Global Site
Breadcrumb navigation
Estimating swelling and body weight from faces captured in images
Technology for estimating edema from facial images
Featured Technologies February 10, 2023
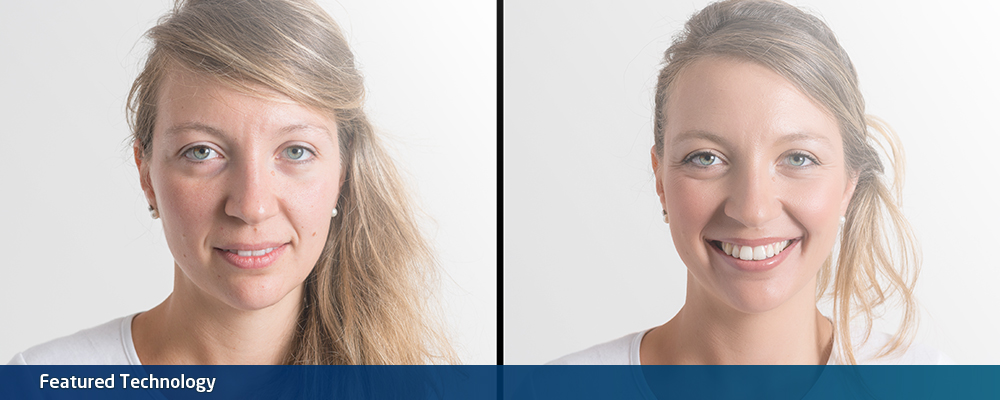
Recently, as face recognition technology has gradually expanded into areas such as payment systems and entry and exit control, NEC has developed technology for obtaining health information from faces based on such face detection and face recognition technology. This technology, which estimates the degree of swelling (body weight) from facial images, is expected to be used in self-care for dialysis patients. We spoke with the researchers about the details of this technology and its objectives.
Obtaining health information from facial images for use in online medical care
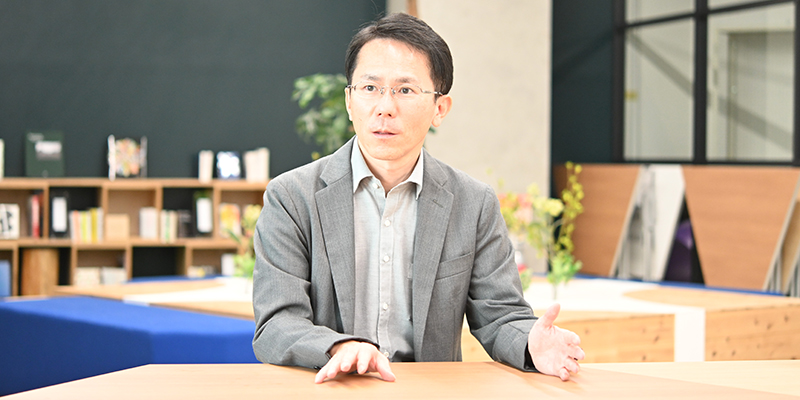
Principal Researcher
Yoshifumi Onishi
― What kind of technology is involved in estimating edema from facial images?
Onishi: We are using technology that can estimate the degree of edema (swelling) by analyzing facial photos taken with ordinary cameras such as those on tablets and smartphones. For a long time, NEC has been promoting a project to apply our world No. 1 ranked (*1) face recognition technology in the medical and healthcare fields, and this technology was developed as part of that project. We believe that there is great potential in obtaining health information from facial images, particularly since online medical care has been expanding in recent years.
We are conducting the research in collaboration with a medical doctor at the University of Tsukuba, who advised us that edema would be easy to assess based on information obtained from the face. For this reason, we decided to focus on edema first.
Akamatsu: Edema may occur due to various organ disorders such as kidney, heart, or liver disease. In particular, kidney patients who receive dialysis treatment need to be aware of the amount of fluid that accumulates in the body, and pay careful attention to the amount of fluid that they regularly consume. This new technology makes it possible to estimate the patient's body weight before and after dialysis, based on facial images. For dialysis patients, body weight is a direct reflection of the amount of fluid in the body, which makes it an important indicator for fluid consumption. Our technology makes it easy for patients to determine their body weight and estimate how much fluid they should drink when, for example, they are eating out at a restaurant, simply by taking a picture of their face with their smartphone.
Onishi: There are also many patients who are elderly or in wheelchairs. In many cases, it is not easy to have the patient get on a scale. Our technology will improve convenience in that regard once it is operational.
- *1:Ranked No. 1 in benchmark tests conducted by U.S. National Institute of Standards and Technology (NIST)
Achieving high-accuracy estimation with pre-training and personalized AI models
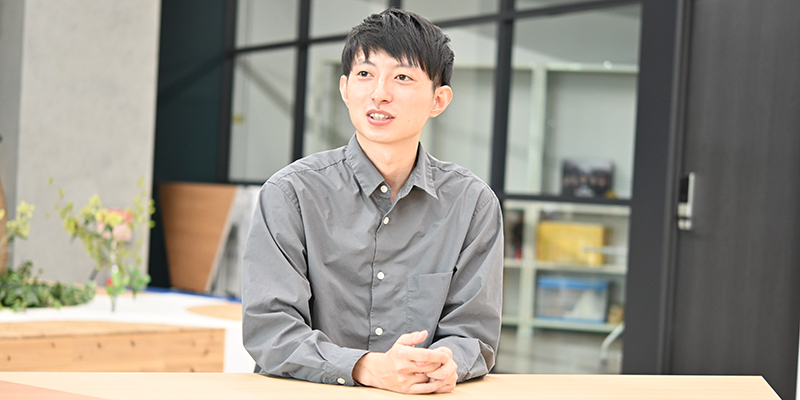
Special Researcher
Yusuke Akamatsu
― Specifically, what kinds of technologies are used?
Akamatsu: First, AI model is trained with data from multiple patients, and then "transfer learning" is performed to tailor the model to each individual. We initially took a general deep learning approach, and tried to train the AI with data from multiple people. However, this did not function well, as the accuracy rate was only 60% when pre- and post-dialysis classification was adapted to new patients. This is because each patient's face is completely different, and the physical appearance of edema varies widely. For example, since edema may affect the eyes of one person, or the nose of another, the appearance cannot be generalized. We thought that the only way to address this was to create personalized AI models, which led us to our current approach of performing transfer learning for each individual after pre-training of data from multiple people. The idea is to first perform pre-training to develop a basic model of what edema is, and then create unique AI models that are tailored to each individual's edema symptoms.
Onishi: As I mentioned earlier, the reason we focused on edema is that a medical doctor advised us that edema would be easy to recognize in faces, so we began our research with the assumption that a doctor would be able to interpret the images. However, when we actually started looking at images, we found that there were minute differences at a level that we absolutely could not comprehend. This is not a straightforward task, even for AI, and Akamatsu took the lead in developing the technology.
Akamatsu: The most significant point we devised is the pre-training algorithm. We are conducting what is called "contrastive learning," using two labels of "before/after dialysis" and "body weight." The model is designed on the assumption that if the patient's conditions and body weights before and after dialysis are close, the degree of edema is similar. We were able to achieve practical accuracy through training of pre- and post-dialysis information, as well as information related to the amount of difference between body weights, such as degrees of edema that are closer together when the body weights are 60 kg and 58 kg, and further apart when the body weights are 60 kg and 50 kg.
A research paper on this technology has been submitted to and accepted by the IEEE Journal of Biomedical and Health Informatics, which is one of the most prestigious journals in the field of medical and healthcare AI. (*2)
- *2:Y. Akamatsu, Y. Onishi, H. Imaoka, J. Kameyama, and H. Tsurushima, "Edema Estimation from Facial Images Taken Before and After Dialysis via Contrastive Multi-Patient Pre-Training," IEEE Journal of Biomedical and Health Informatics (J-BHI), 2022 (accepted for publication).
URL:https://ieeexplore.ieee.org/document/9976202
Verifying technology that obtains heart rate from facial videos
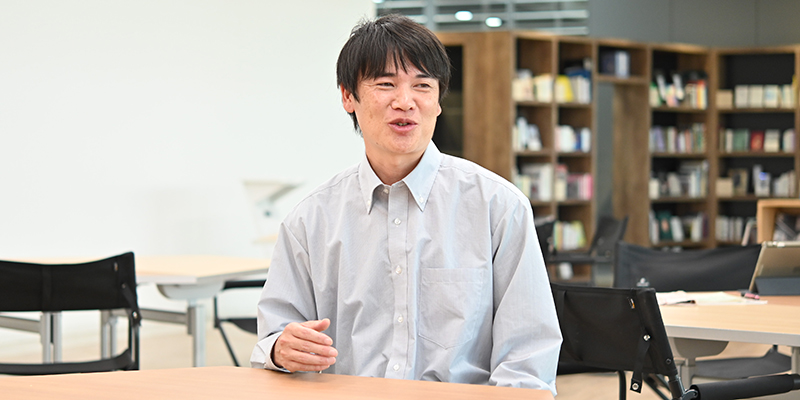
Senior Principal Researcher
Yuji Iwadate
― Does this technology have further potential, and what are the future goals?
Onishi: Our technology is still in the research phase, so I think the discussions about full-scale application will take place as we move forward. The patients and hospital doctors we spoke with in the course of our research were interested in the future potential of this technology. We also received positive responses such as requests for feedback on the types of use cases the technology will lead to. Since the types of applications this technology will bring about will depend on the discussions we have going forward, we would like to talk with people in a variety of fields and expand the range of possible applications.
Iwadate: Our technology is part of a project aimed at obtaining medical and healthcare-related information from a subject's face or external appearance. We hope to continue our research on obtaining health-related information from images and videos. For example, NEC is currently developing technology to estimate heart rate and SpO2 (oxygen saturation) from facial videos (*3). By combining our approach with such technologies, we will be able to expand the possibilities for online medical care and self-medication. Our group will continue to make use of NEC's biometric authentication technologies and pursue research aimed at applications in such medical areas.
- *3:Y. Akamatsu, Y. Onishi, and H. Imaoka, “Heart Rate and Oxygen Saturation Estimation from Facial Video with Multimodal Physiological Data Generation,” in IEEE International Conference on Acoustics, Speech and Signal Processing (ICASSP), pp. 1111–1115, 2022.
URL:https://ieeexplore.ieee.org/abstract/document/9747109
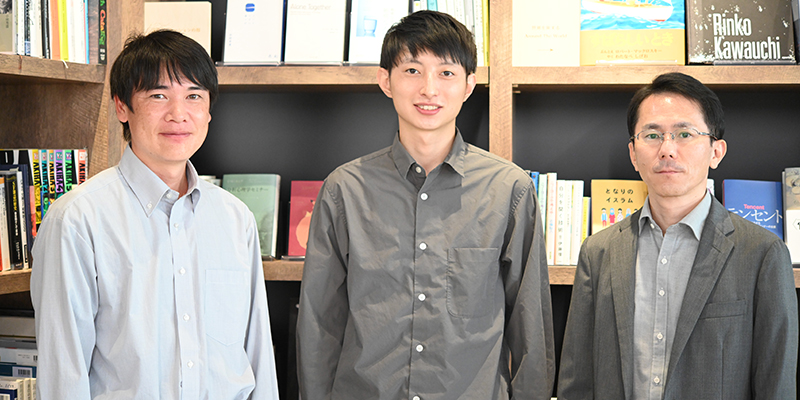

This technology originated from a project aimed at using NEC's face recognition technology, which boasts the world's No. 1 recognition accuracy, in the medical and healthcare areas. The technological research is being conducted with NEC's unique approach to obtaining health information from facial images and videos.
To estimate edema, pre-training is performed using the data of multiple patients, and then transfer learning is performed to build a model for each individual patient. Pre-training is an essential step because a high volume of data cannot be obtained from an individual patient. In addition, the physical appearance of edema varies for each individual, so it is not possible to capture a full understanding of edema through ordinary learning. It was therefore considered essential to create individual models, which led to this method. For the pre-training, a unique algorithm linking pre- and post-dialysis timing to body weight was developed. The achievement of a learning method that uses two labels has made it possible to perform high-accuracy estimation.
- ※The information posted on this page is the information at the time of publication.