Global Site
Breadcrumb navigation
Streamlining Private 5G Operation with Learning-based Wireless Quality Analysis Technology
Featured TechnologiesJune 7, 2023
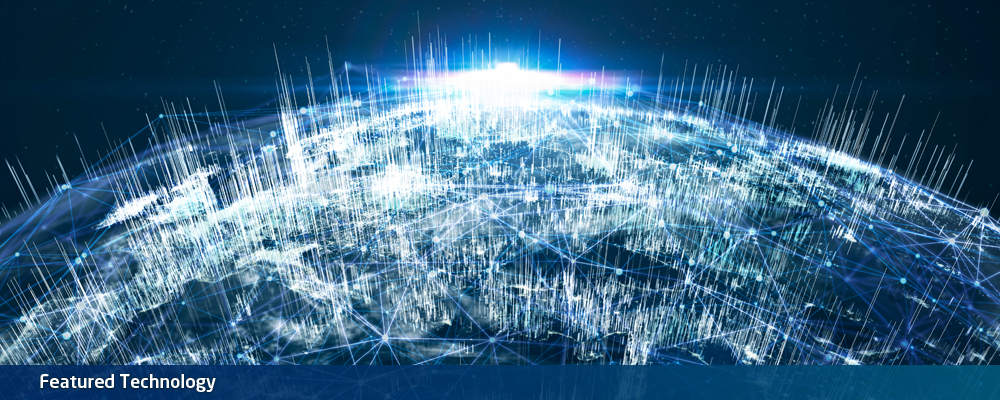
5G networks are essential to Digital Transformation (DX) and Internet of Things (IoT). In recent years, an increasing number of companies have been independently creating private 5G environments. However, the question of how far costs can be reduced is an issue in the operation of private 5G. The learning-based wireless quality analysis technology announced by NEC addresses this issue and achieves wireless quality monitoring at a low cost. We spoke with three researchers to learn more about the details of this technology.
Automatic and immediate identification of the root causes of communication performance degradation
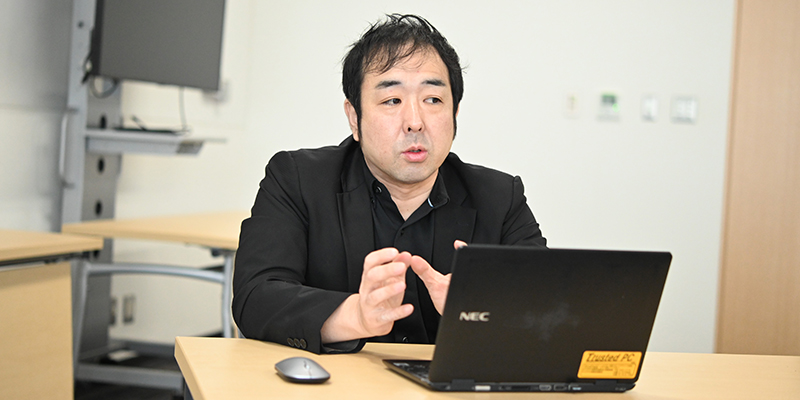
Director
Takanori Iwai
-- What kind of technology is the learning-based wireless quality analysis technology?
Iwai: It is a technology which streamlines private 5G operation with AI. With the progress of DX and IoT, there has been a growing movement in recent years to independently create 5G environments at plants and construction sites. NEC provides private 5G to various customers, and careful fine-tuning is essential for maintaining the high communication performance which is unique to 5G. This is because radio waves can be shielded or reflected, etc. at plants and construction sites due to layout changes, movement of equipment, work progress, and other changes which result in the unexpected degradation of communication performance. Moreover, when utilizing communications in applications such as the automatic operation of machinery, the degradation of communication performance directly leads to a decrease in productivity. To prevent such degradation from affecting the business, we needed to monitor the wireless quality, immediately identify the root cause when a problem occurred, and address the problem.
Takahashi: However, the process of identifying the root cause and addressing the problem currently requires the implementation of a detailed investigation and response by an expert. This can take up to several weeks, and the majority of that time is often spent on analyzing the root cause.
The learning-based wireless quality analysis which we recently announced is a technology which automates and streamlines this root cause identification. This technology is able to constantly visualize the wireless quality on a map and immediately identify and indicate the root cause when the communication performance degrades. As a result, it enables private 5G operation to be more stable and efficient.
Realizing automation at the minimum cost
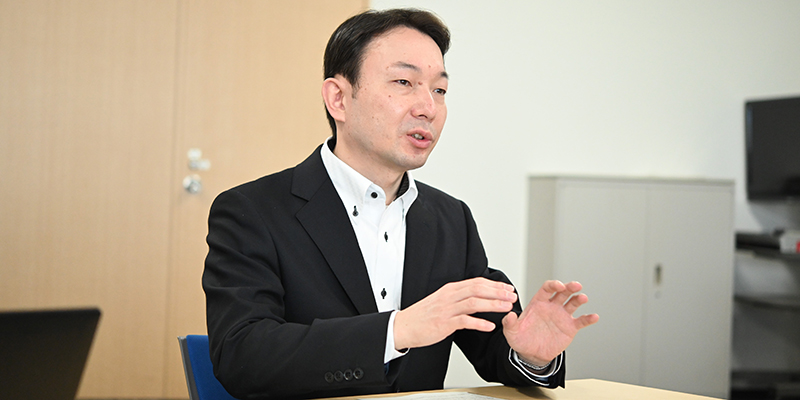
Senior Research Architect
Eiji Takahashi
-- What is the mechanism used to realize this automation?
Takahashi: Broadly speaking, this technology consists of two elements. One technology visualizes the wireless quality in real time. In the past, if you wanted to create a map showing the radio field strength, a worker had to walk around the site carrying a sensor each time, which required a significant amount of work to display the measurement results as a graphic. With this method, it is impossible to keep up with changes in the site environment. For this reason, it was first necessary to constantly sense and monitor the wireless quality at the site.
Nishikawa: That being said, if you focus only on the mapping of the communication conditions, there are many previous research studies. What is important about this new technology is that it achieves the visualization at a low cost. In private 5G environments, there are limitations on the cost and space available network operation. You cannot just install expensive sensors all over a plant or construction site to observe the communication conditions.
Therefore, we developed a technology which can create a map using only the radio field strength information collected from the minimum number of observation points. The unique approach of this technology is that while it creates a map through detailed sensing of the site conditions during the initial implementation similar to conventional methods, it abstracts the features of the radio field strength distribution which are determined by factors such as the floor-specific reflections and diffraction as well as the relationship to the base station at this time. During subsequent operation, this technology makes it possible to visualize the wireless quality in real time at the minimum cost by applying corrections based on this model and the information from the sensors installed on site.
Takahashi: The other technology automatically identifies the root cause of communication performance degradation. In public 5G networks, there is a need to analyze the communication performance trends in each service area across the country and make overall improvements. However, private 5G networks require an analysis with a higher temporal and spatial resolution. This is because each piece of equipment and application operating at the site must demonstrate a high level of performance without interruption rather than the macro level of quality for a large number of users, which becomes a problem in public 5G networks.
At the same time, as Nishikawa discussed a moment ago, countermeasures were needed in a form which optimizes the costs. Just collecting a large volume of logs requires a certain cost, so we needed to identify the root cause using as little information as possible.
Nishikawa: Therefore, we developed an AI technology which can identify the root cause from the variability of commonly available radio signal strength indicator. We compensated for the lack of training data by utilizing a simulator to make it possible to identify the root cause with high accuracy using machine learning.
Takahashi: The technology is able to identify the root cause from variability of the radio signal strength indicator by using a simulator to recreate many of the phenomena which occur on site and training it in advance. Another technique that we used was instead of having the AI fully process the information at one time, we established intermediate indicators and processed the information in stages to increase the accuracy of the final root cause identification.
Nishikawa: In addition, this technology won the Best Paper Award at the 23rd Asia-Pacific Network Operations and Management Symposium (APNOMS2022).
Research with the goal of realizing everything up to automatic optimization
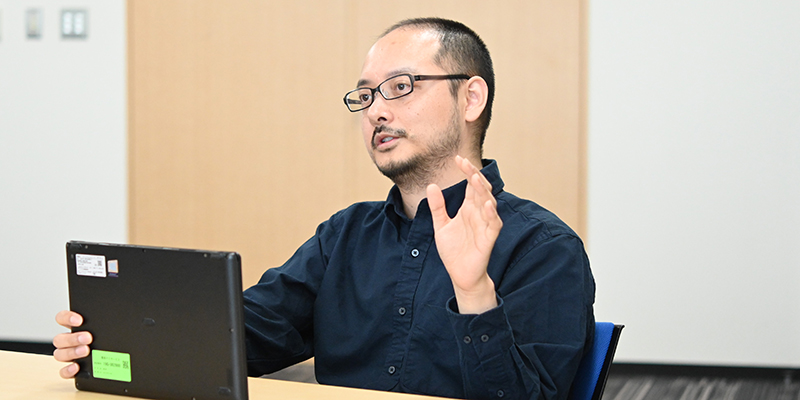
Senior Researcher
Yoshiaki Nishikawa
-- Why was NEC able to develop such a technology?
Iwai: Our team attracts many personnel who have experience in various divisions. Both Takahashi and Nishikawa were involved in LTE and Wi-Fi wireless quality analysis. In particular, Nishikawa gained practical experience from working for three years in a team that was involved in wireless communication itself. Our team handles the entire network system, but it is not the case that we have a deep understanding of all of the principles and mechanisms behind wireless communication. To that extent, Nishikawa has the aptitude to decipher and understand not only the system logs but everything including the physical phenomena behind radio waves. Perhaps it can be said that this collection of skills crossing the boundaries of specialized fields became the soil in which this technology took root.
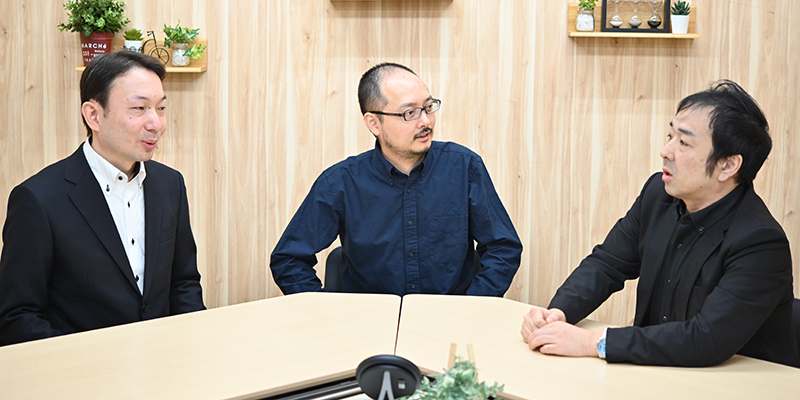
-- What are the future prospects for this technology?
Iwai: We expect that the primary usage scenarios will include operation at plants, construction sites, and warehouses. In particular, we have received many inquiries and consultations from customers in conjunction with robot automation, and many projects are actively under consideration.
Takahashi: We are already working on a demonstration verification internally, and verification is currently underway at a group company plant. In addition, we are also conducting verification using the living lab methodology in our offices at the Tamagawa Plant, and we hope to engage in co-creation with our customers and partners going forward.
Nishikawa: Furthermore, in the current phase this technology notifies a person about the root cause, and the person solves the problem. However, we hope to automate everything including the solution in the future. We plan to continue proactively advancing our research while focusing on automatic optimization solutions which automatically solve everything from analysis to control in conjunction with applications.
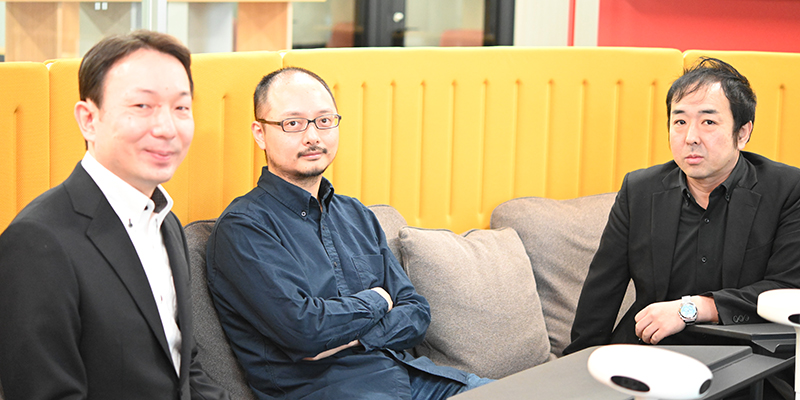

The learning-based wireless quality analysis technology is able to visualize the network communication conditions in real time, identify the root cause in the event of communication performance degradation, and rapidly present the information to the user. While there are prior technologies for the visualization of wireless quality, this technology is novel for its ability to achieve monitoring at minimal cost through the utilization of overall modeling and AI. Furthermore, when it comes to the root cause of communication performance degradation, the use of simulation and machine learning makes it possible to identify the root cause with high accuracy at low cost using only the variability of commonly available radio waves.
- ※The information posted on this page is the information at the time of publication.