Global Site
Displaying present location in the site.
“Anokorowa CHOCOLATE” Lets People Savor Delicious Chocolates that Reflect the Mood of Special Moments in History
The result of a unique collaboration between NEC’s AI technology and a renowned bean-to-bar craft chocolate maker, “Anokorowa CHOCOLATE” captures the flavor of some of the most exceptional years in the past half century. Our AI analyzed a massive number of words in newspaper articles and converted results into multiple taste index values, which were then used to create five different chocolates with distinctive flavors that capture the mood of the time. This paper introduces the methods used to convert words into taste index values and discusses the potential for product co-creation between humans and AI.
1. Introduction
In what may be a sign of things to come, a unique series of chocolates has been created through a collaboration between NEC the WISE, a suite of cutting-edge AI technologies, and Dandelion Chocolate Japan, a small-batch bean-to-bar chocolate maker that originated in San Francisco. Dubbed “Anokorowa CHOCOLATE”, these chocolates feature distinctive flavors that capture the mood of some of the most stand-out years in the past half century. Now with “Anokorowa CHOCOLATE”, we have tried to capture the flavors of the past in delicious chocolates whose creation drew on the results of AI analysis of a massive number of words from newspaper articles from specific periods.
This paper explains how “Anokorowa CHOCOLATE” was developed, focusing on how we used AI to analyze and convert words into taste indices of chocolate.
2. The Development Process
“Anokorowa CHOCOLATE” both derived from our efforts to find ways to make AI better comprehend human feelings and senses. Humans often express feelings and emotions using taste as a metaphor, such “bitter” or “sweet.” One of the biggest challenges was how to train AI to learn these human sensations. With the end of the Heisei Era approaching in Japan, people were expressing a renewed interest in history, so we thought it would be fun to make it possible for people to actually taste the mood of historical moments using a familiar snack like chocolate and the power of AI to create a unique experience never before possible.
Here’s how we did it. First, the AI learned the meanings of words contained in newspaper articles from the past sixty years or so. This massive amount of words was then converted into the seven taste indices that determine the flavor of chocolate. Next, by aggregating the words used in newspaper articles every year, the AI created recipes that would express each year’s mood with a different flavor of chocolate. Then we picked five years which were particularly momentous and asked Dandelion Chocolate to make chocolates expressing the moods of those years based on the recipes the AI had concocted (Fig. 1).
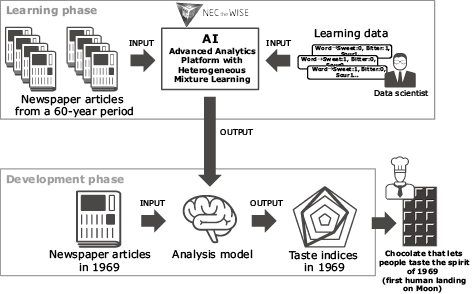
The newspaper article analysis process performed by the AI is described in detail below.
2.1 Segmenting of sentences into words
In order to allow the AI to analyze the data of newspaper articles, we first performed a morphological analysis on sentences in newspapers. In morphological analysis, a sentence is broken down into the most basic meaningful units (i.e., words). The words can then be used to apprehend the content of the sentence and phrases. For example, if the phrase, “a sentence is segmented into minimum units” is run through a morphological analysis, it will be segmented into “a,” “sentence,” “is,” “segmented,” “into,” “minimum,” and “units.” For this project, we used a dictionary called NEologd1), which is also compatible with proper nouns and new coinages, to perform morphological analysis. Adjectives and verbs were converted into base forms and then extracted.
2.2 Vectorization of words
Words extracted from newspaper articles using morphological analysis were then read into the AI for analysis. We used a method called Word2Vec2) to digitize the words (conversion into a vector representation with approximately 200 dimensions). Word2Vec makes it possible to represent words in vectors by using neural networks to analyze massive amounts of textual data. Learning in Word2Vec is based on the distribution hypothesis, which claims that the meaning of a word can be determined by the peripheral words when the word appears in a sentence. For example, in the sentences “I keep my dog inside” and “I keep my cat inside”, the same words appear around “dog” and “cat,” implying that “dog” and “cat” have similar meanings. By applying this hypothesis to an enormous amount of text data, the AI is able to learn how words are used, which makes it possible to use vectors to express words with similar meanings.
Representation of words with vectors enables the AI to calculate the similarities between words and work on word formulae, such as “Paris” – “France” + “Japan” = “Tokyo”. Table 1 shows examples of words with high similarities.
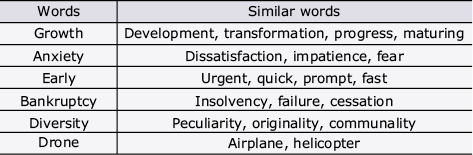
2.3 Creation of learning data
Having received the cooperation from Dandelion Chocolate, we decided upon the seven taste indices — sweet, bitter, sour, nutty, floral, fruity, and spicy — that express the flavors of chocolate. Then we extracted about six hundred words that appear frequently in articles on the front pages of newspapers from the past sixty or so years and allocated one of the seven taste indices to each of them (Table 2). The tastes people associate with words are set. For example, the sweet taste index is allocated to words like “recovery,” “improvement,” and “growth” while the bitter taste index is allocated to “anxiety,” “slump,” and “severity.”
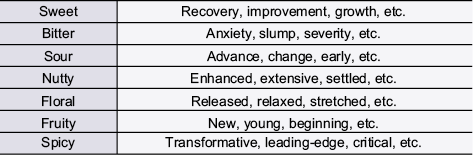
2.4 Building prediction models to the “taste” of a word
We used Heterogeneous Mixture Leaning, which is one of NEC’s AI technologies, to model the relationships between the words and taste indices. Specifically, we built prediction models to judge whether words vectorized with Word2Vec correspond to the seven taste indices (whether it’s sweet or not, whether it’s bitter or not, and so on) by using the numerical values of each dimension of vectors as explanatory variables. The use of these models makes it possible to calculate the tastes of various words (taste index values).
To perform this analysis, we used the NEC Advanced Analytics Platform (AAPF) with Heterogeneous Mixture Learning. The AAPF is an AI utilization platform that integrates various NEC the WISE components and standard open source software. It allows you to perform analysis while linking the NEC-original Heterogeneous Mixture Learning AI technology with Word2Vec as well as morphological analysis and other tools required for text analysis — all on the same platform.
2.5 Predicting how words will taste and creating recipes for the selected years
Using the prediction models we built, we estimated the tastes of all the words contained in the newspaper articles for about sixty years. Since similar words show similar vectors (values of explanatory variables), their taste is assumed to be similar, as well. We drew radar charts of the taste indices by adding up the tastes of the estimated words for each year, adjusting the weight according to the degree of frequency of a word’s appearance. Various types of statistical processing such as normalization were performed on each year and each taste index — in order to emphasize the characteristics of the particular year.
In this way, we analyzed the newspaper articles over a period of about sixty years and created a radar chart (a chocolate recipe) for each year. We selected five years which were particularly momentous and developed chocolates to express the taste of each of those years. The recipes for each of the five years are shown in Photos 1 to 5.
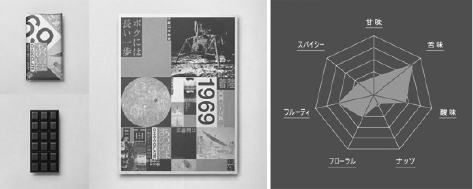
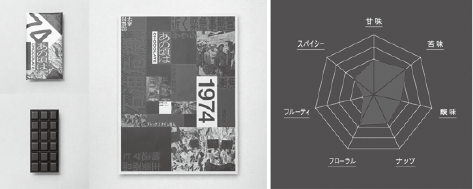
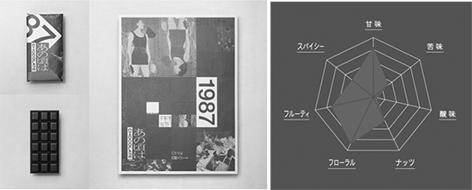
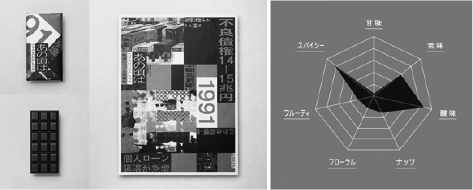
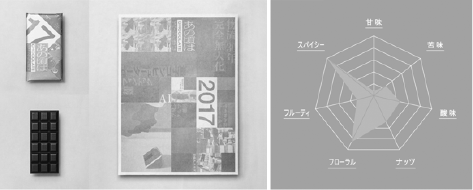
According to the recipes created by the AI, the values for sweet, floral, and fruity tastes were especially high in 1987 when Japan’s bubble economy hit the ceiling because words high in those values such as “growth,” “highest,” and “increase,” were frequently used in newspaper articles. As a result, the chocolate representing 1987 is sweet and mouthwatering. In contrast, the chocolate that expresses 1991 — the year the bubble burst — features sour, spicy , and bitter tones, reflecting the frequency of words such as “bankruptcy,” “recession,” and “collapse.”
3. Product Development through Co-creation between Humans and AI
When developing “Anokorowa CHOCOLATE”, we not only trained the AI to learn human sensitivities — that is, the impressions people get from words — as numerical values of taste indices, we also succeeded in demonstrating the potential for humans and AI to work together and co-create something new — in this case, chocolate whose taste reflected a specific period in history. Typically, when a chocolate is developed, the first thing that must be decided is the type of cacao beans to use. Then the chocolate is made in such a way as to maximize the good points of the cacao beans. This is how the flavor of that chocolate is determined. In this project, however, the recipe expressing the mood of the period came first. Cacao beans were then selected to achieve the flavors required and adjusted as necessary to obtain just the right taste. In other words, the process was completely opposite. Dandelion Chocolate found the project invaluable, gaining a new perspective and understanding of their craft, as well as stimulating their imaginations.
At NEC, we believe that AI is more than just a tool for driving massive improvements in efficiency, we also think that it can be used as a kind of idea generator, providing sophisticated suggestions to people that can be applied in various fields; in other words, we anticipate that AI can serve as a partner in the co-creation of new products and experiences. For instance, when you face a situation in product development where you can’t focus on a single goal, AI could offer up new ideas from different perspectives. We believe that this will add a new dimension to product development. We are now conducting intensive research and development in both these areas — AI for efficiency and AI for creation. As the world grows increasingly complex and the challenges we face become more difficult, we are hopeful that humans and AI working together can help bring about a safer and more prosperous society.
4. Conclusion
This paper has introduced “Anokorowa CHOCOLATE”, a unique line of delicious chocolates specially created to capture the flavor of specific moments in history through a collaboration between NEC’s AI technology and artisanship of Dandelion Chocolate, a renowned craft chocolate maker. Using AI to analyze and convert newspaper article data into chocolate taste indices, we created recipes for five selected years, which Dandelion Chocolate used to produce the final product. We are committed to continue to offer experiences you’ve never experienced before by leveraging the power of AI and will also continue our efforts to develop AI that will boost human creativity.
References
Authors’ Profiles
Assistant Manager
AI Analytics Division
AI Analytics Division