Global Site
Breadcrumb navigation
Communication technology that supports autonomous and unmanned driving
Featured TechnologiesJanuary 8, 2021
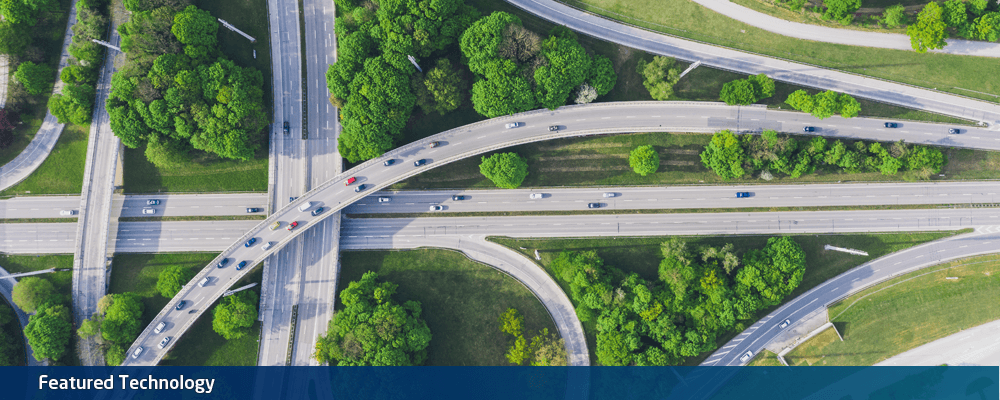
NEC has developed two communication technologies, "learning-based media transmission control technology" and "learning-based communication quality prediction technology," which support autonomous driving for automobiles and various other mobility applications. We spoke with the R&D team about these technologies, which will be installed on buses used in actual operations, in a demonstration experiment starting in January 2021.
Takanori Iwai
Research Manager
System Platform Research Laboratories
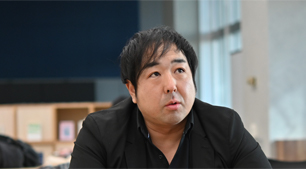
Koichi Nihei
Principal Researcher
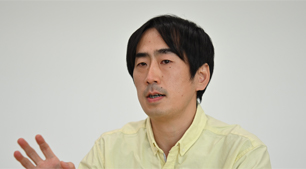
Yusuke Shinohara
Senior Researcher
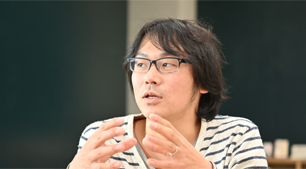
Hayato Itsumi
Senior Researcher
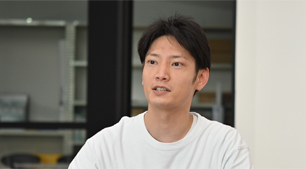
Anan Sawabe
Senior Researcher
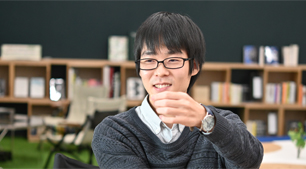
Ensuring driving safety through latency-free communication with monitoring centers and other vehicles
― What kinds of technologies are learning-based media transmission control technology and learning-based communication quality prediction technology?
Iwai: These are technologies that ensure the smooth functioning of AI and IoT, which are expected to accelerate with the adoption of 5G. In the 4G era, the primary focus was on communication that connected "people" with each other, mostly through mobile devices such as mobile phones. In the 5G era, however, the main focus is expected to be on communication that connects "people," "physical things," and "contexts" through the core technologies of AI and IoT. One area that is considered highly important is the field of mobility. For example, in regard to autonomous driving technology at present, the technological evolution of individual vehicles is attracting a great deal of attention, along with the realtime sharing of information between vehicles, street cameras, and remote locations using wireless communication. The goal of our team is to utilize communication technology to achieve safe and efficient autonomous driving.
People tend to assume that communication is simply a matter of establishing a connection. However, in wireless communication, the communication quality, including the communication speed, varies depending on the radio environment or the amount of transmitted data. In the past, it was only necessary for the communication quality and speed to reach a certain level. In recent years, though, greater attention is being paid to communication quality as well as the quality of applications that use communication. This is presently known as "quality of experience" (QoE) or "quality of control" (QoC), and it is becoming a performance indicator. Therefore, in addition to simply understanding the communication quality, it is now also very important to understand the characteristics of the applications and to implement effective and efficient communication control to improve the quality of the applications. This mindset is also at the core of safe driving assistance and remote control operations that use the communication we are currently working on.
This is why our team includes multiple specialists, such as experts in video distribution for applications, in addition to experts in communication. It is only with such a team that these two technologies could be achieved.
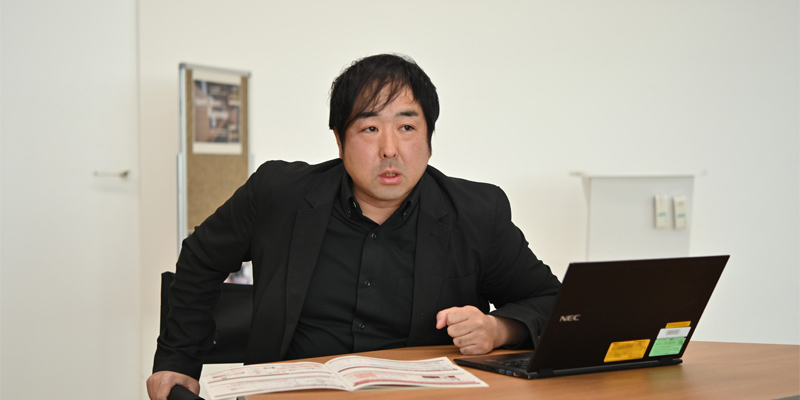
Research Manager
System Platform Research Laboratories
Video transmission that sharpens only the areas of interest that affect driving decisions
― Tell us more about the learning-based media transmission control technology.
Iwai: This is a technology that can automatically detect danger by using video analysis AI with video that is transmitted in real time to a remote location such as a monitoring center. The main usage scenario we envisioned when developing the technology was unmanned driving operations for public transportation such as buses. According to the roadmap for the realization of autonomous driving set by the Japanese government, public transportation is required to incorporate systems that enable remote monitoring and remote control in the event of an emergency. This technology satisfies these needs.
Itsumi: When transmitting video from a moving vehicle, it is usually necessary to communicate as much as 5 Mbps of information. However, the communication speed always fluctuates with such a large amount of data, even with 5G, so there is a considerable amount of noise and latency in the video received by the monitoring center. If noise occurs, there is danger of missing some risk factors such as oncoming vehicles and pedestrians, and if any delays occur, remote control operation of the brakes may not be performed in time.
Therefore, this technology uses AI to automatically assess only the areas of interest in the video, and it scales down the image quality of the other parts of the video as much as possible before transmission. For objects that contribute to the driving decisions, such as other vehicles, pedestrians, and traffic lights, the image quality is kept to a level at which AI can perform video recognition. On the other hand, the image quality of objects such as trees and buildings that are not related to driving decisions is greatly reduced. By doing so, 5 Mbps of video can be reduced to approximately 1/10 the original size, to about 500 kbps.
Shinohara: The area of interest in the video is automatically determined by AI, then it is automatically adjusted to the optimum image quality for transmission. This technology was realized by performing machine learning using data transmission indicators that determine the lowest image quality that can be recognized by video analysis AI at a remote location. We used an open-source recognition engine for future scalability, and the recognition process is completely black box, as is the case with many deep learning frameworks. We carried out fine tuning through a rigorous and repetitive process of trial and error.
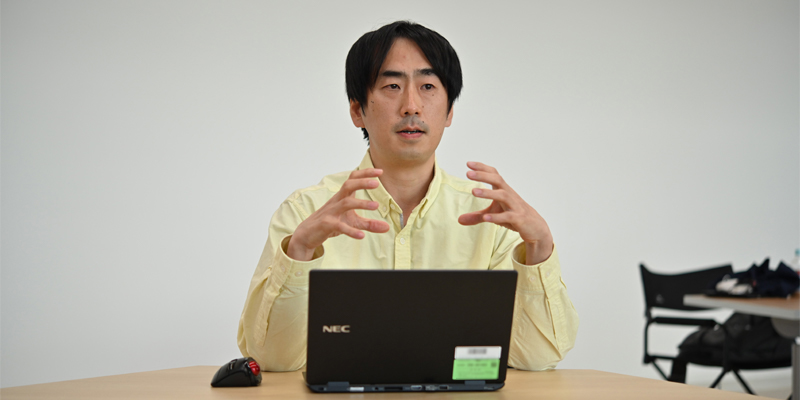
Principal Researcher
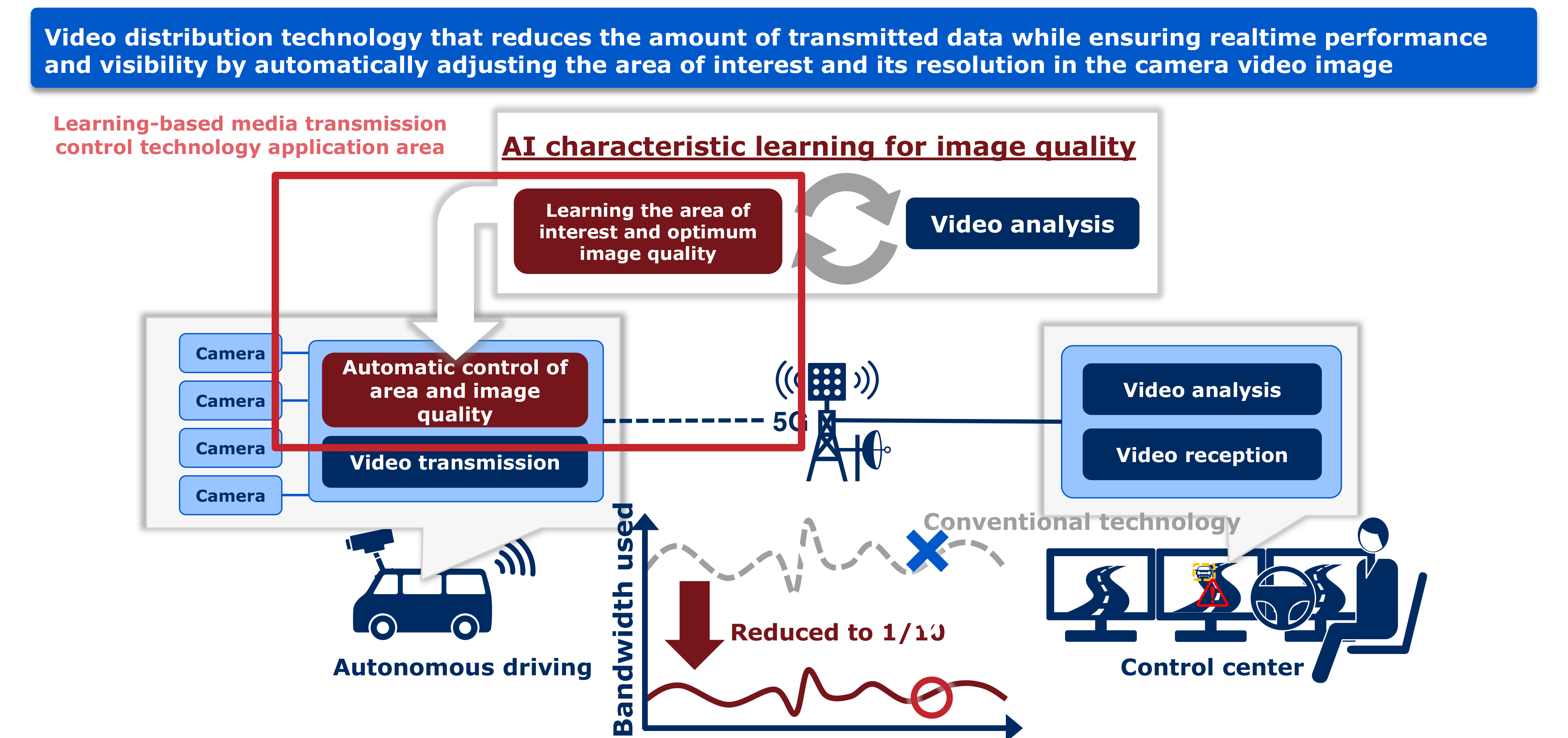
Nihei: After deciding what to concentrate on in the video and what the image quality should be, the next important factor is to compress the video. Since I was already doing research in video distribution and video compression, I assumed the task of sharpening only particular areas in the video for transmission. NEC had previously developed the base video compression technology that we utilized. However, since I do not have the expertise to decide which areas should be transmitted at what resolution, I have been working to develop this technology in a way that complements the work of Shinohara and Itsumi.
Iwai: That's right. As I mentioned earlier, I think that the collaboration between two specialists in communication and video has contributed greatly to the development of this technology.
Achieving realtime video transmission and video analysis
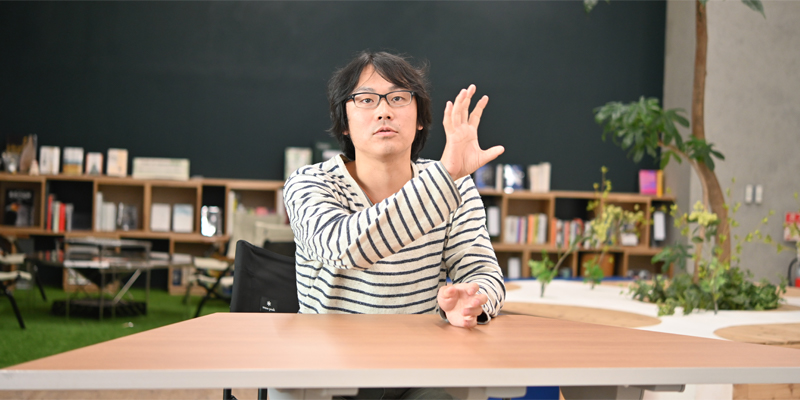
Senior Researcher
― Does this technology mean that video transmission as well as analysis can be processed in real time?
Iwai: Yes, that's correct. That was the most difficult point. If we try to process video from an in-vehicle camera without any additional measures, it takes about 300 milliseconds per frame. For video that is captured and transmitted at a rate of 10 frames per second, the processing needs to be performed in 100 milliseconds or less, so the current speed is not fast enough. Furthermore, this technology is being considered for use in mobility applications such as autonomous driving. For this reason, the need to wirelessly communicate and process video captured by moving objects was a major issue.
Shinohara: It is relatively easy to estimate the optimum image quality if you use a large, complex computational model. However, since the devices that can be installed in vehicles have limited performance, it is not possible to use large computational models that can only be handled by the high-performance devices used in research. Therefore, we have made adjustments that enable even small devices to achieve high accuracy. Specifically, there is not enough time to analyze each and every frame of video, so we are building a framework that can predict the areas of interest and the appropriate image quality based on prior learning. Since the recognition rate varies depending on the direction of the vehicle, small changes are made, such as fine adjustments to the image quality. This reduces the amount of processing to ensure realtime performance.
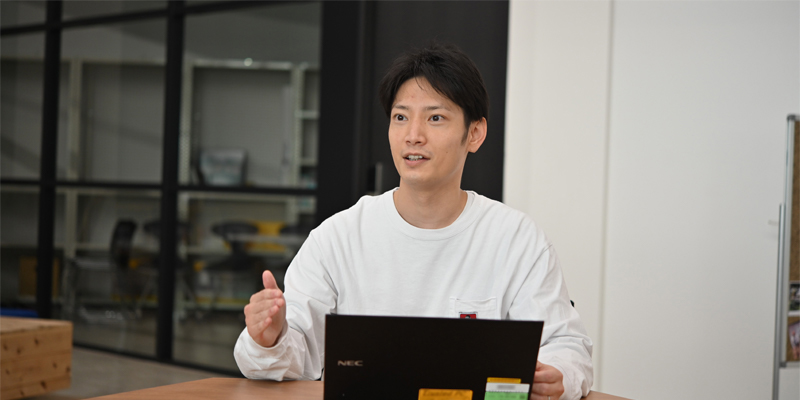
Senior Researcher
Itsumi: That's right. Since in-vehicle devices have low processing performance, we struggled with the gaps between evaluation and actual implementation of the technology. Even though we were concerned with sharpening only the areas of interest in the video, we had to deal with other issues at first, such as problems with tracking.
Iwai: In that respect, the engineering when implementing the technology was very important. No matter how much you adjust the algorithm, you end up with unexpected delays when you try to implement the system. Nihei had a lot of experience with implementing technology, so he was a great help.
Nihei: High quality can be achieved through technology, as well as through repeated implementation and optimization. When I joined NEC, I worked on product development in a business division. I think I have been able to successfully incorporate that know-how into the video compression technology.
Iwai: We are conducting collaborative research on this technology with Gunma University for a national project under the jurisdiction of the Ministry of Internal Affairs and Communications, and a demonstration experiment is scheduled to start in January of this year. The system will be installed on buses operating with the cooperation of the city of Maebashi. We recognize that this demonstration experiment is attracting worldwide attention for verifying the extent to which communication technology can improve the accuracy of autonomous driving. We look forward to thoroughly evaluating the results of this experiment for future use.
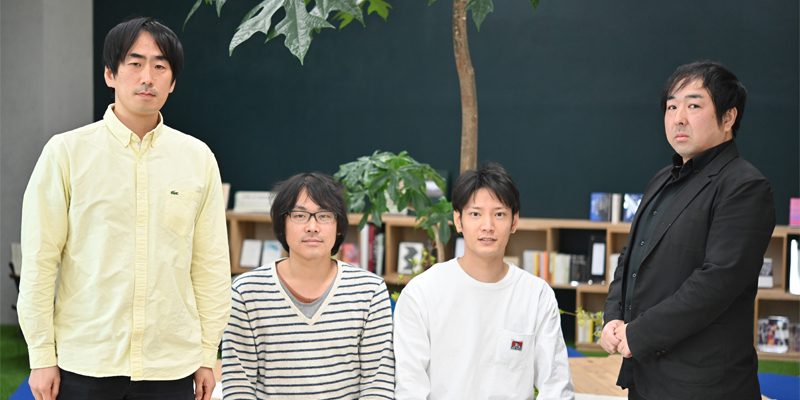
Accurately predicting communication delays to avoid risks in mobility
― Could you tell us more the learning-based communication quality prediction technology?
Iwai: It is technology for predicting communication delays. Although we have been developing technology for predicting communication throughput, we have taken this one step further and optimized it for mobility.
Sawabe: With conventional communication prediction technology such as communication throughput prediction, changes over time are used to predict communication throughput in the future. However, when it was envisioned that the mobility field would be the main usage scenario for this technology, the starting point was to consider that changes in position rather than changes over time would be the main cause of fluctuations in the communication status.
For example, other vehicles on the road enter the area and pass through while sending data to the same server. Since many vehicles travel on the same road, it is possible to learn from past data about the types of patterns that exist in the communication during travel. We created multiple models from the data by dividing the area into multiple sections, and then further dividing those sections with similar communication quality into smaller segments, and we designed the models to achieve a high prediction accuracy. The previously announced "learning-based communications analysis technology" was used to determine which applications were being used based on similarities in the communication status. This time, we have enhanced this technology and are using it to find similarities between areas. We are currently preparing a research paper that describes our work in detail.
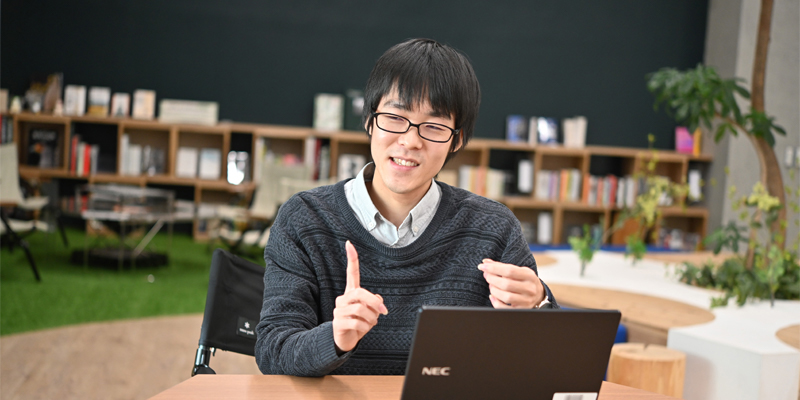
Senior Researcher
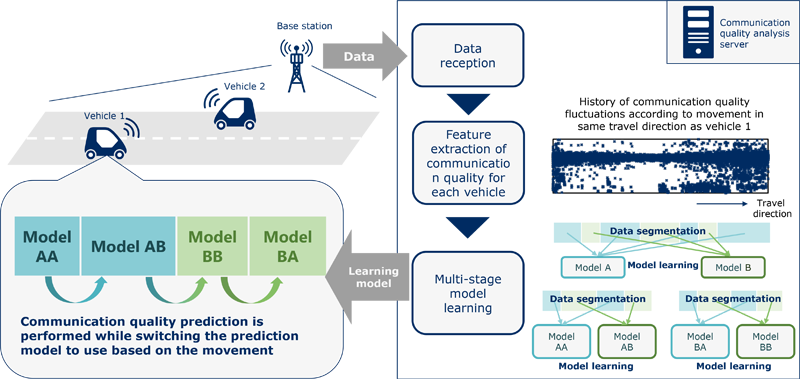
Iwai: Since a vehicle moves at high speed, the radio wave conditions change rapidly as the position changes. It is therefore not possible to achieve a sufficient prediction accuracy with time-series data only. I think that this approach of dividing the space based on the data, creating multiple models, and switching between them depending on the situation is the first of its kind in the world.
At present, it is said that in order to improve automobile safety, additional technologies for operations such as inter-vehicle communication and remote monitoring are required in addition to the operation of in-vehicle sensors. For that reason, there could be a breakthrough in the direction of communicating location information between vehicles. However, real time communication without latency is essential to realizing this idea. For example, consider a situation where location information needs to be transmitted in 100 milliseconds or less. If it is likely to take longer than 100 milliseconds, it is necessary to predict the situation in advance and take some action. Learning-based communication quality prediction technology was initially developed to realize high-accuracy predictions with such applications in mind. In addition to autonomous driving, this technology can also be used in safe driving assistance for ordinary vehicles. Furthermore, we believe that it can be applied and deployed in various scenarios such as those involving transport robots.
Trial operation of this technology will also be included in the demonstration experiment that is scheduled to start in January. We look forward to receiving the actual data and carefully verifying the prediction accuracy. To that end, we are making thorough preparations to ensure the success of the demonstration experiment.
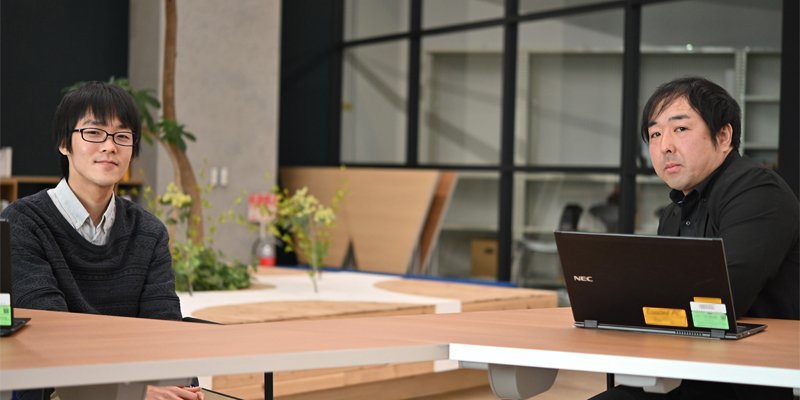
- ※The information posted on this page is the information at the time of publication.