Global Site
Breadcrumb navigation
Neuroscience x Deep Learning
A Revolutionary Technology Which Promises the Optimization of Speed and Accuracy
Featured Technologies May 6, 2021
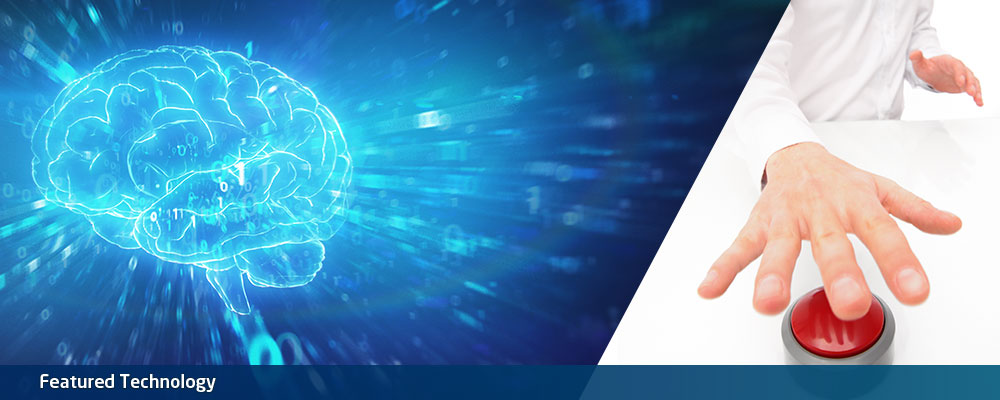
NEC announced "SPRT-based algorithm that Treat As Nth-Order Markov series: SPRT-TANDEM"(*1), a new and revolutionary technology that can be utilized in face recognition and other applications. We spoke with NEC Fellow Hitoshi Imaoka, who has led the development of face recognition for many years, and Researcher Akinori Ebihara about this technology (algorithm) developed based on hints from neuroscience.
- *1An algorithm based on a sequential probability ratio test which treats a data series as an Nth-order Markov process.
Hitoshi Imaoka
NEC continues to rank number one in the world in third-party benchmark tests when it comes to the accuracy of its fingerprint recognition, face recognition, and iris recognition. Imaoka is the leading figure overseeing all of NEC's research on biometric authentication technologies and leading the company to be ranked number one in the world for face recognition five times. Appointed as the youngest NEC Fellow in the company's history.
Biometrics Research Laboratories
Assistant Manager
Akinori F. Ebihara
Studied neuroscience at Rockefeller University in the United States where he earned a PhD in biology. In 2020, he won the

Achieving a decision speed which is three to twenty times faster than conventional methods while maintaining accuracy
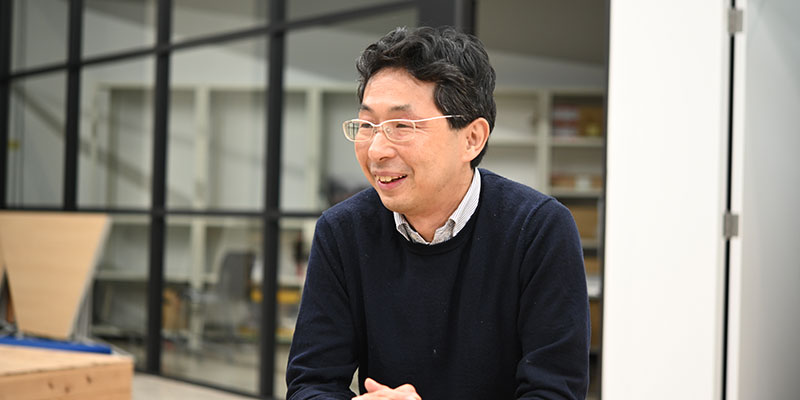
Hitoshi Imaoka
― What can you tell us about this newly released algorithm (SPRT-based algorithm that Treat As Nth-Order Markov series: SPRT-TANDEM) (*1)?
Imaoka: It is a technology which can increase the decision speed and decision accuracy for various types of sequential data. It is a technology which can be applied to anything if you are using chronological and other sequential data, but first let us use face recognition to explain it in an easy-to-understand manner.
Ebihara: Imagine a scenario in which a face recognition device is installed in front of an entry gate. In this case, you need to recognize a person's face while they are walking toward the device as quickly and accurately as possible to open and close the gate. In conventional face recognition, it was common to record a fixed interval with a camera and comprehensively interpret a fixed number of frames to recognize a face. For example, if 30 frames were specified, it would analyze 30 images to determine the recognition results. However, even in the case of a simple decision which immediately recognizes a face in one of the earlier frames, you would have to wait for all 30 frames to be analyzed, which would take too much time. Conversely, if it proved difficult to make a decision within 30 frames, there was a chance that a decision error would occur.
The recently developed algorithm is a technology which sequentially determines the recognition results. You could even go so far as to say that it is a technology that can instantly complete the recognition task as long as you ensure a certain level of accuracy in the first frame. Vice versa, in the case where a consistent level of accuracy cannot be maintained, it will continue acquiring and examining more frames, so it can ensure a sufficient level of accuracy. We have confirmed in experiments as well that this technology can achieve a recognition speed which is three to twenty times faster while maintaining the same accuracy as conventional methods.
To put it more simply, this technology works like a "push-button quiz." In a push-button quiz, you have to push the button as quickly as possible while answering correctly. For example, if the question, "What is the name of the author of 'The Narrow Road to the Deep North' who was active during the Edo Period?" was read aloud, how would we answer? Based solely on the information "active during the Edo Period," the accuracy of our answer would be low, so we cannot produce an answer just yet. However, once we hear the keywords, "The Narrow Road to the Deep North," the accuracy suddenly increases. Conversely, if the question was, "Who is the Edo Period person known for the 'The Narrow Road to the Deep North'?" then we would be able to immediately answer with high accuracy the moment that we heard the keywords "The Narrow Road to the Deep North." So you would think slowly for a difficult question and answer quickly for a simple one. In fact, evidence accumulation neurons act in the parietal lobe of the cerebrum when answering a push-button quiz type of question. Their response is weak and small for weak evidence such as "active during the Edo Period" and significant for strong evidence such as "The Narrow Road to the Deep North." Our decision making is thought to be mediated by these activities of the evidence accumulation neurons. This recently developed technology was inspired by this mechanism of the brain.
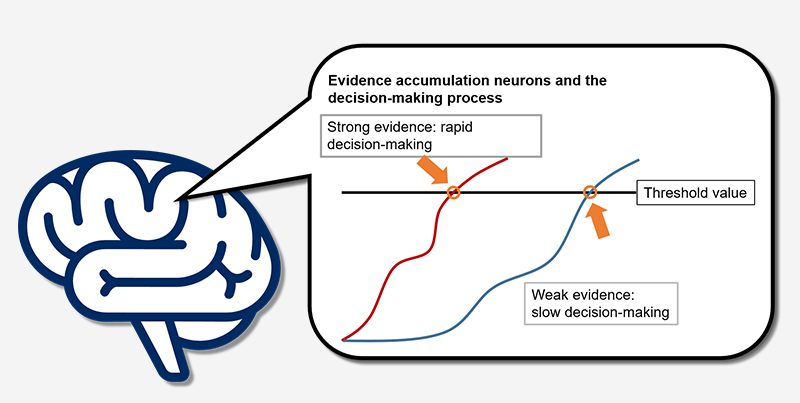
Imaoka: The reason why Dr. Ebihara knows so much about the brain is that he researched neuroscience at Rockefeller University, which is the same university that Hideyo Noguchi and Nobel Prize winner in Physiology or Medicine Yoshinori Ohsumi also attended. Dr. Ebihara earned a PhD in biology. He was investigating the brains of monkeys every day.
Ebihara: Yes, that is correct. I was researching how primate brains recognize faces. However, the brain mechanism mentioned just now was reported in prior research on monkey brains in 2002 (*2). Moreover, a paper in 2015 showed that the SPRT (Sequential Probability Ratio Test) can explain the decision-making mechanism in the brain (*3). Based on these reports, we examined the practical applications of SPRT as a technology for making faster and more accurate decisions in a technology such as face recognition.
- *2Jamie D. Roitman and Michael N. Shadlen
Response of Neurons in the Lateral Intraparietal Area during a Combined Visual Discrimination Reaction Time Task
Journal of Neuroscience 22 (21) , pp.9475-9489, November 1, 2002https://www.jneurosci.org/content/22/21/9475
- *3Shinichiro Kira, Tianming Yang, Michael N. Shadlen
A Neural Implementation of Wald’s Sequential Probability Ratio Test
Neuron Volume 85, ISSUE 4, P861-873, February 18, 2015https://www.cell.com/neuron/fulltext/S0896-6273(15)00008-2?_returnURL=https%3A%2F%2Flinkinghub.elsevier.com%2Fretrieve%2Fpii%2FS0896627315000082%3Fshowall%3Dtrue
Theoretically proven to optimize the speed and accuracy to a greater degree than any other method (*4)
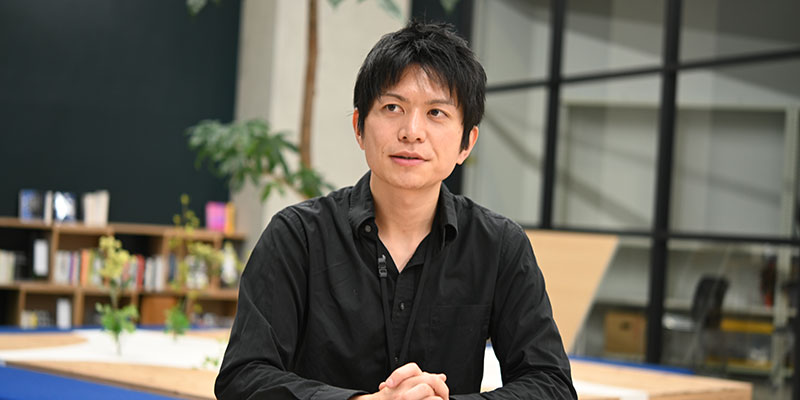
Assistant Manager
Akinori F. Ebihara
― What kind of technology is SPRT? How is the recently developed algorithm different?
Ebihara: SPRT is a method which was proposed by Abraham Wald, a mathematician who was active mainly in the 1940s, that utilizes values called "likelihood ratios." As the name indicates, they are values which represent the likelihood. You could also rephrase it as "confidence." Simply put, it is a method which sequentially updates the confidence to make a decision.
In addition, while speed and accuracy generally have a trade off relationship in a push-button quiz type of question, it has been theoretically proven that if you use SPRT, you can ensure the accuracy while optimizing the speed. In fact, there are currently many technologies which sequentially make decisions. However, it was already proven by Abraham Wald that you can make the fastest and most accurate decisions in any sequential hypothesis test if you can implement SPRT.
However, "the likelihood ratios are already known" and "the data is independent and identically distributed" are two conditions present in SPRT. While it can be utilized in limited scenarios where the likelihood ratios are known in advance, the conditions for use were too strict to broadly handle real-world problems, which made practical application difficult.
The algorithm that we recently developed made it possible to relax these conditions for real-world use. Applying deep learning made it possible to achieve high accuracy likelihood ratio inference, something which was impossible in Abraham Wald's day. In addition, by constructing a new neural network for likelihood ratio inference and designing a loss function to efficiently perform the likelihood ratio inference, we achieved a broad application of SPRT. This technology was created through interdisciplinary research spanning the three fields of neuroscience, early classification, and probability density ratio inference.
- *4Strictly optimal when the data is independent.
When the data is dependent, it becomes asymptotically optimal as the threshold value widens.
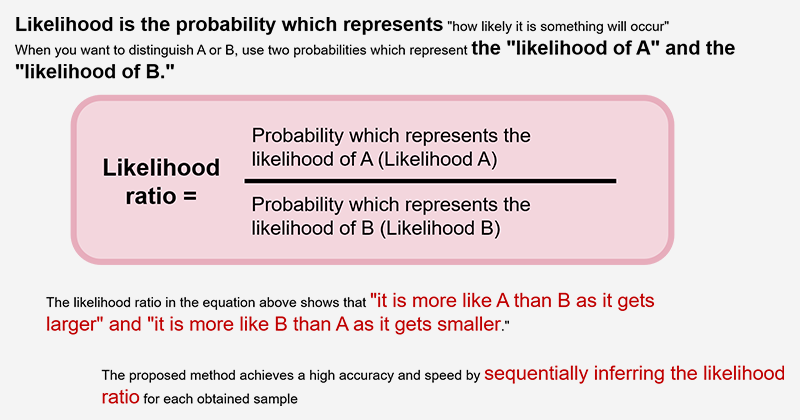
A revolutionary, new core technology that can be applied to any scenario
― In what ways can the recently developed algorithm be applied going forward?
Ebihara: As a matter of fact, the practical application of this technology is complete. NEC is planning to apply this technology to the facial recognition AI-engine "NeoFace." This research originally came into play during the development, but we were able to verify extremely good results when it was actually put into operation, so we thought that we should definitely write and present a paper about it. This paper was accepted by ICLR (International Conference on Learning Representations), the most authoritative conference on machine learning and artificial intelligence, and was also selected among the top 5% of research projects given an opportunity to be part of the Spotlight Presentations.
Imaoka: This technology was created in order to be applied to face recognition, but it is an extremely revolutionary technology that can be effectively applied to any sequential data. The application to face recognition is just one example. For example, in addition to speech recognition, cyber attack analysis, and cancer detection, the number of scenarios that it can be applied to will greatly expand going forward. We believe that it is an impactful invention which could become a new core technology for NEC.
I myself was involved in research concerning visual information processing in the brain when I first joined the company, so I always believed that experts in brain science were necessary for conducting biometric authentication and data science. I feel very inspired that a talented person such as Mr. Ebihara joined our team. Going forward, NEC will continue to proactively hire highly talented people from different fields and PhDs with cutting-edge knowledge to produce new types of innovation.
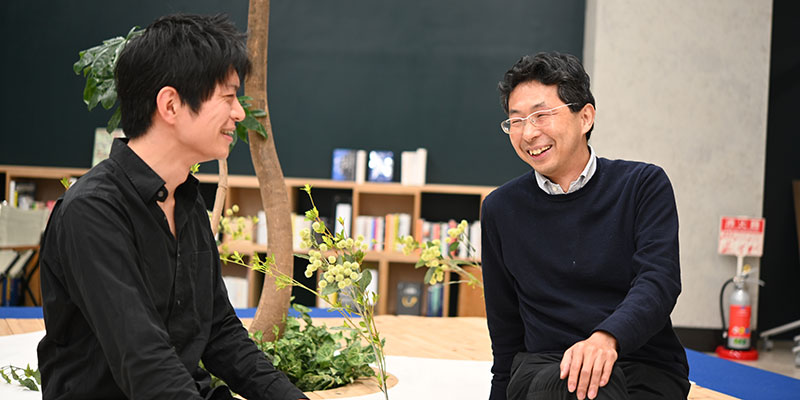
- ※The information posted on this page is the information at the time of publication.