Global Site
Breadcrumb navigation
Able to Search for Arbitrary Similar Human Behaviors On-Demand Action Detection Technology
Featured TechnologiesApril 5, 2021
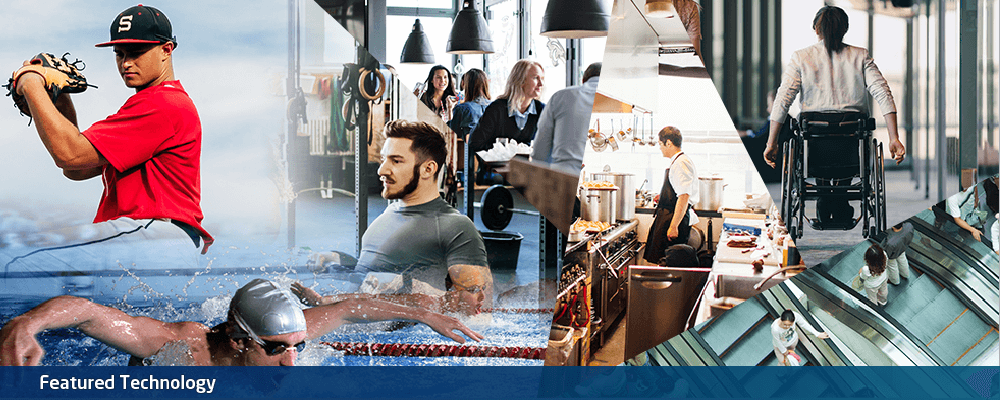
NEC announced its "On-Demand Action Detection Technology" employing the similarity search technology. We spoke in depth with two researchers about this technology which can immediately detect specific behaviors similar to a target behavior within a video from the live video and video archives.
Enable flexible and practical action detection by daring to abandon machine learning
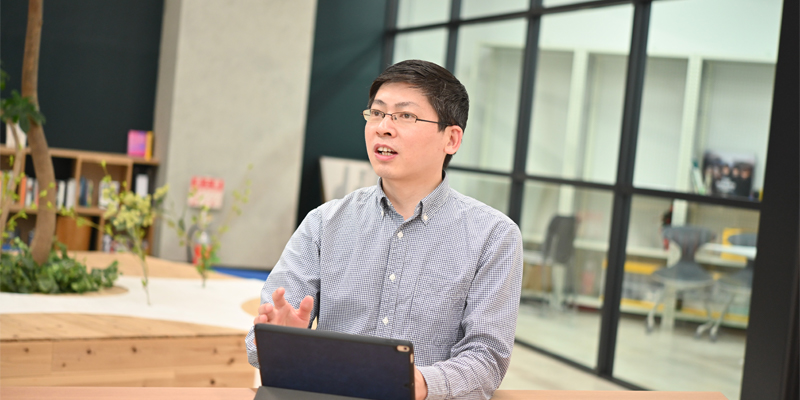
Principal Researcher
Jianquan Liu
― What kind of technology is the recently released "on-demand action detection technology?"
Liu: It is a unique technology developed by NEC which can detect arbitrary human behaviors in a video. It extracts approximately 18 different points from various joints including the shoulders, elbows, wrists, and knees, connects these points using lines and detects similar actions based on skeletal structure. For example, it can detect hand disinfection movements in a video for the purpose of infectious disease countermeasures. Naturally, it can detect actions in real time operations as well as action retrieval from video archives for any type of actions for statistical purposes as it is capable of handling both cases.
When it comes to traditional action detection technologies, video recognition using deep learning was the mainstream approach. However, our technology in this case draws a clear distinction with that trend. We used the "profiling across spatio-temporal data" that we previously developed as a foundation of this technology from the perspective of similarity search. By specifying the person as well as the start and the end frames of a target action in any scene of a video, the technology can detect all instances of similar action from the past to the present in a video. Because the action that you wish to search for can be handled in an on-demand manner for any type of movement within the video and it does not require machine learning, it is low cost and can be rapidly deployed and operated.
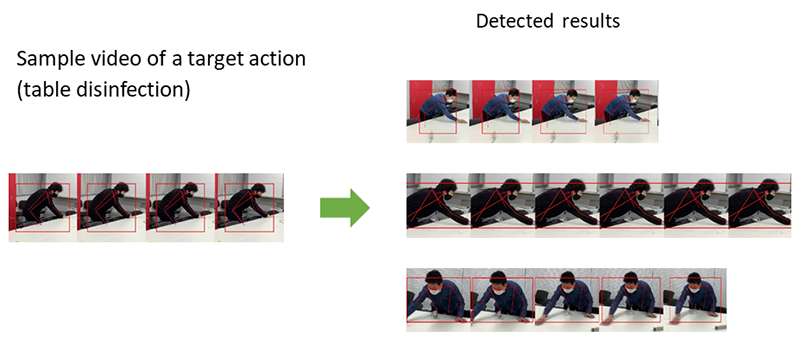
Yoshida: Since deep learning based approaches require a large volume of learning data to teach the system, they are incredibly costly. In addition, when you think about it realistically, it is essentially almost impossible to cover the learning cases to detect all arbitrary behaviors. Previously, I tried to use machine learning to detect the "unsteady walking" of people, but I realized that human behavior greatly exceeds the scope of what we can imagine. While one person may shake slowly while standing still, another may suddenly fall over without any warning. It is extremely difficult to cover and label a wide variety of unique individual actions. Moreover, even if you were able to label the actions up to a certain level, unknown patterns would likely be discovered once it was actually put into operation. With machine learning based technologies, it would require a significant cost and time to augment the data and retrain the system every time. For those reasons, we adopted the similarity search method for this latest technology. Because you can add detection targets on demand with similarity search, you can cover the detection targets during actual operation. In addition, because our technology does not specialize in a certain action such as "unsteady walking", but only searches the action itself from the video, it can flexibly adapt to the detection of any actions.
In truth, I was originally researching lithium-ion batteries, so I was not a researcher who specialized in machine learning. However, for that very reason, I continued to think that "machine learning was useful, but not all-powerful." I think that this way of thinking also appears in this latest technology approach.
Liu: That's right. Our team has been researching similarity search technologies which do not rely on machine learning. I think that the arrival of Mr. Yoshida from a completely different field generated an extremely positive chemical reaction.
High robustness that can adapt to any environment
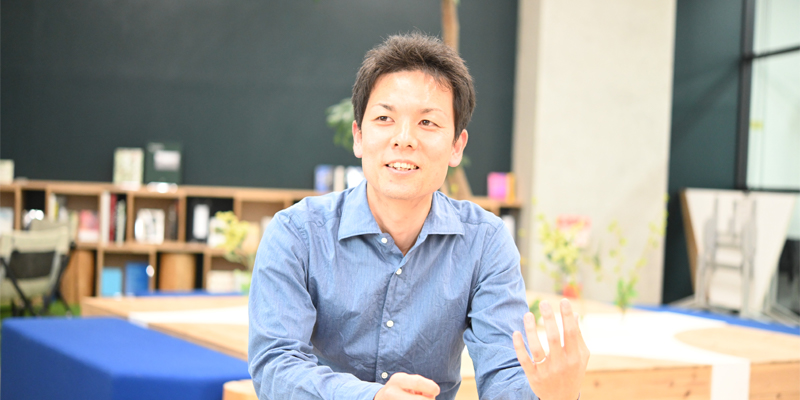
Senior Researcher
Noboru Yoshida
― Where were the issues and breakthroughs which occurred during the development of this action detection technology?
Yoshida: It was difficult to guarantee the robustness that enables the technology working stably in any environment. This is because in traditional video recognition based on deep learning, there was a problem in that the accuracy would drop due to the changes in the video brightness as well as people's clothing and belongings. Even if you train the system on a large volume of data, it was possible that you would be unable to obtain the desired accuracy when the video brightness changed at the application site or when people's clothing and belongings are different from the learning data.
For that very reason, the skeletal information was necessary to solve this problem. By focusing on a person's skeleton and posture, we removed visually unstable information such as the video brightness and people's clothing.
However, as a result of using skeletal information, "subject's orientation" became a problem. For example, even if a person was engaging in the same behavior, the appearance of their skeleton would significantly change depending on if they were directly facing the camera or facing it from the side. In response, the method that we came up with was to focus only on the "height." Instead of looking at the entire skeleton structure, we look only at the height of the points. While it is a simple concept, even if a person changes their orientation, the height of each specific point does not significantly fluctuate. By applying this approach, we solved the problem regardless of a subject's orientation.
The "positional relationship between the camera and the person" was also a huge problem in addition to their orientation. The distance between people's joints would change depending on whether they were filmed looking down from above or captured from a head-on position. Moreover, a person who was close to the camera would appear in different size from someone who was at a distance. We solved this problem using a method which estimates people's physical height. Based on a person's skeletal information, we estimate that person's height when standing upright on screen, or in other words their physical height, to accurately compensate for the inter-joint information. In this way, we became able to extract video information captured in three dimensions as two dimensional skeletal data regardless of the orientation.
In addition, it was also a problem of "speed/frame rate" for video processing. For example, even for the same hand raising motion, while one person may raise their hand slowly, another person may raise it quickly. While the person who moves slowly is recorded in many frames, the person who moves quickly is only recorded in a few frames. The same problem occurs even when the camera frame rate setting is different, but detecting similarity in these conditions becomes difficult. To deal with this issue, we came up with a method that extracts the key frames representing the change characteristics. Instead of looking at a series of movements, we focus only on the frames with significant changes such as the point where an arm starts to raise. As a result of doing so, we were able to create a technology which does not rely on the movement speed or camera frame rate.
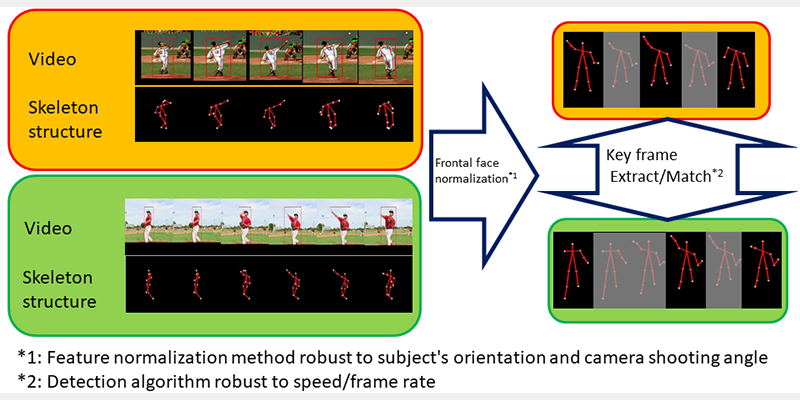
Liu: In addition, the person tracking technology developed at NEC Laboratories America is also a significant factor. By also adopting a technology that determines whether or not it is the same person in the video, the accuracy of this new technology dramatically increased. This new technology was created by the synergies through combining NEC's unique similar action detection technology developed by Mr. Yoshida with the “profiling across spatio-temporal data” technology and the tracking technology.
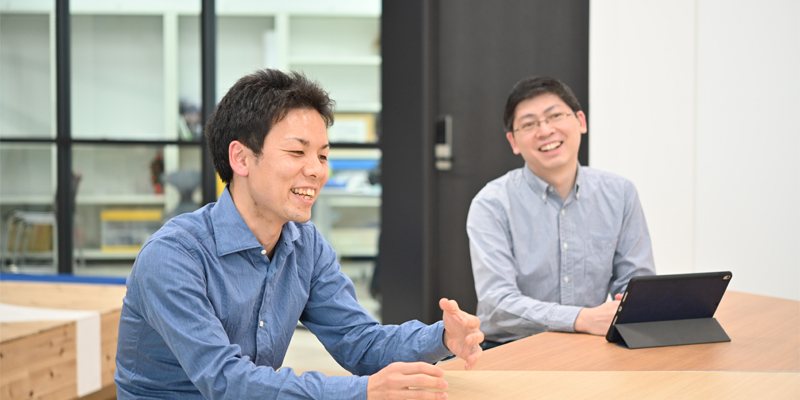
Capable of analyzing videos while protecting privacy
― In what ways can this new technology be applied?
Liu: I think that it can be applied in various different fields. Naturally, it can be applied to crime prevention and monitoring, and as well as applications to marketing. For example, if a person is raising their hands in front of a retail store shelf, you would understand that they are reaching for the shelf to pick up a product. This would indicate that they are interested in the product.
Yoshida: If you were to detect the number of times that they engaged in the act of extending their hands in front of the shelf, you would likely be able to extract data which represents how many people were interested in that product. Moreover, because such insights can be derived based only on the skeletal information, it can be operated in a way which takes privacy into consideration. If you only wish to capture the number of times that people reached for the shelves, it would be no problem to even delete the video in which the people appear.
Liu: As in this product interest example, I think that our video analysis technology is significant for the ability to show unknown and useful information which we would not notice just by looking at videos. We call these forms of value which are hidden within video, "insights," and going forward we have a vision that will converge not only people but people and items as well as the two axes of space and time as we hope to develop technologies that can present more valuable insights from videos.
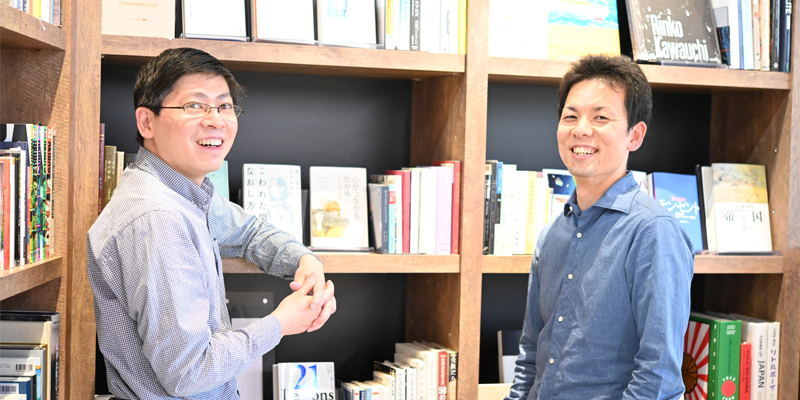
- ※The information posted on this page is the information at the time of publication.