Global Site
Breadcrumb navigation
Supporting the Rescheduling Process for Recovering Train Timetables with AI
Operation Optimization Technology
Featured Technologies June 21, 2023
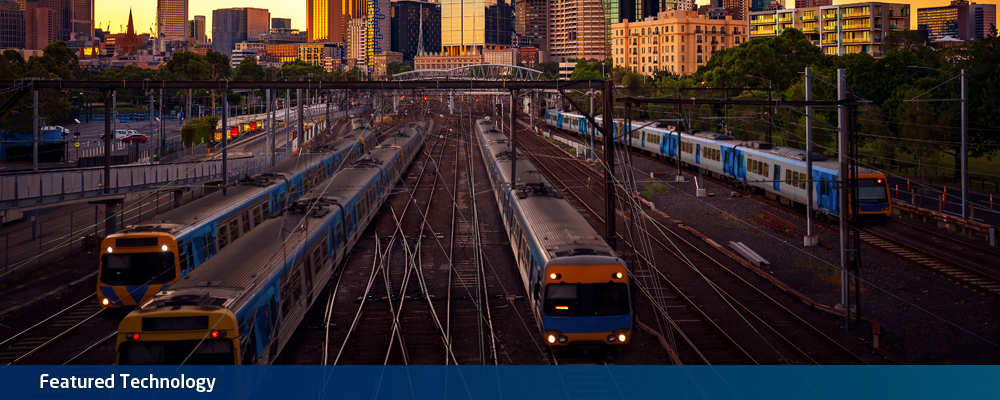
Disasters or accidents may cause trains to be suspended or delayed. In such cases, train operations should be restored to regular schedules through shuttle services, time adjustments, and other changes to the original timetables. These changes, known as ‘operation rescheduling,’ require considerable experience and expertise. In many cases, they require a rapid response, which places a significant burden on staff. Recently, NEC developed an operation optimization technology to support these operation rescheduling tasks using AI. We interviewed a researcher about the details of this technology.
Railway companies place high expectations on the operation rescheduling support system
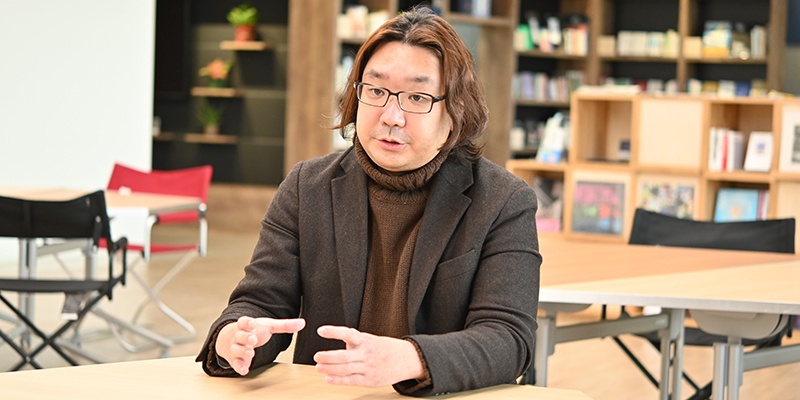
Principal Researcher
Shumpei Kubosawa
― What kind of technology is the operation optimization technology?
This technology can help develop a recovery plan when rail operations are disrupted by a disaster, accident, or other problem. Using AI, it can create and propose a recovery plan in a matter of minutes.
In recent years, the elements involved in train scheduling, such as four-track railroads and mutual through services, have become increasingly complex. Rescheduling operations to restore the original schedule (timetable) is still performed by experienced controllers. This work requires a high level of decision-making based on extensive knowledge and experience, but it places a significant burden on the workers to respond quickly to sudden events. Therefore, for more than 50 years, railway companies have played a central role in advancing studies of systems aiming at automating and streamlining this work. With the rapid decline of the labor force and other changes in social conditions, this technology can finally realize such requirements.
The most significant advantage of this technology is that the command staff can see the entire restoration plan. When dealing with problems by humans, they would tend to focus on only a part of trains and sections. However, this AI technology can simulate the results from the next move across the total line to show how it will change. This kind of overall prediction is simply tricky for inexperienced human operators. It also provides immediacy, so it can respond to ever-changing conditions on-site and always provide the latest overview.
Additionally, it can be used as a reference to help human command staff decide whether to take a specific modification by presenting a complete picture. So, it can support work at the site as an AI equipped with interpretability, which shows the basis of decisions.
We conducted a joint evaluation of this technology with the Odakyu Electric Railway Company to check its effectiveness in a desktop verification based on Odakyu's railway lines.
Presenting the appropriate measures through reinforcement learning
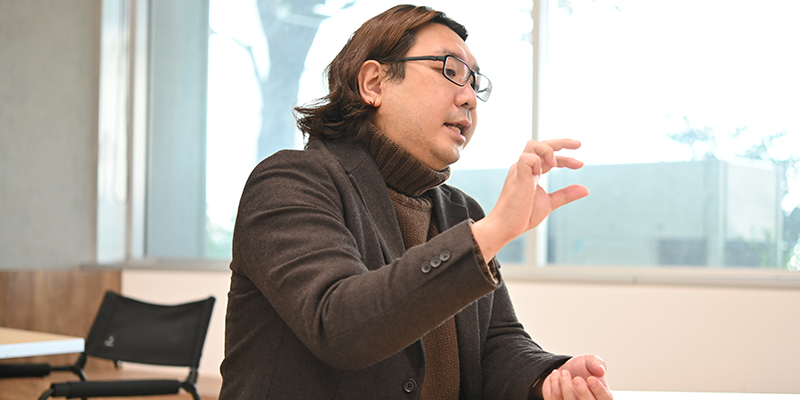
― What mechanism is used to realize this technology?
It utilizes deep reinforcement learning. Creating a restoration timetable is an optimization problem that combines many elements in a complex way. Is shuttling trains back and forth is effective? If so, what sections shall be chosen? Should the trains be put in the garage? What shall be informed to the train crews? How many minutes should the trains stop at each station? The explosive increase in the number of combinations across many variables made it impossible to calculate, which is why the automation of operation rescheduling could only be realized until now.
With this new technology, we create our own simulator to reproduce the railway operation with high accuracy and use it to perform reinforcement learning. Reinforcement learning is a method of machine learning that uses trial and error to train the AI. After setting evaluation criteria (reward function) for the AI, trial and error is used to make it more intelligent. For example, if train bunching (Note 1) occurs as a result of turning back at a certain station at a certain hour and minute, the AI learns that this is an error, and no longer selects it as a measure in a similar situation. Conversely, if train bunching does not occur as a result of turning back at a different station, that result is considered as a success and is more likely to be selected as an action.
In this way, the AI gradually achieves high performance by taking various actions in the simulator and repeatedly evaluating the results. In fact, it is a complex optimization problem that not only avoids train bunching, but also has to satisfy multiple objectives simultaneously, including restoring the original timetable and keeping the congestion rate below a certain number. In addition, because there are also site rules and tacit knowledge, it is designed to incorporate these factors one by one, while listening to customer needs.
Because we train the AI intelligently in advance through reinforcement learning, it is able to present a solution in a matter of minutes when an operation requires rescheduling. And because the AI is trained in a simulator, we can even reproduce events that it has never experienced.
- Note 1:condition in which the distance between trains narrows and train operations are delayed
Building a digital twin for control
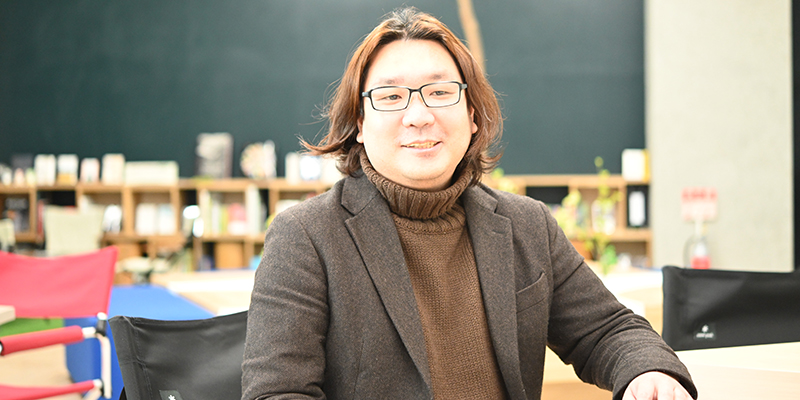
― Tell us about the future prospects for this technology.
First, our immediate goal is to continue the demonstration with the Odakyu Electric Railway Company. The Odakyu Odawara Line has four tracks and nearly 50 train stations between the Shinjuku and Odawara stations. An extremely large number of trains run on this line every day. Demonstrating the effectiveness of this technology on one of the Japan's largest railway lines in this way is significant.
We also anticipate a future where it will be connected to the railway operations management system. When this is achieved, we can realize elaborate simulations that link in real time to the exact train positions. Our goal is not to effectively operate the AI in the simulator but to improve the real situation. To do this, we must be able to accurately sense the current state. This is because a delay occurs when applying feedback to reality in simulation results based on information that is one kilometer off from the position of the trains that are actually operating on the line.
Taking this idea further, it should be possible to build a digital twin that represents the state of the train line in real-time. The digital twin would simulate the line in the digital world, predict the future, and provide feedback to the real world. In a future world where this is achieved, it may possible to implement semi-automation or automation of control.
In addition, we believe that the reinforcement learning utilized in simulators such as this one can be applied to more than just railways. We hope to pursue further research while also keeping an eye on horizontal expansion into other areas.

This technology uses a deep reinforcement learning approach to successfully achieve complex railway operation rescheduling, which was previously intractable due to combinatorial explosion. It is unparalleled in the world for its ability to handle operation rescheduling on large-scale railway lines. Further development is underway to successfully link NEC’s originally developed railway AI simulator with on-site expertise and deep learning.
- ※The information posted on this page is the information at the time of publication.