Global Site
Breadcrumb navigation
AI Automatically Identifies Manual Work to Help Improve Productivity
Work Process Recognition Technology
Featured Technologies September 15, 2022
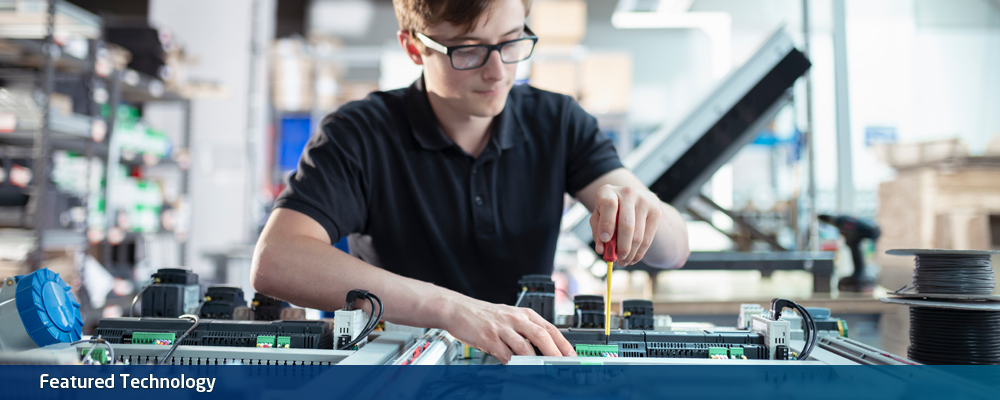
In sites such as manufacturing plants and warehouses, significant amounts of manual work are still responsible for important processes today. However, it is extremely challenging to objectively ascertain the time required for detailed work that is performed by hand, and it has become a major issue for the improvement of productivity. At NEC, we developed a "work process recognition technology" to bring about a digital transformation in such work as well. We spoke in depth with several researchers about this technology which visualizes the time spent on the manual work in each process and has the potential to significantly increase productivity in the field.
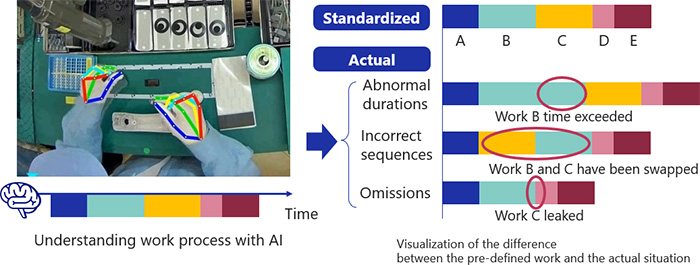
Automatically identifies dozens of processes with high accuracy from video data
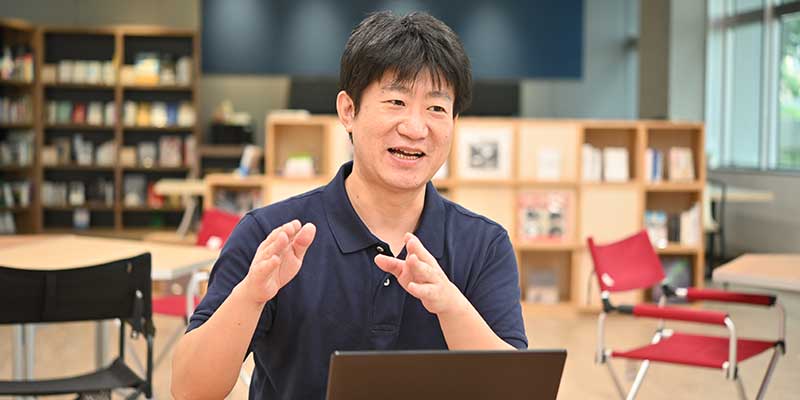
Principal Researcher
Tetsuo Inoshita
― What kind of technology is work process recognition technology?
Inoshita: It is a technology that can automatically identify worker behavior from video. It can identify several dozen types of detailed work in high accuracy with AI that analyzes hand shapes and images of the area around the hands.
Currently, the automation of work is advancing in various locations such as plants and warehouses, but there are still many processes in which manual work is essential, and there are issues with improving productivity. We conceived this technology as something that would target such manual work. It was developed with the goal of efficiently assessing the time required for each individual process to help improve productivity. Until now, the only way to do this was to rely on analog methods in which the person in charge periodically measures the time with a stopwatch, but by using this technology, you become able to automatically, accurately, as well as constantly assess the work time for each process.
Ishihara: Until now, there were approaches which analyzed an entire set of video data with deep learning, but doing so required a massive amount of learning data, and the work which could be analyzed was limited to just a few processes. Meanwhile, there is also an approach which analyzes posture from the joints of the human body, but this was effective when targeting large motions that use the entire body. It is not suited to detailed work such as component assembly.
In contrast, this technology takes a new approach of analyzing the shape of the hands and images of the surrounding area. As a result, it can identify dozens of processes even for detailed manual work with high resolution and high accuracy.
Creating an AI that can identify roughly 20 different processes from several example work videos
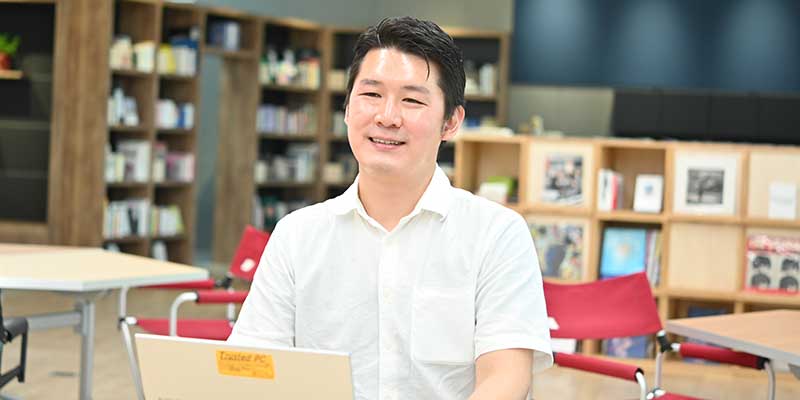
Researcher
Kenta Ishihara
― Specifically, what kinds of technologies are used?
Ishihara: The algorithm which recognizes hand shapes uses general methods, but we devised ways to increase the robustness at actual work sites such as support for special gloves worn at manufacturing sites. We successfully increased the precision and efficiency by fully utilizing the knowledge accumulated in biometric authentication, a field where NEC excels.
Inoshita: We also pursued and carried out development aimed at making the technology easy to introduce and easy to operate. The reason is because conventional technology which used entire sets of video data required an extremely high cost to prepare the teaching data. We film example videos and repeat the process of labelling the "correct" work, with the time from here to here labeled as process A, the time from here to here labeled as process B, and so on. Moreover, you have to perform that work for several hundred videos. This placed a significant burden on the customers. For that very reason, the number of processes which could be analyzed was limited to just a few types.
However, with this technology the analysis focuses on the hands and a small area around them, so if you create teaching data consisting of just a few videos, you can create an AI that can automatically analyze dozens of different processes. It depends on the analysis details, but the learning finishes in about a day, so the introduction is extremely fast.
Ishihara: Moreover, it learns the "co-occurrence relationships" between the hands and the surrounding image information to identify several dozen types of processes with high accuracy. The reason why is because if you are looking only at the hands, it is possible that it might identify a movement as the same process when a different component is grasped. For that very reason, in addition to the shape of the hands, the technology was polished by having it reference image information such as the color and shape of components shown in the surroundings. It learns the "co-occurrence relationships" combining both types of information to become able to identify even detailed work with high accuracy.
A research paper summarizing this technology was accepted by MIRU2022, which is Japan's largest image recognition/understanding technology conference and presented orally on July 26. In addition, the research paper was also accepted just the other day by ICIP2022, which is one of the top international conferences in the world. It is scheduled to be presented in the middle of October.
Identification results for telephone assembly work
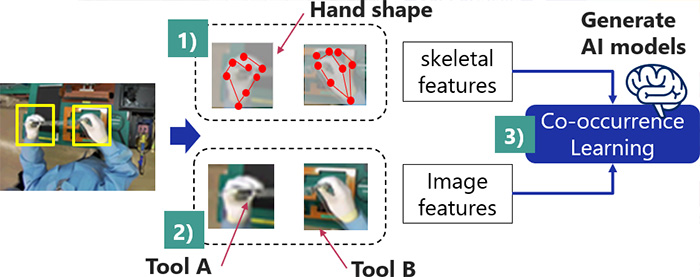
Verifying the technology in consideration of anomaly detection and the simplification of advance preparation
― What level of completion is the technology currently at?
Inoshita: We conducted a demonstration experiment within a manufacturing plant owned by NEC Platforms, Ltd., a member of the NEC Group. We successfully identified the work processes and derived the times, which enabled us to ascertain the differences with the work times prescribed in advance. As a result, we visualized the work bottlenecks and were able to verify the technology's ability to contribute to productivity improvements, which was a major outcome.
We have already received inquiries from several customers, so currently we hope to accelerate further demonstrations. We think that this technology can prove to be useful to a wide range of customers not only in the manufacturing industry but also warehousing work and box lunch packing work at food service plants, so we plan to carry out demonstrations from a broader perspective.
― Does this technology have further potential, and what are the future goals?
Ishihara: As for myself, I would like to embed "anomaly detection." The system would be able to issue an alert when the order of the prescribed processes changes or processes are skipped. If such anomalies can be detected during work, then it would definitely help to significantly improve the productivity. I hope to simultaneously verify this technology as well during the demonstration experiments.
Inoshita: In parallel with that effort, I would like to make the technology easy for customers to introduce and use. Regarding the preparation of the learning data mentioned a moment ago, I would still like to examine if there are ways in which it could be further simplified. For example, if we could completely automate the data preparation, it would likely be a significant advantage for customers. Going forward, I hope to continue researching technologies which promote commercialization from the customer's point of view at all times.
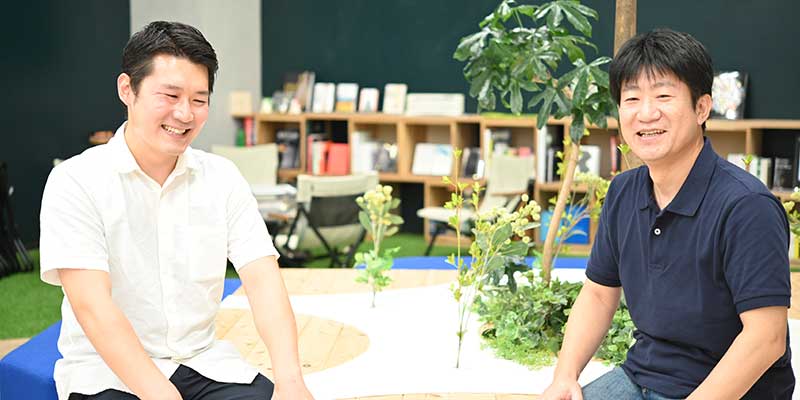
- ※The information posted on this page is the information at the time of publication.

The work process recognition technology combines a technology that recognizes hand shapes with deep learning and image recognition technologies. While hand recognition itself uses a general method, we have significantly increased the robustness through special techniques devised by NEC so that it can support any kind of scenario. Moreover, a unique NEC breakthrough can be seen in its ability to learn mutual co-occurrence relationships by combining not only the hand shapes but also the surrounding image information.
As a result, it became able to recognize more than several dozen different work processes with high accuracy and an overwhelmingly small set of data compared to conventional image analysis approaches based on deep learning.