Global Site
Breadcrumb navigation
Understanding disaster information by asking simple questions without needing specialized knowledge
Featured TechnologiesMade possible by combining large-scale language models (LLMs) and satellite dataFebruary 28, 2025
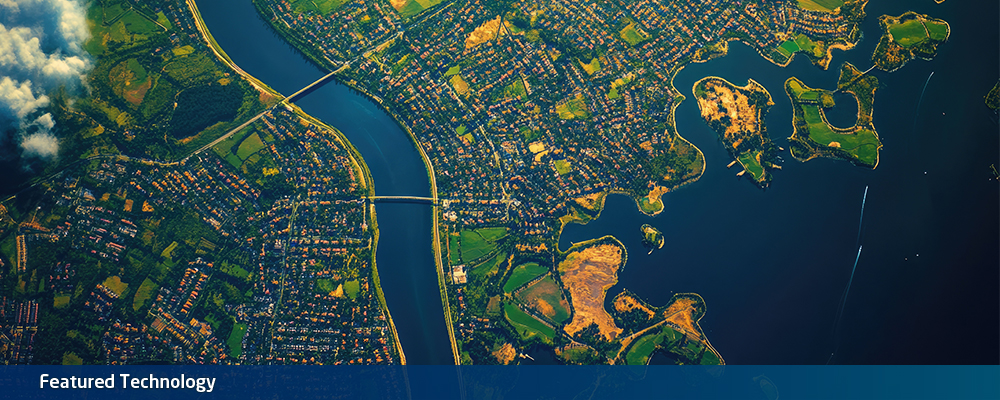
While the use of satellite data to understand disaster situations has attracted attention, the analysis of satellite data requires a high level of expertise. Thanks to technology developed by NEC's Japanese and US teams working together, satellite data and LLM (Large Language Models) have been fused together to enable expert-level analysis from large volumes of satellite data without the need for advanced expertise.
Thanks to technology developed by NEC's Japanese and US teams with expertise.
NEC's technology enables expert-level analysis of satellite information without the need for special expertise
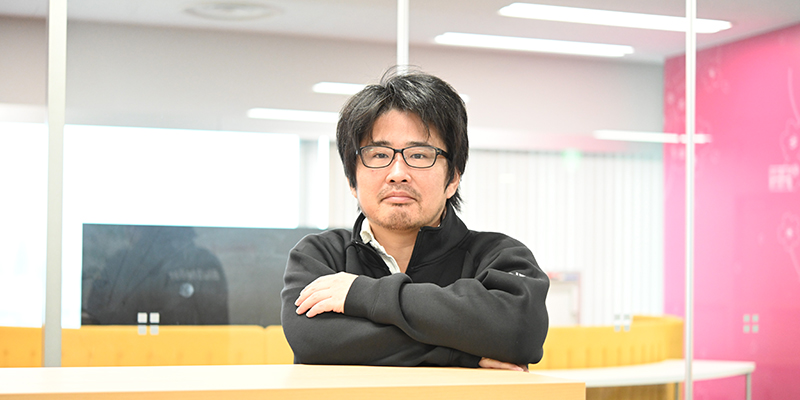
Professional
Masato Toda
―Please provide an overview and benefits of this technology, the integration of the satellite image data and LLM.
Toda: This technology is designed to present information derived from satellite images to users in an easy to understand manner through language. The system combines satellite image analysis technology and LLM (Large Language Model) so that the user simply enters a query and the system gives a clear answer based on the information available from the satellite data.
Eric: The ability to process multiple data in a single user interface and to handle intuitive natural language are key features. Because of a simple interface, it becomes more accessible to a large number of customers with little or no training. Although typical tools to extract insights from this type of data are complicated and take time to learn for users, our system, users only need to enter a query: no specialized knowledge is required.
Murugan: The first benefit is that it enables a deep understanding of events such as disasters or infrastructure-related changes through satellite data interpretation.
The second key benefit is the fusion of this aerial and ground-based data streams allows for precise situational awareness. And the third one is it enables dynamically question and refine their insights by improving the relevance and then their decision making based on the response to the queries.
The fourth key benefit is our optimized multimodal retrieval and augmented generation technique for the AI technology. It ensures faster and more accurate information retrieval for time scenarios as the situation is evolving on the ground.
The last benefit is it supports tailored solutions across different industry domains, for example, insurance, energy and disaster management.
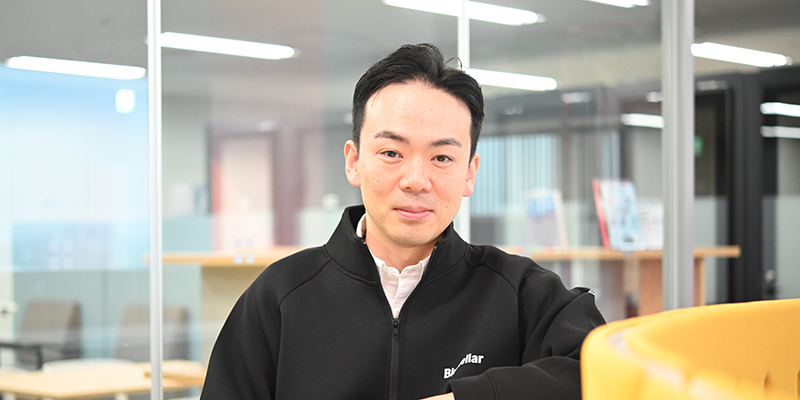
Professional
Kenta Senzaki
―What is the background behind the development of this technology?
Please share the motivations and the necessity of developing it.
Senzaki: The satellite images can observe areas spanning tens of kilometers by tens of kilometers at once, enabling comprehensive understanding of entire cities and towns. They are considered to be valuable sensing data with potential application in various scenarios, such as disaster situation understanding or disaster damage assessment.
On the other hand, manually analyzing such large areas is challenging. Specifically, synthetic aperture radar image, a type of satellite data, requires expert skills for interpretation. We believe that this complexity in analysis limits the wider use of satellite images.
Therefore, we developed this technology to make it easier for those unfamiliar with satellite images to access information easily.
Biplob: One of the key motivations behind our technology is the increasing frequency of natural disasters, the impacts of climate change, and the growing need for infrastructure monitoring, all of which demand advanced, scalable solutions for accurate and timely decision-making. While traditional satellite imagery offers valuable data, it often lacks the necessary contextual depth.
The vast volumes of geospatial data can overwhelm analysts, leading to data overload and challenges in interpretation. Our technology addresses these issues with flexible, open-vocabulary geospatial recognition and retrieval-augmented generation (RAG) systems, specifically tailored to meet the needs of various sectors. By leveraging large language models, we bridge the gap by interpreting complex information, extracting relevant insights, and enabling interactive analysis.
Diverse uses, including disaster management, insurance compensation, and carbon credits
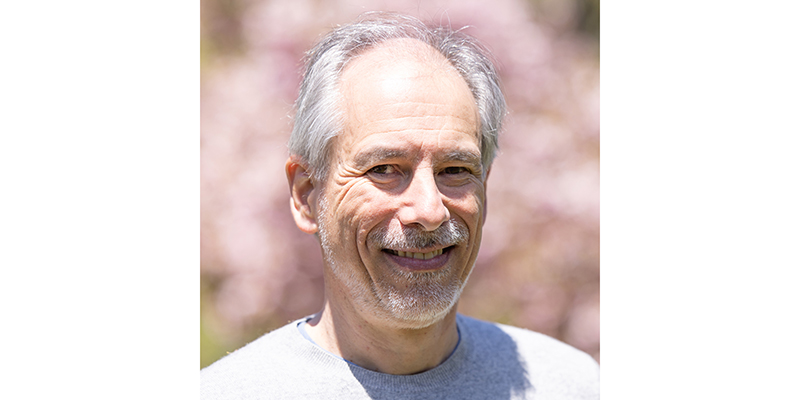
Senior Researcher
Eric Cosatto
―Could you provide the use cases?
Eric: We have envisioned several scenarios for use cases. One is disaster management. For example, an earthquake strikes and you want to have a quick assessment of what's going on the ground. Our technology enables fusion of multiple data sources, such as earth observation from satellites and from ground level data, such as video from users, from social networks.
And, owing to the intuitive, easy-to-use interface, a responder, even without much training can quickly ask, "what's the area that is the hardest hit?" and then the system would show you some videos of destroyed buildings after the earthquake.
Also, after a disaster, if many houses are destroyed, quickly have an estimate of how many houses have been destroyed, what's the level of destruction, is it severe. This is the scenario we have in mind for disaster management. Furthermore, it can be applied to the assessment of post-disaster property insurance coverage.
Our technology can also be used for trading carbon credits. Carbon credits are a mechanism for granting credits for reductions in greenhouse gas emissions and making them tradable, mainly between companies. Monitoring can be carried out to see whether people claiming to have planted trees to offset carbon credits have actually planted trees or whether they have really installed solar panels to supply renewable energy.
We can use our system to find out if they have planted as much forest as they claim to have planted, and if the solar power they claim to have installed is as large as they claim, and how many MW it can generate.
Biplob: Let me elaborate on how our technology benefits the insurance industry. For example, our solution uses generative AI to analyze satellite images and assess property damage by comparing pre- and post-natural disaster imagery.
This comparison enables us to quickly identify pre-existing damage, helping insurance companies accurately detect fraudulent claims. Additionally, with detailed damage assessments, insurers can take quick action to optimize manpower deployment and prioritize their workforce, expediting claim processing and improving efficiency.
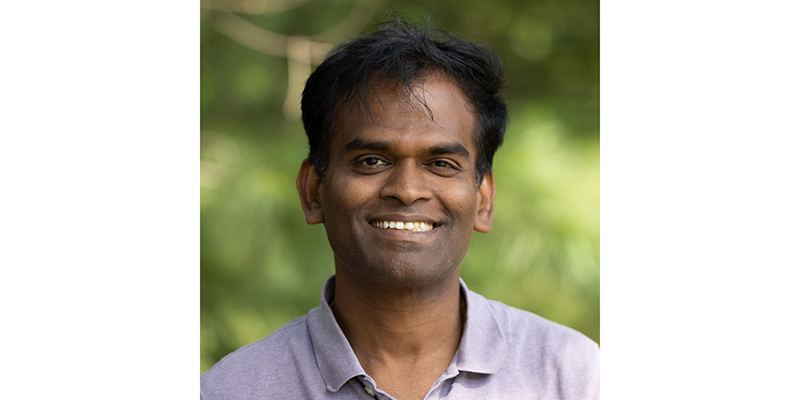
Senior Researcher
Murugan Sankaradas
―Are there any other similar approaches by other companies and competitors?
Eric: Many companies, of course, that provide geospatial data services are starting to consider the use of LLM in their services and products. There is potentially lots of competition. But NEC has several strengths. One is large and broad experience with AI models. In addition, the vertical concentration of know-how from satellite providing to image analysis as well as data services to customers. We are well situated to make an impact in this field.
Murugan: Comparing to competitors, we believe that NEC's technology using this quantitative vision language model capability and also using some of the innovative retrieval-augmented generation (RAG) technology can give a better query accuracy, interactive query response capability as well.
Close cooperation between Japan team and the US team
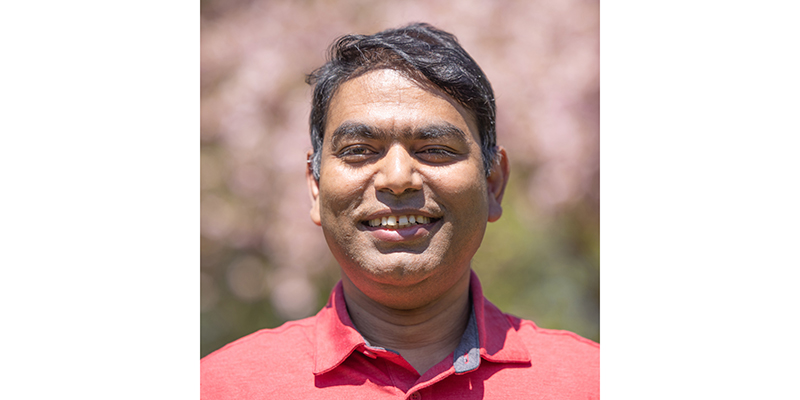
Senior Researcher
Biplob Debnath
―What are the key factors for making the U.S. and the Japan teams successfully collaborate as one team to develop new technologies?
Toda: There are many people with various personalities. But in this project, I believe that it is significant that we are able to share the vision and the value we want to provide.
Biplob: We leverage the expertise of our Japan team to gain a deep understanding of customer pain points. Our teams maintain frequent communication online and meet face-to-face on multiple occasions to strengthen collaboration and deepen our understanding. Through close interaction between our US and Japan teams, we gain valuable insights into our customers' needs.
Once we identify customer pain points, we develop technologies to address them. We then invite customers to review the improved solution, gather their feedback, and implement further refinements. This iterative process enables us to continuously improve our offerings. By repeating this cycle of refinement, we create technology that is not only highly effective but also intuitive and user-friendly for our customers.
The next step is to integrate more data to give customers access to better information and help them make faster decisions.
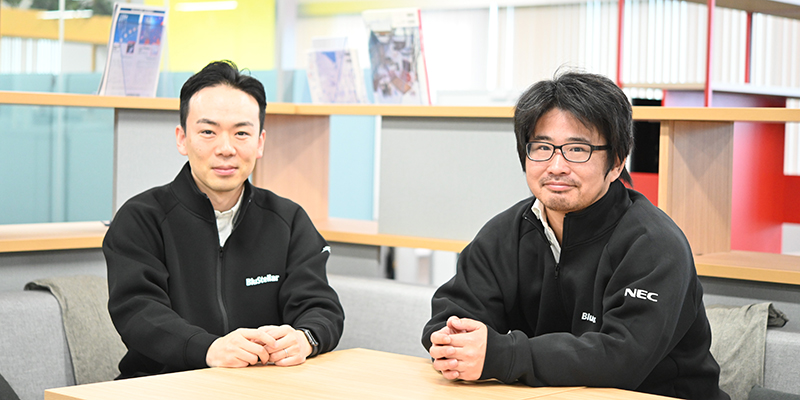
―Are there any areas where you aim to further develop the technology or next steps that you plan to pursue?
Senzaki: We are considering integration with more data sources.
On the sensing side, we would like to accelerate the utilization of ground image data and also consider leveraging open documents published by local governments or national government and some companies as well as increasingly developed three-dimensional urban data.
On the other hand, because the data linked to geospatial information is extremely diverse, we recognize that there are many challenges in the technology needed to appropriately extract the information users want from it. We aim to advance this integration and expand the possibility of the technology.
Eric: The next step is to identify key areas and customers, and really collaborate with the customers. What can we do with our technologies and make them understand how we can help them with our product and services and so then we collaborate with them to scale it up into real full products and services. That's our next plan.
Biplob: Our next step is to move beyond just images and integrate as many data sources as possible, enabling customers to access more accurate information and make quicker, more informed decisions. For example, we can integrate documents, web information, and domain-specific knowledge alongside satellite images to create a comprehensive multi-modal data integration platform. This integration empowers customers to make faster decisions by leveraging a unified framework that leverages the strengths of various data modalities.
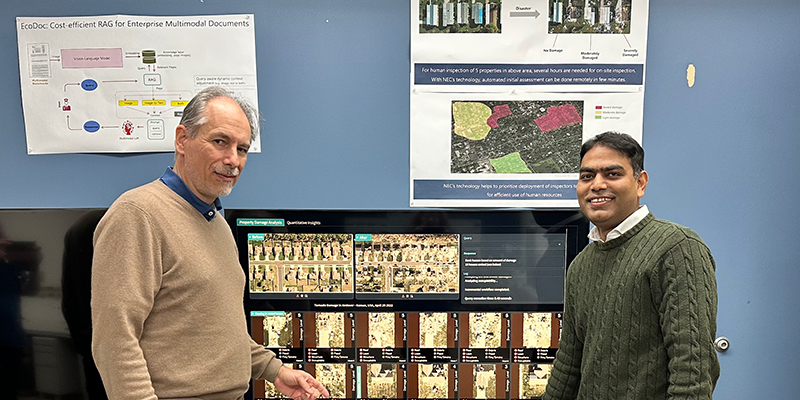
- ※The information posted on this page is the information at the time of publication.