Global
Breadcrumb navigation
NEC develops a deep learning technology capable of accurate recognition even with half the amount of training data required by conventional technologies
Tokyo, Japan, August 19, 2019 - NEC Corporation (NEC; TSE: 6701) today announced that it developed a new deep learning technology that can maintain high recognition accuracy even with roughly half the amount of training data required by conventional technologies.
An effective way of improving the recognition accuracy of machine learning is to use more training data for cases that are hard to recognize, and it is important to ensure that a quality of data suitable for training is available. NEC’s technology intensively creates artificial training data that is hard to recognize. This is done by intentionally changing the feature values obtained in the middle layers of the neural network. Thus the recognition accuracy can be greatly improved, even with a small amount of training data, which helps to reduce the time taken to develop systems that use deep learning.
"NEC's technology reduces the amount of training data needed for deep learning by about half. Furthermore, since this technology is universally applicable to any type of data, there is no need for experts to make any adjustments," said Toshihiko Hiroaki, General Manager, Data Science Research Laboratories, NEC Corporation. "This makes it possible to speed up the deployment of systems for a wide range of applications, such as product inspection and infrastructure maintenance, where the time and expense of collecting training data has until now been a challenge."
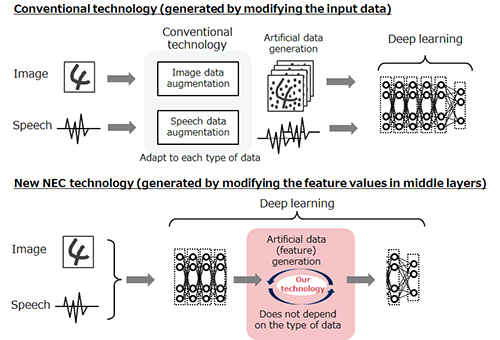

Background
In recent years, deep learning has brought tremendous advances in image recognition and speech recognition, and is widely used in many fields, including security, manufacturing, and infrastructure maintenance. For example, manufacturing businesses want to replace skilled inspectors (who are difficult to recruit) with camera-based image inspection systems. To apply deep learning to the visual inspection of manufactured products, it is necessary to train using data from defective products. But since it is difficult to obtain large numbers of defects that seldom occur, it is very time-consuming and expensive to collect enough data on defective products or to prepare enough data from simulated defects.
This issue has been tackled by using a process called data augmentation, whereby the training data is intentionally modified to artificially increase the amount of data. However, this has not led to the creation of training data that is effective at increasing the recognition accuracy. Furthermore, it has been difficult to apply data augmentation to many different types of data in a short period of time. This is because experts are needed to adjust how augmentation is performed for each type of data.
Features of this Technology
-
Reduces the amount of training data required by about half compared with the conventional technology
It is widely known that an effective way of increasing recognition accuracy is to perform training using larger quantities of training data that is hard to recognize. In the conventional data augmentation technology, the data is intentionally processed and modified (e.g., by rotating the images, making them larger or smaller, or adding noise) before being input to the neural network so as to artificially increase the amount of training data. However, in this sort of augmentation, the quantity of "hard-to-recognize" training data is often insufficient and does not help to improve the recognition accuracy, and so adequate recognition performance is not achieved.
In NEC’s proposed technology, the feature values obtained in the middle layers of the neural network are intentionally modified instead. This increases the recognition accuracy by artificially generating hard-to-recognize training data that is more likely to cause recognition errors. This technology has been used to evaluate public datasets for handwritten digit recognition (MNIST) and object recognition (CIFAR-10), and confirmed that there is no change in accuracy even when the amount of training data is halved.
-
No need for adjustments by experts to accommodate different data types
In conventional data augmentation, the data generation method had to be changed for each type of data. For example, this might involve artificially rotating or rescaling images, or changing the pitch or speed of speech samples. Furthermore, experts were required to carefully select the data generation methods and make adjustments without producing any data that might have an adverse effect on training.
Since this technology generates training data automatically based on the neural network’s internal values, it can be universally and efficiently applied to a wide variety of data and eliminates the need for expert adjustments.
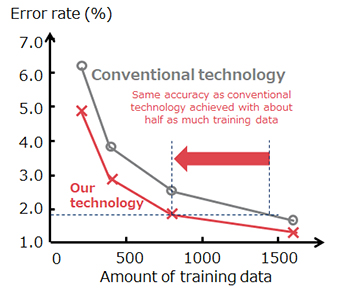
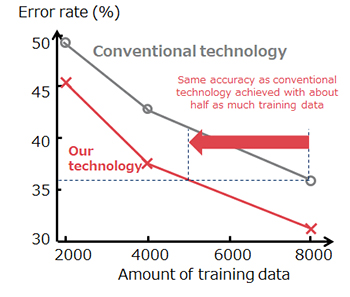
Results of evaluation using public datasets
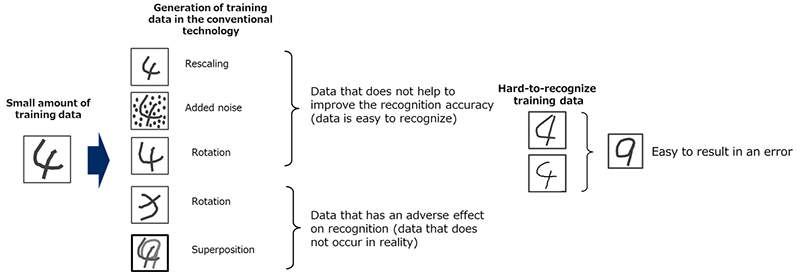
The results of this research were presented at the International Joint Conference on Neural Networks (IJCNN2019), which was held in Budapest, Hungary, 14–19 July 2019 (https://www.ijcnn.org/)
***
For More Information
Small data learning technology for deep learning enables highly accurate learning
https://www.nec.com/en/global/rd/technologies/201910/index.html
About NEC Corporation
NEC Corporation is a leader in the integration of IT and network technologies that benefit businesses and people around the world. The NEC Group globally provides "Solutions for Society" that promote the safety, security, efficiency and equality of society. Under the company's corporate message of "Orchestrating a brighter world," NEC aims to help solve a wide range of challenging issues and to create new social value for the changing world of tomorrow. For more information, visit NEC at https://www.nec.com.

LinkedIn: https://www.linkedin.com/company/nec/
YouTube: https://www.youtube.com/user/NECglobalOfficial
Facebook: https://www.facebook.com/nec.global/
Twitter: https://twitter.com/NEC_corp
NEC is a registered trademark of NEC Corporation. All Rights Reserved. Other product or service marks mentioned herein are the trademarks of their respective owners. © NEC Corporation.