Global Site
Breadcrumb navigation
Completing complex video analysis in real-time:
Application-aware IT & network control technology
Featured Technologies March 2, 2023
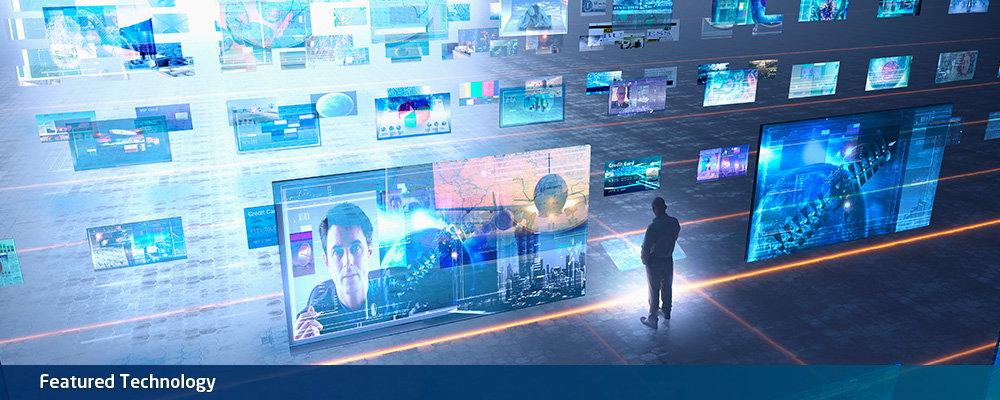
In recent years, the logistics, manufacturing, and construction industries are experiencing labor shortages and issues in ensuring safety. To address such issues, there are increasing needs for installing numerous cameras on site for real-time remote monitoring of work progress and unsafe activities by using behavior recognition and other AI video analytics. However, it has been supremely difficult to analyze and process in real-time video images of many people and heavy machinery moving around, captured by numerous connected cameras. The limitations of edge device processing capacity and wireless communication performance prevented efficient, high-accuracy processing. NEC solved this issue by developing the application-aware IT & network control technology. We spoke with the researchers about the details of this technology.
Predicting important regions for preferential processing between edge devices and the server
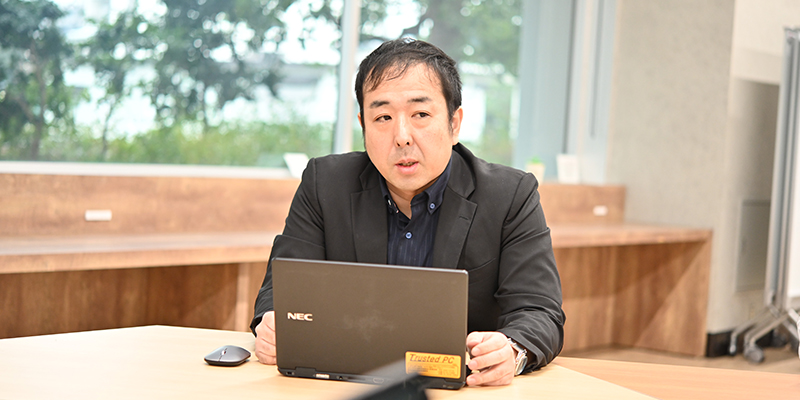
Director
Takanori Iwai
― What kind of technology is the application-aware IT & network control technology?
Iwai: This technology improves real-time video processing and its reliability. Our team has previously developed the learning-based media transmission control technology and the learning-based communication quality prediction technology, which helped the efficient transmission of camera-captured video images to cloud servers or other servers, as well as the server-side analyses with low latency. The new technology further enhances this capability by achieving a balanced distribution of video data transmission and analytical processing between the edge devices installed on site and the server. This enables stable real-time processing of complex AI video analytics even for large-volume video data captured by numerous cameras.
Application-aware ICT control technology
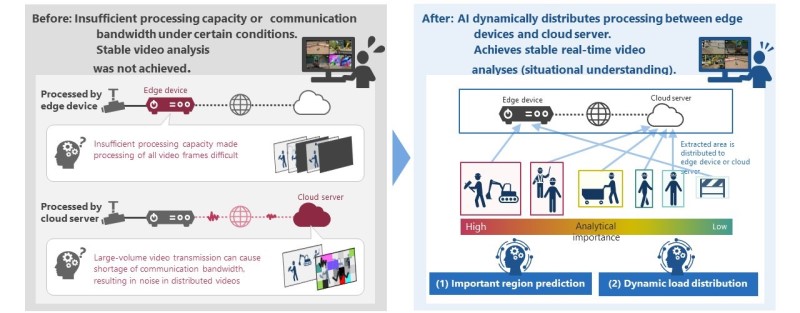

The primary use case that we had in mind in our previous research was the remote monitor and control of autonomous driving vehicles. Since our purpose was to understand the surrounding of the vehicle, we used object detection and other relatively light AI video analytics for video analysis. On the other hand, in this project, we started with a new approach of visualizing the entire logistics, manufacturing, or construction site. This requires a large number of cameras to capture everything on site without any blind spots. Also needed is a sophisticated AI video analytics system that recognizes human behavior and identifies potentially hazardous situations, such as instances where heavy machinery approaches close to humans, among the multitude of people and things in motion. Comprehensively considering all the necessary functions, the current system just cannot handle the processing, both in terms of communication for video transmission and computing for video analyses.
Therefore, a joint project was launched to work on this technology development, combining forces of our team, which specializes in video streaming and communication, the Digital Technology Development Laboratories team,*1 which excels at trimming down computing processing, and the Laboratories America team,*2 which is expert at improving the efficiency of video analysis. It was an approach that only NEC could have achieved, hosting a diverse range of technologies in-house, including communication technologies, computing, and video analysis.
Nihei: To respond to the need to remotely supervise multiple construction sites or a large-scale construction site, it is estimated that at least 30 cameras, maybe even 100 at most, will be connected at the same time. When we thought about doing complex analysis under such challenging conditions, it was already shown in the figures that the required performance was beyond what can be accomplished by merely combining conventional technologies. Therefore, we considered an architecture that addresses this issue and took two major research approaches.
One was to find important regions for preferential processing instead of processing entire videos. This is an advanced version of the learning-based media transmission control technology, and this time we wanted to predict important regions using a more universal AI model than that dealing with only driving videos.
The other approach is a technology that dynamically distributes processing between edge devices and the server based on the importance of each region in the videos. While the processing power of on-site edge devices and the communication capacity of the network have their own limitations, the required performance cannot be achieved without taxing both to the maximum extent. Therefore, this approach was taken based on our conclusion that there is a need to determine whether it is more efficient to process with the edge device or the server for each important region in the video and to distribute this processing in real-time. These two research topics materialized in the form of the important region prediction technology and the dynamic load distribution technology, which are at the core of this technical project.
Advancing techniques based on NEC’s technological developments and know-how
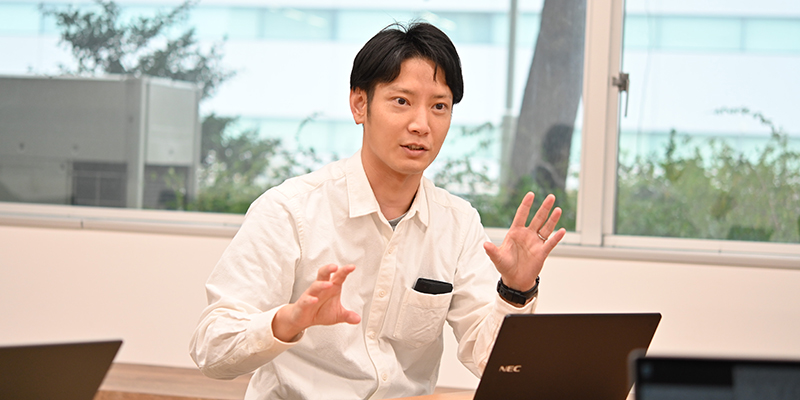
Senior Researcher
Hayato Itsumi
― Please explain the two technologies that compose the technology in this project; the important region prediction technology and the dynamic load distribution technology.
Itsumi: The important region prediction technology can predict in real-time the video regions that are important to ensure that the video analysis model produces correct analyses. For example, a forklift coming towards a person is a hazardous situation, so it can be determined as an important region that needs preferential processing. On the other hand, regions that show no person or machinery have less importance. This technology aims to provide more efficiency in processing by setting priority levels to regions in the video.
Nihei: Recently, an approach has been developed where one video frame is processed by the edge device while the next one is processed by the server, alternating processing media by frame. However, this approach cannot respond to disturbances such as fluctuations in network capacity and computing load. We can also foresee that it cannot support scaled-up systems. In contrast, the important region prediction technology not only achieves temporal separation in distributing processing by frame, but also takes the two-dimensional approach of performing distributed processing by region, cut out and sorted from each frame.
Itsumi: Another notable feature is the capability to universally use any video analysis model. Previously, humans needed to set and teach what part of the video image is important. While setting the necessary picture quality can be automated, important regions needed to be meticulously and individually set manually for teaching every time the application or camera angle is changed, which made scaling arduous to say the least.
However, the new technology successfully automates the learning of important regions. We create images of various regions with lower picture quality through simulation and repeat reinforcement learning, done by comparing with correct recognition results, to improve accuracy. Enhanced video analysis is made possible by a two-step process of analyzing contextual information―”what” is pictured in the video―and performing locational analysis―”where” things show up in the video.
As this technology does not touch the recognition AI model itself, whether the AI is a black box or not, it can universally use any model.
Key area prediction
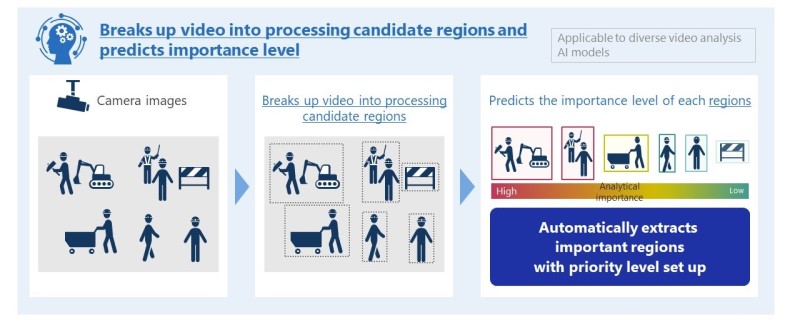

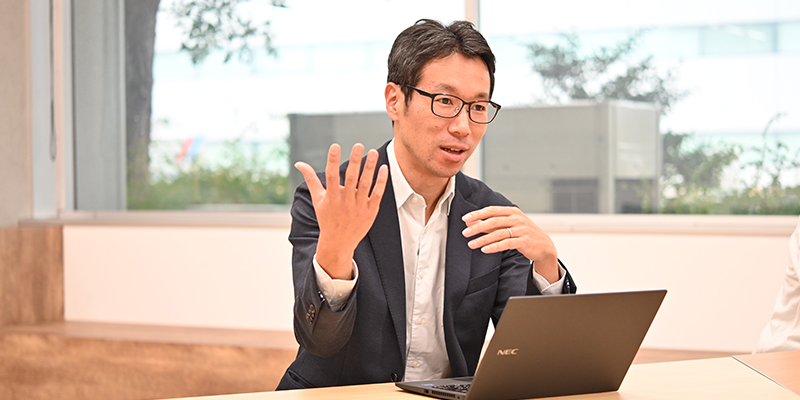
Principal Researcher
Masaharu Morimoto
― What kind of technology is the dynamic load distribution technology?
Morimoto: It is a technology that instantly decides whether the important regions predicted using the important region prediction technology should be processed by the edge device or should be transmitted to the cloud server via wireless communication. In addition to the load fluctuation due to the increase and decrease of people and things in the video, it considers fluctuations in communication capacity of wireless networks in real-time to decide whether the video processing should be sent to the cloud or not.
There are two technical key points in the new technology. One is that it pursues optimization so as to speed up processing wherever possible. When it comes to predicting bandwidth for wireless communications, the accuracy of the predictions diminishes as we attempt to forecast for time periods that are further into the future. Therefore, we sought to accelerate the decisions about processing distribution while maintaining the shortest possible cycle of predictions. The other key point is the handover of processing between the edge device and the cloud server. For example, if something was processed by an edge device up to a certain point but was switched over to cloud processing mid-way, there is a risk of data getting lost. We made adjustments to ensure smooth handovers. As a result, we succeeded in controlling data loss and maintaining accuracy.
I also feel that the accumulation of technologies that NEC has developed up to this date, which includes the learning-based communication quality prediction technology, played a huge part in developing this technology.
Iwai: I agree. NEC has the “technology that enables prediction of communication capacity in advance,” which we developed, to help with preparing for handovers. Also, as the name “application-aware” implies, the new technology processes data with an understanding of the contents and behavior of the application, which can streamline processing to the maximum possible extent. Such know-how became the foundation of research.
Dynamic load distribution
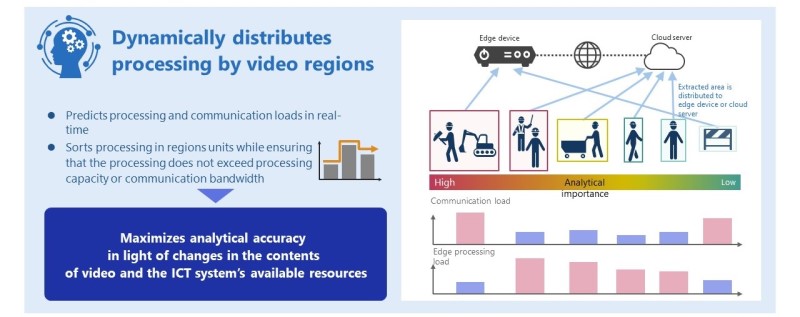

Aiming for more universal application and dissemination by making an application-aware IT & network control platform
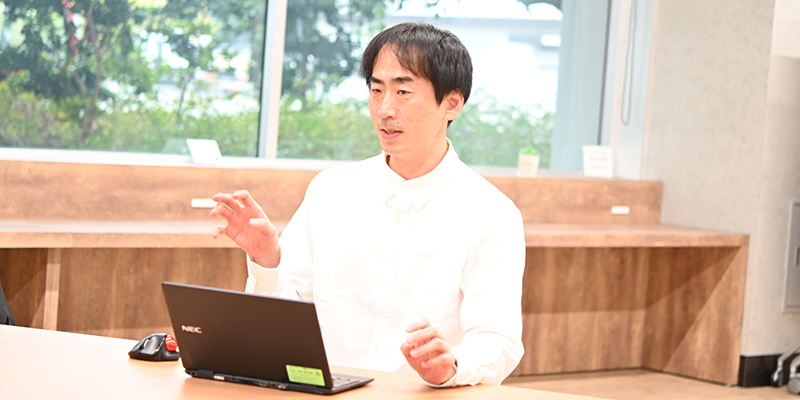
Principal Researcher
Koichi Nihei
― Please tell us about the future prospects for this technology.
Iwai: We are looking at two major goals. One is the expansion of the scope of application by means of collaboration with AI video analytics. The need for grasping the entire situation of the site spans across industries, including construction, manufacturing, and warehousing, and the video recognition AI required depends on the use case. NEC hosts many core technologies that recognize tasks and behavior from video images.*3 By enriching collaborative use of such technologies, we are currently working toward expanding applications to a broader range of fields.
The other challenge is to achieve automation, including control. NEC also has a number of core technologies related to robot control. For example, we are thinking of combining this technology with the automatic control of heavy machinery*4 to offer a one-stop solution that covers everything from analysis to control.
As a solution that NEC should provide, it is our essential goal to achieve full system automation, including control, in addition to presenting analysis results. To that end, it is our role to compile the technologies for commercialization in collaboration with NEC’s business divisions.
Nihei: Yes. I also think that this technology should not be limited to the network domain, but rather be open to a wide range of areas. In fact, our research paper about the important region prediction technology was accepted at the International Conference on Intelligent Robots and Systems (IROS), one of the largest and most impactful robotics research conferences worldwide. Where we have previously mostly submitted papers to communication-related conferences, this is the fruit of our challenge to showcase our technologies in a new field. The recognition this technology received in the field of robotics has great significance to us.
It is also very important to build a platform with wide coverage together with the expansion of the scope of application. While we managed to complete this technology in this project, it must be applicable to various use cases rather than still being something only researchers can handle. I believe that preparing a platform and tools that enable system engineers in the business divisions to deliver solutions to customers is key to spreading this technology, and I would be continuing research based on that awareness.
Itsumi: I completely agree, and aiming for a direction where this technology can be easily used by anyone is a team vision that we share and work on. Nevertheless, as Iwai mentioned earlier, that’s not something we as a team can do alone. A platform can only be made through combining this technology with other diverse technologies that different teams have. Our current goal is to mobilize different technologies to build a platform where even people who aren’t familiar with IT or AI can handle the technologies with no problem.
Morimoto: I also think that how we can make a platform more user-friendly will become increasingly important. Especially, as a feature of our “application-aware” technology, it works with the understanding of application behavior. Similar trends can be observed globally, and this also means that the boundary between applications and platforms becomes blurred. For that reason, going into the contents of application too deep may compromise the versatility of this technology. In light of this, how well we can design this technology into a platform will be the key for future research and development.
Iwai: That is exactly the point: how to easily and automatically achieve processing while understanding the contents of applications. This approach is also manifest in how the important region prediction technology does its job without looking inside the AI and how the dynamic load distribution technology automatically does processing. While we completed the mechanism of efficiently handling processing with the new technology, we will need new techniques to scale up this technology. In that sense, our team consists of reliable members, all with engineering skills. We will continue to accelerate research toward building a platform together with data implementation.
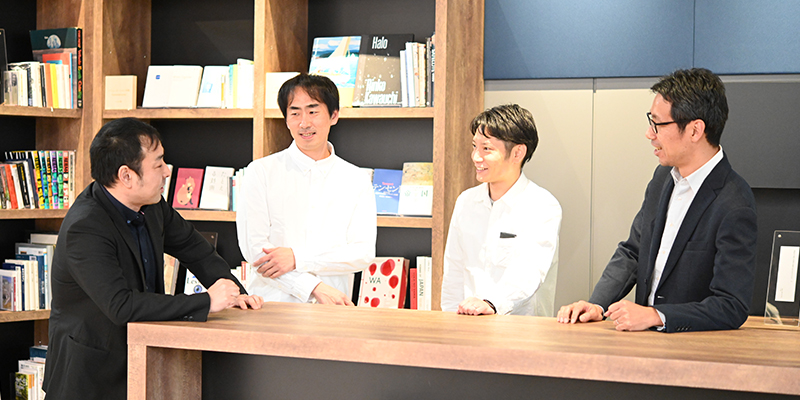

The application-aware IT & network control technology efficiently and dynamically distributes processing between edge devices and a server, thereby achieving real-time video processing and improving its reliability. It consists of two core technologies: the important region prediction technology and the dynamic load distribution technology.
The important region prediction technology predicts regions that are important to the video analysis model to support accurate, real-time video analyses. It utilizes two elements for prediction―the contextual significance and the locational significance within the video image―to achieve accurate judgments on importance level. Also, by using simulation and reinforcement learning, the AI learns the importance level. This technology works without relying on the analytical model, which is a breakthrough feature that makes it universally useful. In addition, this technology adopts neuroevolution (method that uses evolutionary algorithms for neural network learning) for learning importance.
The dynamic load distribution technology instantly distributes processing between edge devices and a server. It sorts processing based on real-time monitoring and decisions based on results output using the important region prediction technology, processing load, and changes in the communication capacity of wireless networks.
- ※The information posted on this page is the information at the time of publication.