Global Site
Displaying present location in the site.
Customer Profile Estimation Technology for Implementation of Precise Marketing
The assignment of features to individual products (product DNA as seen from the perspective of the customer) is becoming popular. Although the aim of this technology is to implement marketing based on the hobbies and tastes of customers, it is often accompanied in practice by troublesome issues. NEC’s customer profile estimation features low labor input and yields high accuracy. It is a technology based on NEC’s unique strategy of relational data mining technology that predicts the profiles (job, hobby, annual income, etc.) of all customers, being evaluated from some of entire data. When the customer profiles are enhanced using this estimation technology, it becomes possible to discover the real needs related to buying, display, new product planning and sales promotion for “individuals” who have previously been overlooked. The technology will thereby enable the effective planning of more specific measures.
1.Introduction
It is predicted that the use of big data in applying AI and machine learning technologies will be effective in implementing marketing methods that can adaptively meet different consumer needs (micromarketing).
1.1 Insufficiency of Detailed Customer Profiles
Nevertheless, even when a large amount of data is available, there are many cases in which the “features” of the data are insufficient for an effective use of machine learning. For example, the “features” that are mentioned in the customer data refer to profile information such as age, gender, hobbies and lifestyles etc. Many enterprises already collect basic customer profile data, such as age and gender, but they do not collect detailed profiles such as for hobbies and lifestyles etc.
In addition, even when the basic profile offers a definition such as for a “woman in her 40’s”, this can hardly be considered an effective measure. If a more specific profile such as “a woman fond of traveling” is obtained, the means and channels of promotion can be clarified and their effects can be anticipated. In order to make this possible a detailed profile of the customer is needed.
In general terms, the estimation of a customer profile is the enhancement of the master data (basic data of business elements), which can be classified into the vertical (line) orientation and the horizontal (row) orientation, depending on the orientation of the enhancement (Fig. 1). An example of vertical orientation is to define customer features that are considered to be important for promotion (exercise, annual income, travel, etc.) and then to do a questionnaire on a sample of the customers and estimate the profiles of all customers based on the answers to the questionnaires. This procedure often employs supervised learning techniques such as regression and discriminant analysis (Fig. 2). On the other hand, the horizontal orientation discovers new customer features without estimating them in advance (Horizontal in Fig 1). The method often employs unsupervised learning techniques such as clustering. Below we focus on the vertical orientation.
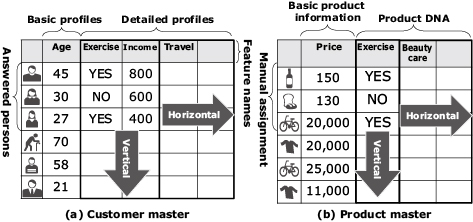
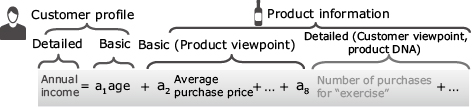
1.2 Product Features from Customer’s Perspective (Product DNA)
The kinds of products bought by a customer can become a reference for estimating the customer profile. However, as for the customer master data, the product master data also only consists of basic information, such as price and product categories, and does not have the information that enables the estimation of customers’ hobbies and lifestyles. Some advanced retail enterprises handle this issue by authorizing human personnel to assign product information from the customer viewpoint (product DNA) to each product. This strategy is aimed at defining a detailed profile of each customer based on product DNA and purchase data (Fig. 2 and Fig. 3).
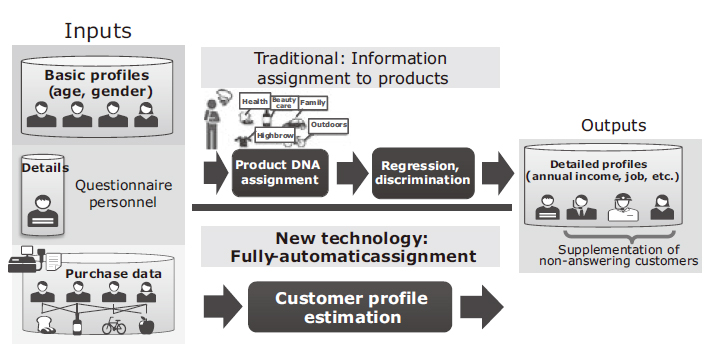
2.Issues
However, the traditional method using the product DNA presents certain issues, the first of which is the volume of man-hours needed for the work. For example, convenience stores and supermarkets handle tens of thousands of products. Several months’ work would be necessary if their product DNA was assigned by human labor. The second issue is that the product DNA is subjectively influenced by the personnel assigning it. For example, some regard low-calorie foods containing artificial sweeteners as healthy products and some see them as unhealthy products.
3.Customer Profile Estimation
To solve these issues, we have developed an AI technology that can automatically estimate the customer profiles without manually assigning features (product DNA) to the products (Fig. 4). This technology employs AI, to replace human personnel in extracting the customer features and the product DNA from the purchase data. It then hypothetically allocates detailed customer profiles by using the extracted data.
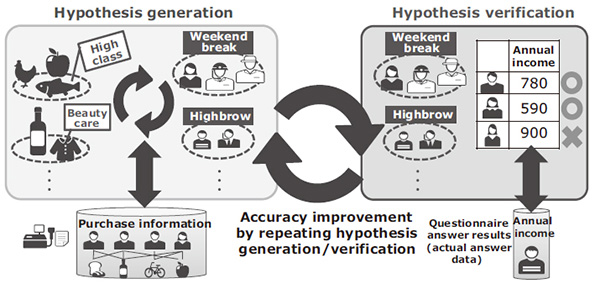

3.1 Generation and Verification of “Hypotheses”
The detailed customer profile estimation is composed of two phases; 1) the phase of hypothesis generation (extraction of customer groups and product DNA) from purchase data, and 2) the phase of hypothesis verification for verifying hypotheses in contrast to the sampling of actual answer data (detailed profiles of sample of customers, obtained via returned questionnaires). The accuracy of profile estimation of this technology is improved by repeating these learning processes in turn (Fig. 5).
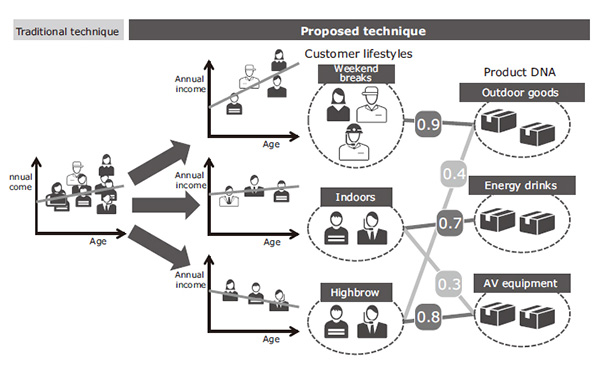

3.2 “Hypothesis” Generation from Purchase Data
In the hypothesis generation phase, the customer features and product DNA information are extracted using the purchase data. Those extracted data are grouped by customers with identical purchase and products with similar purchased patterns. In addition to the occurrence or non-occurrence of purchases, the quantities, time and prices of purchase are also taken into consideration in order to reflect more hidden features, such as responses to new products and price reductions.
3.3 “Hypothesis” Verification Using Actual Data from Returned Questionaires
In the hypothesis verification phase, a model of supervised learning (logistic regression and discrimination) of the customer attributes to be predicted is generated for each of the customer groups obtained as hypotheses. Subsequently, the allocation of the customers from which the real answer data is obtained (in the detailed profiles obtained by the questionnaire) to the customer group is corrected so as to improve the profile estimation accuracy. The correction makes it possible to generate hypotheses in the next hypothesis generation phase with higher accuracy (Fig. 6).
Repeating the hypothesis generation and verification several times as described above makes it possible to estimate detailed customer profiles with higher accuracy.
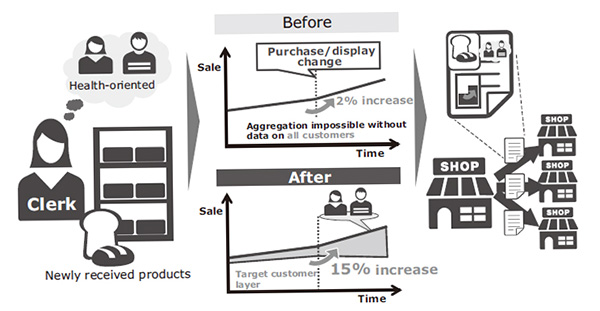

3.4 Support for Product DNA Assignment
In the above, we discussed the scenario about how to estimate the profiles of the remaining customers in case that detailed profiles of some customers are obtained by questionnaire. This technology can be applied to the product DNA assignment. When the product DNAs are already assigned to some of the products manually, the product DNA can be assigned automatically to the rest of products (Vertical in Fig. 1(b)).
The Rolling Ball algorithm is one that supplements the product DNA from that of selected products. This algorithm supplements the product DNA of a product based on other products bought by a customer who also bought the product in question. However, as the influence of products bought by many people (such as eggs and milk) is often exaggerated with this method, it tends to ignore minor, or strongly personal features. Devices such as elimination of data on products with important influence are necessary to alleviate this problem, but this is still an impromptu measure, rather than a radical one. The technology proposed here is free from such a problem because the purchase relationship develops features as hypotheses and the model is created based on the product DNA obtained from the actually answered data.
When this technology was applied to publicized data, analysis was possible in 1/20th of the time and with 6% higher accuracy than for the analysis by experts.
4.Applications for Retail Trade and Marketing
4.1 Application for Business Operation Achieved by Data Visualization
When detailed customer profiles and product DNA are available, visualization of data or rule extraction by machine learning are possible from a different perspective than from formerly. In the following section, we report on an example of applications for marketing activities in retail trade industry.
4.2 Application for the Retail Trade
With in-store merchandising such as merchandise mix, it is important to adopt measures that can promote sales clerk innovations in the field. For example, when a sales clerk receives new products and displays them, a clerk of outstanding ability may decide on an appropriate customer image to her/his store such as a “health-oriented busy member of society” and then display products accordingly (Fig. 7). However, even when the adopted measure is accurate, there is no knowing which layer of customer reacts to it unless detailed customer profiles exist. The accurateness of the measure taken according to the hypotheses can be verified when the corresponding profiles are available. In addition, the possibility of objective measurement of effects from the purchase data permits us to compile a report on effective measures in the field and to share the report with other shops. These hypotheses and verification results can also be utilized in the development of new products.
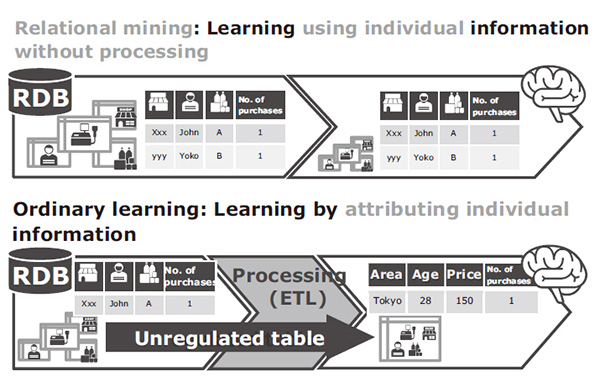

4.3 Application for Promotion Measures
Our technology can be applied to the sign analysis. By analyzing data obtained from communications or credit card members, assessment of their lifestyle information can improve cancellation prediction efficiency. The obtained lifestyle information also helps in considering more specific measures. The predictive analysis target is whether or not one certain customer is likely to cancel a contract, other than predicting the detailed customer profiles in the above case. Since age and gender are insufficient to yield high prediction efficiency, the individual accounts used for deposits and withdrawals by each customer are added to the inputs. As a result, several accounts used by people fond of traveling via travel companies, for example, can be grouped as a common feature, which also allows the grouping of customers in the same way.
If the cancellation rate of a group fond of travel is found to be high in a specific period, it is possible to plan a campaign with the travel company as a measure for preventing cancellation of the corresponding customer layer. In this way, estimation of the detailed customer profiles support design and planning of more specific measures.
4.4 Utilization in Customer Referrals
Ordinary purchase analyses are not capable of analyzing customers who have never bought anything through a sales channel. Moreover, provided that there are multiple channels by which customer IDs can be connected, this technology can group customers based on the product purchase data of the channels they have previously used, so that appropriate products can be recommended to the appropriate customers, even when new channels are needed.
For example, in promoting the use of a department store to customers who use convenience stores but have not used a department store, the use of a department store can be promoted by recommendations chosen specifically to suit each customer from the products available in an optimum department store. The analysis method of analyzing purchases of multiple channels and implementing referrals of customers between channels as described above is called the multi-relational analysis (Fig. 8).
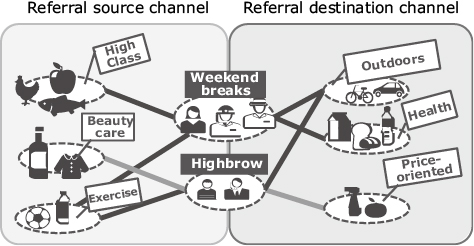
5.Relational Data Mining Technology
5.1 Machine Learning Specializing in Business Data
The customer profile estimation technology introduced in this paper is referred to generically as “relational data mining”. Here, “relation” means a relational data model, which is a combination of predicate logic and set theory. Just as there is machine learning specializing in text or music, this is the one in a relational data model as a form of business information.
An example of the form of business information is the star schema. The star schema is a schema composed of several dimension tables and a fact table. Data is processed in this schema when the BI tool is used for visualization of general business operations. The dimension tables correspond to the business elements (masters) such as products and customers and the fact table corresponds to business achievements such as the purchase data.
5.2 Machine Learning Using Individual Information without Processing It
On the other hand, many machine learning technologies handle data as a single unregulated table instead of using data models. Even when the business operations are represented as relational data models, they aggregate achievements by connecting individual items using keys, such as the shop ID and the product ID. They are then able to perform predictions as features of the shops and products such as price ranges and prices. This process has a variety of attribute design techniques and the processing work is referred to as ETL (Extract, Transform and Load).
Unlike ordinary machine learning technologies, relational mining retains the IDs in the tables, that is, individual information. It performs prediction by estimating the information hidden behind each individual product and shop (Fig. 9). The utilization of business data in learning without altering the information structure makes potential customer referrals as well as sales promotions depending on the context (location, time).
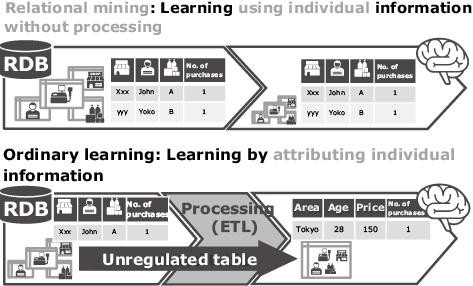

6.Conclusion
In the above, the authors propose a technology for estimating detailed customer profiles, such as by their hobbies, product desirability or annual income etc. With the traditional customer feature estimation technology it has been essential to assign product features. It has been predicted that the use of big data by applying the AI and machine learning technologies will be manually effective from the perspective of the customer (product DNA). Moreover, the proposed technology generates hypotheses on product and customer features that are based on the purchase data. Detailed profiles may then be estimated from the “reliable smart data” collected from the questionnaires, so that the effort of assigning product DNA is not required.
The possession of information on hobbies, product desirability and annual income is expected to support the design of appropriate customer promotions as well as encouraging new product developments.
Authors’ Profiles
NAKADAI Shinji
Principal Researcher
Data Science Research Laboratories
OYAMADA Masafumi
Data Science Research Laboratories