Global Site
Breadcrumb navigation
Recognizing the diverse work activities of multiple individuals at work sites
Featured TechnologiesNovember 28, 2022
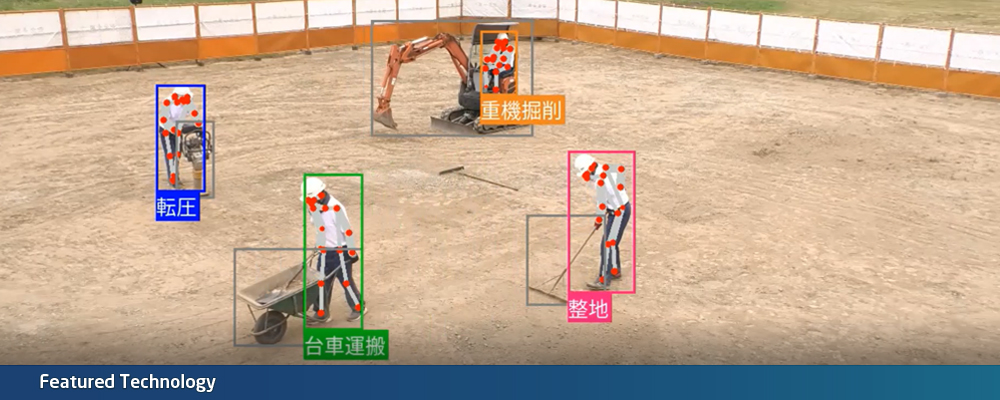
There are increasing needs in industries for recognizing people’s behavior from videos, and many technologies have been developed to cater to such needs. Nevertheless, there has never been a technology that can simultaneously recognize complex behavior of individual people working on different work activities, such as at construction sites and other crowded places where many people come and go. NEC’s newly developed technology can recognize such complex human behavior, something that was previously not possible. We spoke with the researchers about the applications and other details of this technology.
Digitalization of work activities on construction sites contributes to improved productivity
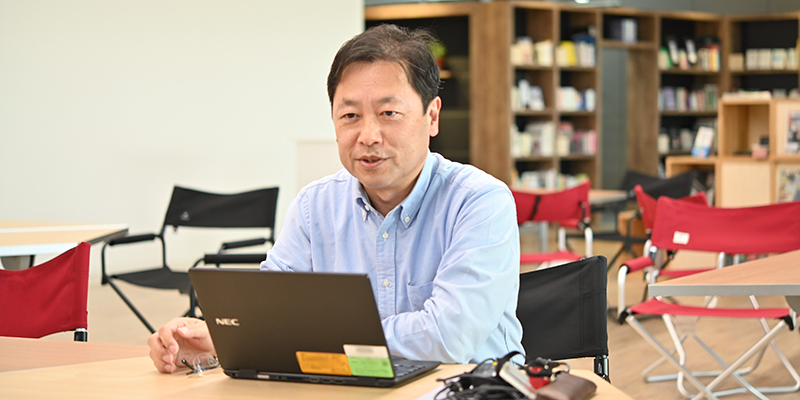
Professional
Katsuhiko Takahashi
― What kind of technology is this technology?
Takahashi: This technology recognizes complex human behavior like work activities with high accuracy by capturing the relationship between the humans and objects in the video. It’s a product of joint development with NEC Laboratories America, a global leader in AI research. Be it construction sites or other places where many people come and go, or settings where the environment changes on a daily basis, this technology can automatically recognize human behavior with high accuracy.
― Why is such a technology necessary?
Takahashi: Construction sites, for example, are seeing an increasing labor shortage due to the shrinking population in Japan in general and the aging construction worker population. Also in Japan, overtime hours will be capped with regulations coming into effect in 2024, which makes working style reformation and improvement in productivity imperative.
In response to this situation, the construction industry has actively embarked on digital transformation, or DX, working on the 3D modeling of buildings. However, the work situation and burdens of on-site workers—the bedrock of production—is difficult to convert to data. Optimization of human resource allocation and improvement of productivity were realms that DX could not set its foot in. This technology enables automatic recognition of what the individual workers are doing at the construction site based on camera images. Therefore, we believe that this can contribute to the fundamental resolution of the issues.
Relationship is analyzed by extracting a variety of information in different representations from images
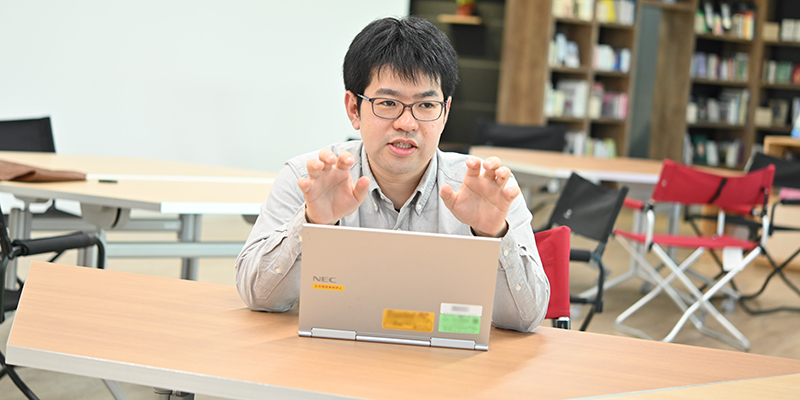
Assistant Manager
Yasunori Babazaki
― What is the breakthrough point of this technology?
Babazaki: Not only the visual features of humans captured in the images, the key point of this technology is that it can link together and analyze multiple sets of information of different nature, such as human poses, class information of nearby objects, and positions of humans and objects in the images. There have been technologies that attempt to recognize human behavior using only visual features or pose information of people in the images. It basically tried to identify a certain behavior from a single feature by using deep learning on a vast amount of image data. However, this method was only applicable to very limited uses. Suppose the AI used only human pose information, you would instinctively see that behavior can be different depending on what the person is holding despite taking the same pose. A single type of features such as human pose is insufficient for high-accuracy recognition of sophisticated behavior such as work activities. Additionally, the conventional methods failed to function when multiple people moved around and occasionally overlapped each other.
In contrast, our new technology extracts multiple varieties of information in the same image, such as what pose people are in, what they are holding and where, and where they are standing, and analyzes the relationships between these pieces of information. This enables precision recognition of more complicated behavior. Even in cases where a part of the body is hidden behind objects or other people, which means that some features are missing, this technology can mutually complement this by using other features such as position information and information on objects close by in order to ensure accuracy. This makes it possible to stably recognize work activities of multiple individuals at sites where many people come and go.
Ando: Another key point of this technology is the selection of information performed to recognize activities when extracting multiple varieties of information from images. To explain further, not all acquired information can be or should be used to achieve accurate recognition of human behavior. Particularly in situations like you would see at construction sites where various heavy machinery and tools are left all over the place or where many people closely gather, some information can turn out to be noise.
For this reason, in order to achieve accurate recognition of complex activities, this technology adaptively weighs different varieties of features, such as human pose, object class information, and positions of human and objects, according to the situation and behavior to be recognized.
Aiming to extend applications to manufacturing, retail, and logistics after ensuring the technology fit for practical use at construction sites
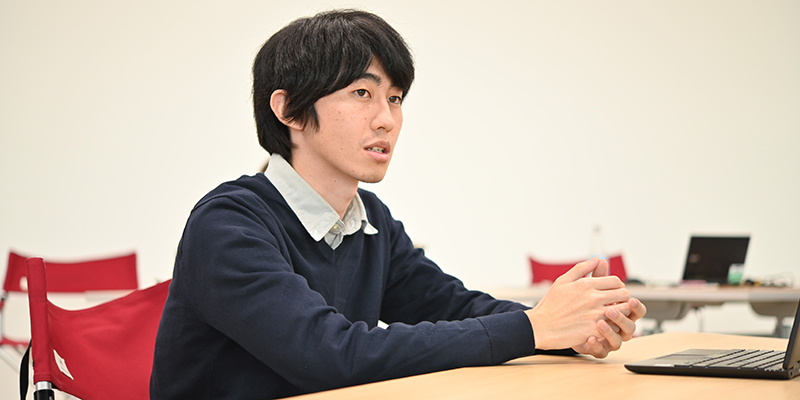
Researcher
Ryuhei Ando
― What level of completion is the technology currently at?
Ando: From March to May 2022, we did a joint testing with Daiwa House Industry Co., Ltd. We set up cameras at a construction site of a stand-alone house to verify the automatic recognition of work activities using this technology.
As a result, we were able to verify that it can accurately recognize multiple work activities, such as "compaction," "root cutting and backfilling," "concrete placement," and "rebar assembly," and estimate the work time of each activity by field workers with less than a 10% error.
This indicates that we can digitally estimate how many workers we need on site and how much time is needed to build the house. We believe our technology can contribute to construction scheduling and optimally allocating workers and other resources.
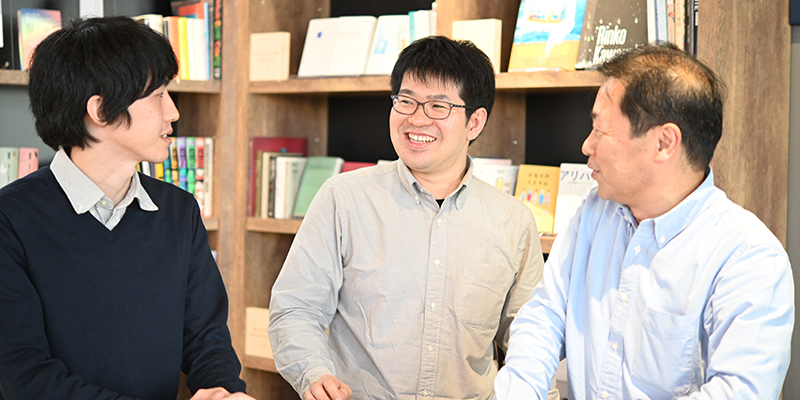
― What are the future goals for this technology?
Babazaki: In this testing, we applied the technology to a construction site of a detached house. In the future, we would like to extend the application of this technology so that human behavior can be recognized throughout the entire process at a diverse range of construction sites, including apartment buildings. We aim for practical application in such a way that it improves productivity.
Also, while we focused on construction sites as our first target, we believe this technology is applicable to other industries as well. For example, we are aiming to contribute to the visualization of work activities and the improvement of productivity in industries such as manufacturing, retail, and logistics.
Ando: Another goal we wish to achieve is to establish a solution that helps introduce this technology to sites. As of present, there is a need to identify and label “correct” target data while collecting training data. We, as data specialists, team up with customers to single out correct data by picking up human behavior that they want to recognize. If we can further assist this task with AI and other technologies, customers should be able to more casually use this technology themselves. We are hoping to develop this technology from such approaches as well.
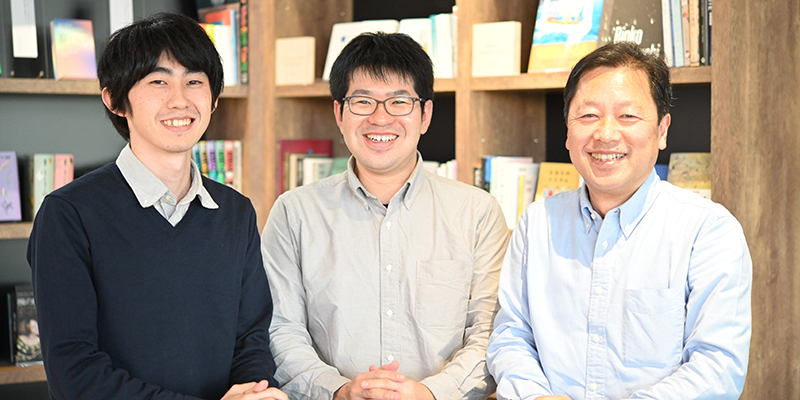
- ※The information posted on this page is the information at the time of publication.

This image recognition technology simultaneously detects a variety of feature information with different representations, such as human pose (skeleton), class information of objects, and position of human and objects, and identifies the human behavior based on the relationship of these pieces of information. While conventional human behavior recognition mainly took the approach of analyzing behavior from single feature, such as human skeletal information or visual information of human and objects, this technology computes by placing multiple features in the same feature space and adaptively weighs key features to enable identification of more complex behavior and simultaneous behavior recognition in places where many people come and go.
Additionally, since machine learning using data from target sites can focus only on parts that concern behavior recognition, the volume of training data to be prepared can be reduced (recognition of human pose and objects can be trained from more accessible different data).