Global Site
Breadcrumb navigation
“World models” evolve even further
AI technology for robotics enables environment-adaptive precision motion
Featured Technologies February 19, 2024
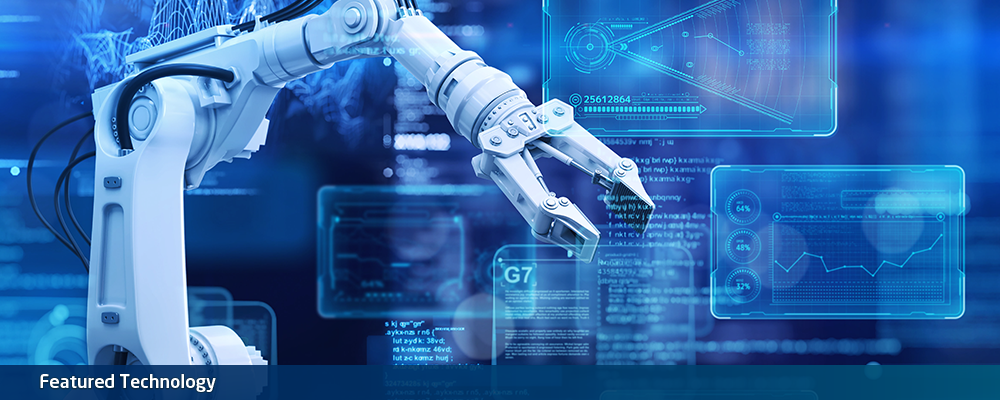
In March 2023, NEC presented an innovative robot motion learning technology that enables robots to autonomously select optimal behavior. What is at the core of this technology is the world model technology. World models are machine learning frameworks in which the AI learns about the robot and its surroundings (world). This technology has been recently garnering attention for its potential to drastically evolve AIs. By applying a world model to an AI, the AI can predict and simulate what is going to happen in the real world, which then allows the robot to autonomously optimize its motions.
Now, NEC succeeded in further enhancing robot control using world models. This approach enables robots to make more flexible and precise movements and applicable to a wider range of settings. Specifically, what can AI do now and how were they achieved? We spoke with the researchers about the details of this technology.
Combined with image recognition, world models make possible higher-accuracy predictions and motions
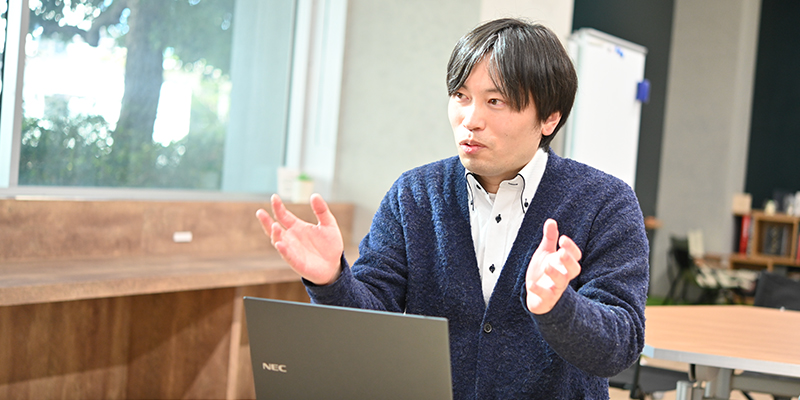
Principal Researcher
Hiroyuki Oyama
―First, let’s review what a world model is.
Oyama: A world model is a machine learning framework that enables prediction and estimation of real-world structures and consequences of actions. Real-world structures mean, for example, predicting that there is something behind a wall when part of it is peeking out. We humans generally act on such predictions and inferences. Adopting such “human subconscious common sense” to robots is what we are working on at NEC. This enables robots to adaptively function in first-time environments that they haven’t learned yet.
―In what ways is this new technology evolutionary?
Oyama: It makes robots perform more complex, more diverse movements based on more sophisticated predictions. We received inquiries from many customers after publishing the previous technology, among which many asked whether “packing” is possible and whether robots can respond to situations where things are disorderly placed. “Packing” means to pack things into a box in an orderly fashion. Unfortunately, at the point of previous release, our technology was not capable of packing or dealing with situations where things were not organized. This owes to the fact that robots were unable to perform “pushing” and “pulling” movements. In fact, it is quite difficult for robot arms to accurately push or pull an object to a given position.
Sakai: Unless the arm holds the center of the object, that object will turn. If the arm fails to identify the center of gravity, it can fall. While we humans can control our grip to push or pull objects while using the senses at our fingertips, doing the same is extremely difficult for robots.
Oyama: That’s right. So this time, we conducted research in collaboration with Mr. Shiraishi and Dr. Sakai's team, which specializes in image recognition. Our team specializes in robot control, but since we knew our limitations in what we could achieve with just that, we had been preparing for the collaborative study from an early stage. AI technology broadly works in three steps: visualization, analysis, and control. We further deepened the world model by coordinating with the process of visualization and analysis. As a result of this approach, the new technology achieved significant improvement in the accuracy of predictions based on the world model. The new technology enables a robot arm to perform delicate movements such as push and pull in addition to the typical pick-and-place motion. Not only can it perform packing, it can also be introduced right away into environments that are not organized for robots. For example, this technology saves operators from having to make space between things and prepare special equipment or systems to make it easier for robots to work. And of course, there is no need to teach robots with detailed programming and instructions. You simply need to put the robot in its work site and leave it to self-adapt to the environment. Moreover, robots can also adaptively respond to any minor changes in the environment such as an increase in objects or object shapes.
Autonomous handling operation using world models
Image training facilitates response to unexperienced events
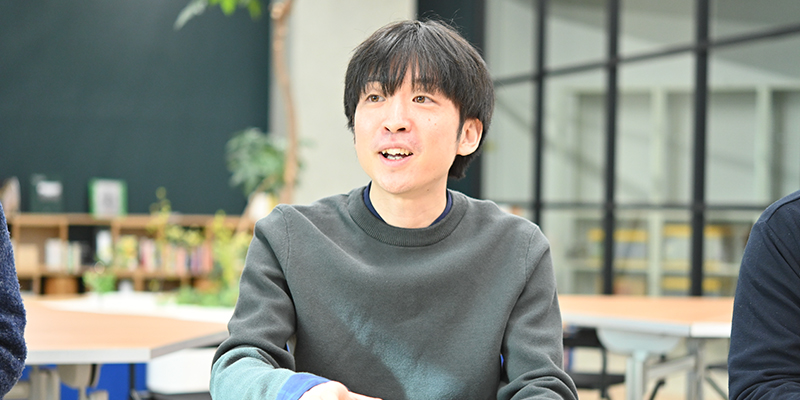
Researcher
Ryosuke Sakai
―Specifically, what ideas and innovations led to the development of this technology?
Shiraishi: In addition to control technologies, the successful coordination with image recognition to more correctly understand the situation at hand contributed greatly to the development of this technology. Standard recognition technologies that we study on a daily basis are intended to return “correct” results to we “humans” as they are pre-taught. In other words, previous technologies were required to show the presence of people and things using frames or coordinates or other such indicators that humans can understand. With the case of the new technology, however, the AI needs to pass on information needed by robots for them to perform tasks. After recognizing physical objects, this new technology predicts what will happen when the robotic arm touches them. Forming the predictive results into something that can be used for robot control and giving that information to the robot was the greatest technical challenge―a challenge of a totally different kind.
Sakai: In order for robots to accurately control objects with robot motion, they need to accurately receive as much recognized information as possible about the actual environment. To accomplish this, we were considering passing on specific numerical values such as coordinates and sizes to robots at first, but since, in the nature of things, things come in a great variety of shapes, it was impossible to numerically represent everything. As such, we worked on encoding images and applying them in the form of features to robot control.
Oyama: The key considerations for the control team were what information to receive for control and what information would make it easy for robots to learn motions. We had close discussions while sharing such information.
Shiraishi: Another key point is that we used a massive amount of data for teaching in order to achieve recognition of objects that are hidden behind other things as well as accurate predictions.
Sakai: For the purpose of improving predictive accuracy, in order to teach the AI how to move when the robot hits something in a certain way, we need a large volume of pattern data concerning different contacts, collisions, shapes of things and such. This requires patient, steady efforts to acquire the necessary massive data through making the actual robot perform different tasks. Not only that, we also enhance and enrich data by combining the use of an original simulator in order to teach a diverse range of data. The build-up of these efforts led to the development of the new technology.
Shiraishi: This is exactly the same process that people go through when they are learning something new. First, you keep looking at successful cases along with failed cases to learn them. This then creates a model inside your head, allowing you to imagine “what will happen if you do this.” Image training will be possible in your brain to figure out what actions are necessary to make things happen. This is exactly what a world model is about. If we can create good models through the approaches that Dr. Sakai explained, the AI can build up accurate imaginations and use the data it learned to generalize and respond to matters that it has never encountered or learned before in the exact same way.
Spatiotemporal prediction using world models
Oyama: This output information is also efficiently utilized in control. Like the previous technology, the new technology also allows AI to make decisions on its own regarding optimal movements and order of movements. On top of that, the new technology offers faster computation for such decision making. Specifically, a technology called diffusion model, which is a type of generative AI, is used to generate trajectories. Additionally, in order to enhance the precision of trajectories generated using the diffusion model, we used the aforementioned technique that automatically optimizes task order to efficiently correct and generate the trajectories.
Robot motion generation based on world models
From a closed world to a wider world: Generalizing world models
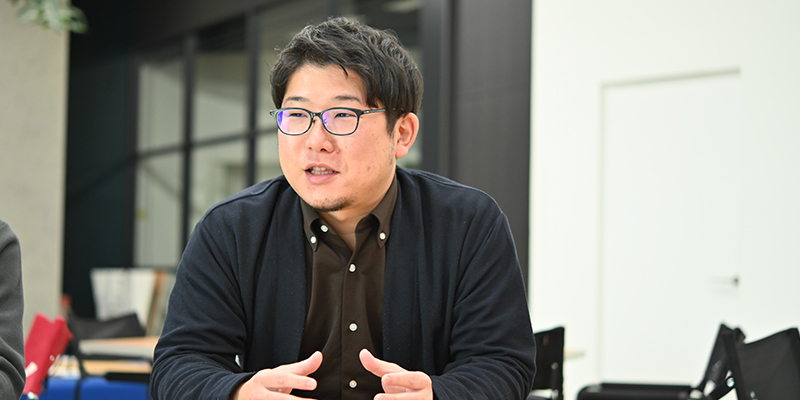
Principal Researcher
Soma Shiraishi
―What are the advantages of being able to recognize things that are hidden behind other objects?
Shiraishi: The spatial predictive ability to “imagine things that are hidden” is one of the core functions of a world model. As a matter of fact, in reality, objects appear overlapped and not fully visible to cameras in most cases. In order to leverage adaptive control on site, you can say that it is an essential function. The world model technology that we are developing observes a massive amount of images of objects being moved beforehand and interprets their boundaries and overlaps to enable estimation of overlapped, hidden shapes. Humans can subconsciously imagine hidden shapes and naturally pick up things, and this technology empowers robots to do the same.
Oyama: When robots can consider hidden shapes, they can start planning more adequately, such as “temporarily moving the objects in front in order to get to the larger object behind them.” Furthermore, while robots had to re-plan pickup every time they found a new hidden object, this technology can improve such behavior and eliminate such inefficient tasks.
From a closed world to a wider world: Generalizing world models
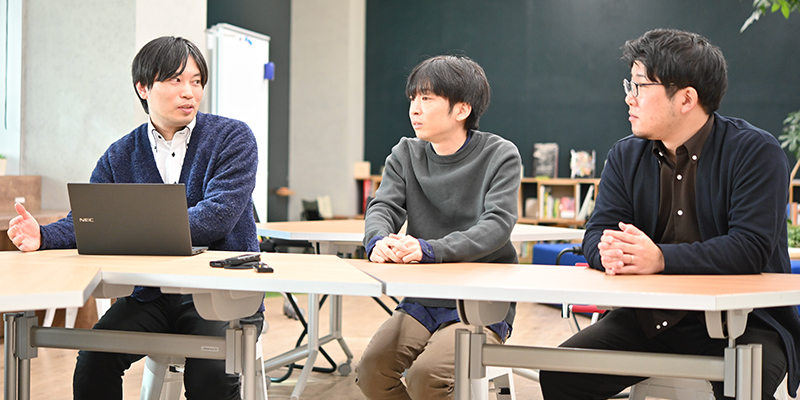
―Do you plan to continue working on projects that utilize world models?
Shiraishi: Yes. We do. From the perspective of my image recognition team, through this research, we felt that world models can contribute to the improvement of the accuracy of image recognition itself. We would like to look into world models from that approach as well. More specifically, we can utilize the technology to produce more physically accurate recognition results, ruling out the implausible. For example, when a person is behind a car and only his face is visible, recognizing it as “a presence of a face” or “a presence of a person” is a totally different matter. Current image recognition registers such picture simply as “a face being there” (or a face in mid-air), but this is not realistic. Under the face, there is a body―being able to acknowledge that a human being is there according to common logic can further step up the level and practicality of image recognition.
Sakai: What I am interested in now is the generalization of world models. Currently, we are collecting data for necessary tasks to use for teaching, but when world models become able to imagine a broader world, it may reduce such hassle of teaching AIs.
Shiraishi: Yes. Just now, Our team are testing the online updating of world models. If this proves successful, world models can be more easily adopted at various sites. For example, a model that works well for one warehouse may not perform well at another site due to inaccurate predictions caused by major environmental differences such as stronger friction and oil-slicked slippery surfaces. In such cases, the conventional method calls for a redo in AI learning. However, if we can update world models online, the accumulated data should facilitate adoption to different sites. We are currently working on such technologies while also ensuring data security as a precondition.
Oyama: We are also contemplating combined use with large language models (LLMs) and vision-language models (VLMs). World models and LLMs/VLMs share lots of similarities, and I feel that the possibilities are huge when you combine them. It will be very beneficial especially in terms of environmental recognition and understanding. I believe that it is also very effective for generalizing models. I am also envisioning a future where we develop it into a foundation model that can be applied to not only robot arms, but also other robots and systems, and combine it with the online update scheme that Mr. Shiraishi mentioned earlier.
From a robot control point of view, we would also like to work on building a large-scale system where robots collaboratively work on tasks. If we can have multiple robots working together instead of a single robot working alone, that will increase task efficiency. However, at the same time, we focus on not only collaboration among robots, but also collaborative robots that work with people. Development of work sites where robots recognize the movement of people around them and work efficiently alongside humans is a theme that I would like to work on in the future. Image recognition technology continues to be important in that sense, so we will work on projects by collaborating across teams.
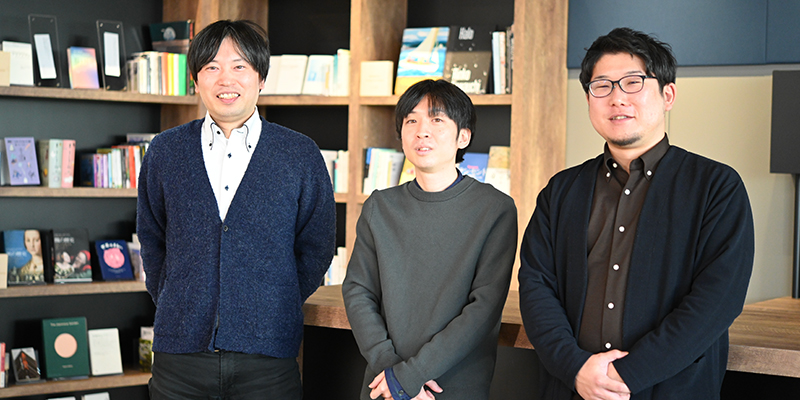

The robot motion learning technology applying world models infers background factors from limited data and applies to robotics a world model that predicts how the world may change in response to a certain behavior. Since there is no need to teach detailed rules to robots each time, robots can decide optimal behavior based on learned or sensed data for autonomous operations.
In March 2023, as robot control technologies, NEC released a technology where the AI learns a model that predicts the success or failure of an action and a technology for efficient learning by selecting data that contributes to improving accuracy. In this release, researchers skillfully combined these technologies with image recognition to achieve improved prediction accuracy and precise motions. While robot control studies that apply world models are recently garnering attention, what makes this research exceptional is that it achieves practical high-accuracy predictions that can be applied to the real world, enabling actual robot actuation.
- ※The information posted on this page is the information at the time of publication.
Related information
- February 19, 2024 Press Releases
NEC develops AI technology for robotics capable of autonomous and advanced handling of disorderly placed items - March 3, 2023
Robots autonomously selecting the optimal behavior Robot motion learning technology applying world models - March 3, 2023 Press Releases
NEC develops robot control AI that flexibly responds to changes in warehouse operations and layout