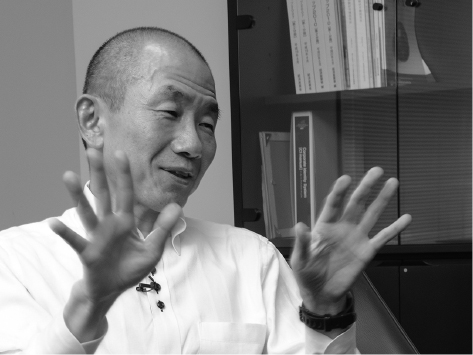
Yamada: The NEC-AIST AI Cooperative Research Laboratory in Artificial Intelligence was established in the AIST’s Artificial Intelligence Research Center in June 2016. Please tell us why you chose NEC as partner.
Washio: Because I had the impression that NEC was approaching AI in a down-to-earth manner. So when I heard about the proposal from the AIST, I thought I could work with NEC. So I accepted the proposal. I’m not trying to be flattering or anything.
Yamada: Thank you very much. You just said down-to-earth. Does that mean you see us a company that conducts research with a practical orientation like solving social issues?
Washio: That’s right. NEC has a good balance between basic research and applied research. There is no discrepancy between them. In the area of basic research, many researchers from NEC have presented their results at society meetings or published them in academic journals, and those results are applied to actual businesses. I think it’s wonderful that NEC has maintained such a research structure for so long.
Yamada: The Cooperative Research Laboratory was launched under your guidance. What do you think we can accomplish in the next three years?
Washio: At the Cooperative Research Laboratory, we hope to achieve social implementation through methodology, while simultaneously conducting basic and applied research. For example, we are planning to research city planning and city development while simulating human movement, show the results to communities, and create a methodology for actual usage.
Yamada: Once all this has been achieved, how do you think it will change our society and industry?
Washio: I think that simulation-based data analysis, machine learning, and data mining will also affect basic science. The third paradigm of science - computational science - is helping science move forward by using models and simulations to help us better understand the underlying mechanisms of various phenomena, while the fourth paradigm - data science - where scientific breakthroughs will be powered by manipulating and analyzing massive datasets. Now everyone is talking about going beyond the current scientific methodology by using AI to integrate simulation and data analysis - that is, the third and fourth paradigms.
Once this new methodology is put into practical use, we will be able to do amazing things, things we can’t even imagine. For instance, in the area of material development, we could develop a completely new material by integrating simulation results and experiment data. It will also have tremendous potential in vast areas of engineering. For example, new principles could be discovered in the information and communications fields that would lead to optimization of these areas.
Yamada: You mentioned that the way science works is going to change. In some sense this will also impact industry trends, as well. For example, if we can reduce the number of trials and errors, the time required for development of new drugs could be shortened to 1/100 or even 1/1000. This will cause prices to go down and reduce the risk of investment, which will have huge impact on society.
The aspect of human psychology you mentioned may be applicable to counter-terrorism measures. To make a world where everybody can feel safe, maybe we need to control potential risks by modeling human psychology - to enter people’s thoughts, so to speak.
Going forward, as you continue your role as leader of the Cooperative Research Laboratory, what do you expect from NEC as a partner?
Washio: I’d like to ask about the actual social issues NEC has already dealt with. I think NEC is in a better position than us to have hands-on experience when it comes to information like this.
Yamada: At NEC we call ourselves “value co-creation laboratories” and a “value provider.” So are you saying that we should bring up specific social issues and work with to develop methods for social implementation and industrial application that will help solve those issues?
Washio: That’s right. That is the primary raison d’être of the Cooperative Research Laboratory. So I look forward to collaborating with NEC in many areas.
Yamada: I’ve got it. We’ll do our best to achieve our shared goal. Thank you very much for talking with me today.
- This article is edited based on an interview conducted in August 2016.