Global Site
Breadcrumb navigation
Significantly Reducing the Time and Effort to Register New Products
Instant object registration for image recognition
Featured Technologies September 30, 2021
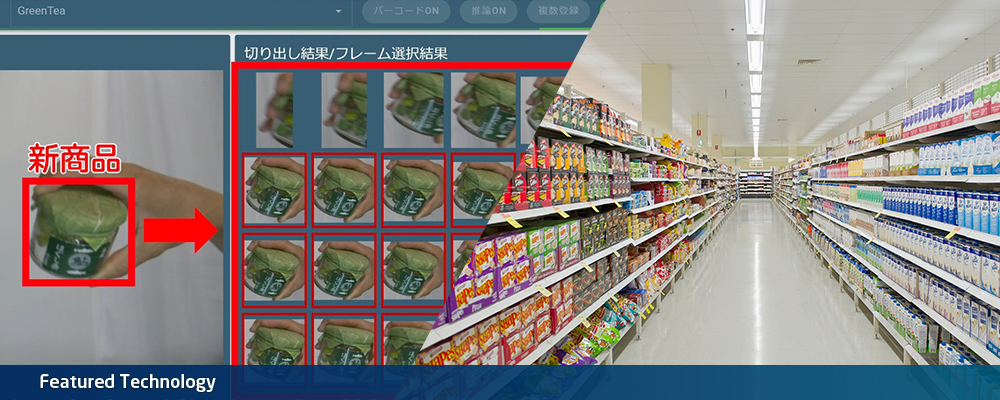
NEC has developed an "Instant object registration for image recognition" that can register new objects in an image recognition model just by rotating the objects in front of a camera. Trials aimed at streamlining work through product management using image recognition and unmanned payment are now starting in the retail and logistics industries where the products being handled change on a daily basis. It is hoped that this technology can dramatically streamline the product registration work in such scenarios. We spoke with two researchers about the details of this technology.
New products can be added to the recognition model just by rotating them in front of a camera
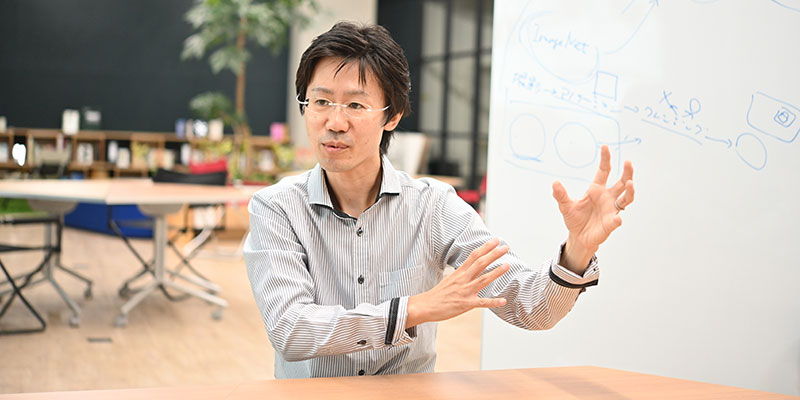
Principal Researcher
Makoto Terao
― What kind of technology is instant object registration?
Terao: It is a technology which significantly reduces the human and time costs when registering a new object in an image recognition model. Anyone can quickly register a new object just by holding it in their hand and turning it in front of the camera. We believe that the new product registration work which is required for product management using image recognition can be dramatically streamlined.
In the past, registering a new object in an image recognition model required a significant amount of work. After an expert had taken several hundred images of one object, an expert called an annotator would inspect those images to remove any data with blurriness or other imperfections to clean it up. In addition, it was also necessary to perform the work to identify the "correct answer" by placing a rectangle on the object that you wish to register within each image using a specialized tool. The work to place the rectangles with sufficient accuracy as learning data without slowing down requires attention to detail. When we created learning data for product recognition, it required about 30 minutes to identify the correct answers for one product. For example, at a convenience store which registers new products one after another, up to 200 hours of manual work per month would be required due to the need to newly register as many as 400 types of products a month. However, with this new technology, the work would be complete just by rotating one product in front of a camera for 10 to 20 seconds. After that, the system automatically learns the images to embed them in the image recognition model. Special skills or know-how are not required, so anyone can easily register new products.
Kaneko: The fact that you can manually rotate a product and take images has significance beyond the ease-of-use. For example, although it is conceivable that you could place a new product on a turntable and take images, this would be unsuitable for recording paper and other flat objects. However, by using your hand, you can easily take images of various sides. Moreover, when considering a bag-shaped product such as potato chips, you can hold it in your hand and take photos in a way which is natural and closer to the actual use to register it in the image recognition model.
Terao: This recently developed technology can register products without relying on the filming location background, so it can be applied to a wide range of scenarios such as managing store shelf products and fixtures or next-generation unmanned payment. In the future, we believe that it can be applied to product management spanning the entire logistics chain from the factory to inventory and stores.
Detecting objects that have not been learned with high accuracy
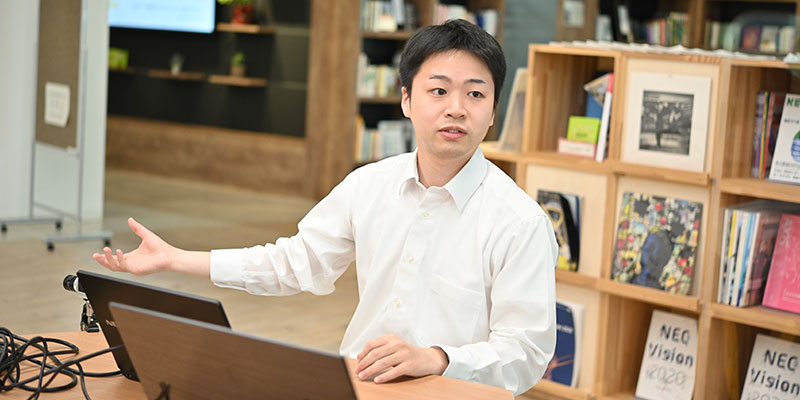
Researcher
Tomokazu Kaneko
― Why is this technology able to register objects in an image recognition model just by rotating them in front of a camera?
Kaneko: While recording video of the rotating object, we extract the frames as images and automatically perform everything from the image cleansing to identifying the correct answers and learning. It is not the case that we are using some kind of special camera. By shooting at 30 frames per second for 10 seconds, we can record 300 images, which allows us to obtain a sufficient amount of learning data to perform deep learning.
We applied AI technology to the data cleansing which extracts only good quality frames from the recorded video. Because subject blurring may occur when recording while rotating an object, we have designed the system to automatically remove any data with significant blurring or poor focus which would be unsuitable for learning.
Moreover, to automate the work to identify the correct answer by enclosing only the object that you wish to register with a rectangle, we utilized a system in which the object is moved by hand. Because the camera shoots from a fixed position, that necessarily means that it is very likely that the area which is moving within the video is the target object. Accordingly, we used a technique called background subtraction to focus only on the moving foreground and developed a system that can accurately detect just the target object. As a result, we were naturally able to detect the object that we wish to register with an extremely high accuracy, and the system can be used anywhere without any dependence on the background. Moreover, because the act of rotating the object in front of the camera itself also matches the essential objective of recording the object from various angles, this process has the advantage of enabling the images to be recorded with a natural workflow.
Terao: A unique aspect of this technology is the ability to detect unknown objects. Because we are trying to create learning data in order to recognize objects which have not been registered in the system yet, it should be essentially impossible to detect and highlight an object that has not been learned yet. Recognizing unknown objects involves the same dilemma as the problem of the chicken and the egg.
As Mr. Kaneko explained just now, what solved this problem was a method which focuses on movement. If you use a general type of object detection technology, it can broadly detect things which "appear to be objects," so it detects the movement which rotates the target object within the video to focus on just the target object.
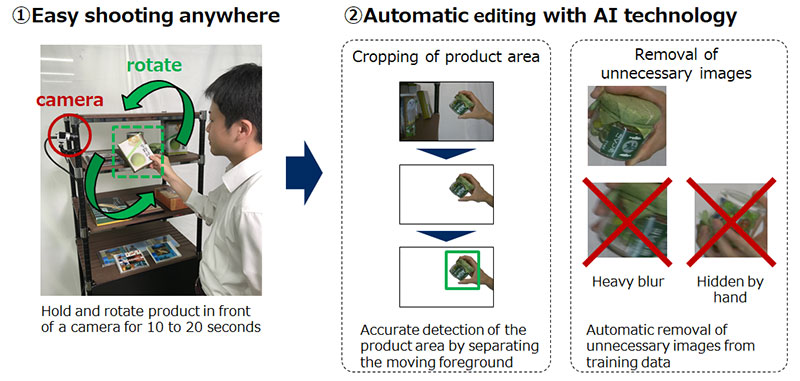
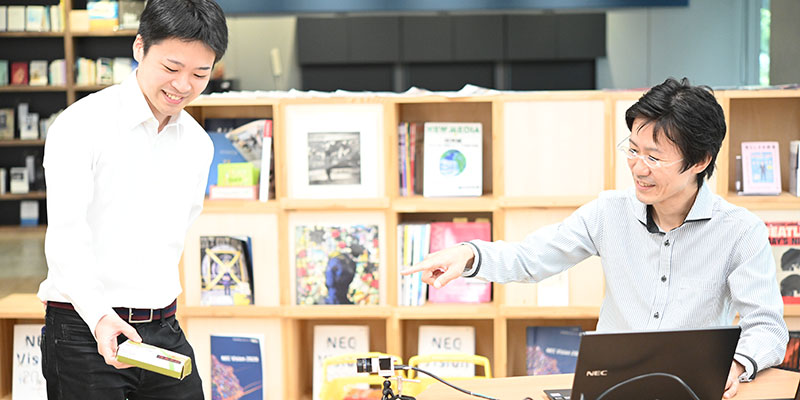
Achieving low cost deep learning with an approach that differs from learning with limited data
― What was the inspiration behind the creation of this technology?
Terao: Our team researches learning with limited data. We have been engaged in researching learning algorithms that achieve highly accurate learning with limited data. One major reason why learning with limited data is required is to reduce the massive cost required to create data for deep learning. Therefore, in addition to advancing the learning algorithms, there must also be approaches which streamline the creation of learning data. However, global research has overemphasized research on learning algorithms until now and paid very little attention to the prior stage of learning data creation.
This may be due to the influence of the AI research environment. In the academic world, public data sets have been prepared with the correct answers and data cleansing already complete. This data is shared around the world with researchers in each country who are continuing their efforts to improve the recognition accuracy of the data. By necessity, the structure focuses on streamlining the learning stage.
In contrast, we drew a sharp distinction from that approach and thought that it would be good to have a technology which automates the data creation process. I believe that thinking about this issue from a different angle is truly the most revolutionary aspect of this technology.
If I were to explain why we came up with this idea, I think that it is because we at NEC have the experience of confronting customer issues and dealing with many sets of live data. Real-world data includes a lot of noise. Moreover, the work to identify the correct answers requires a certain level of skill and a considerable amount of time and cost. Precisely because we confronted this reality and plunged ourselves into that work, we realized the need for automation from our own experience. I think that this technology was born from compelling, front-line needs.
We believe that in the future we can significantly advance the practical application of deep learning by engaging in the research and development of both technologies for automating learning data creation and technologies which can learn from limited data, which is our forte. We hope to test various possibilities in full-scale demonstration experiments which will be carried out going forward.
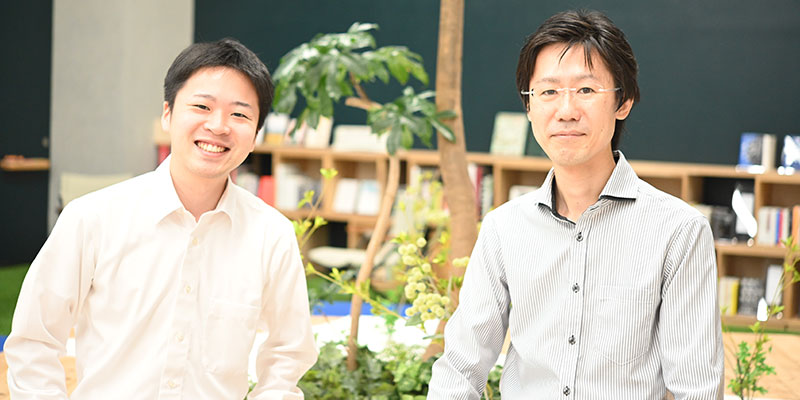
- ※The information posted on this page is the information at the time of publication.