Global Site
Breadcrumb navigation
Discovering the "why" from data Causal analysis technology
Featured TechnologiesOctober 6, 2020
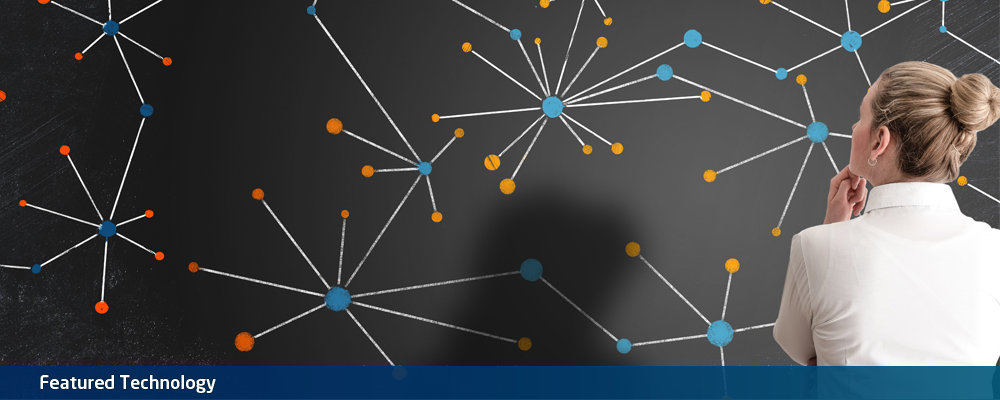
Causal analysis technology can estimate causal relationships among data. It is said that unlike general artificial intelligence technology that relies on the correlations between data, this technology's ability to discover the "why" is key to developing more advanced artificial intelligence. We spoke with an NEC researcher about the details of this technology.
Reaching new peaks in artificial intelligence with causal analysis technology
― Why is the analysis of causal relationships important in artificial intelligence technology?
First of all, the desire to find causal relationships among things is one of the most fundamental aspects of human intellectual curiosity. Long ago, people used to believe that evil spirits were the cause of infectious diseases, which led to many superstitions. Such superstitions drove people to perform rituals to expel evil spirits and cure illness. Eventually, with the development of science and technology, we discovered pathogens and developed the ability to prevent the spread of infectious diseases by researching and developing drugs and vaccines.
In addition, humans naturally begin to search for associations and causal relationships among things from the time they are infants. Only a few months old infants can learn that if they press a switch, lights is turned on and their mother will come when they cry.
Over the past 10 years, great progress has been made in artificial intelligence technology, as exemplified by deep learning. Artificial intelligence is rapidly gaining widespread adoption in the fields such as autonomous driving and machine translation. These developments are accelerating the move toward achieving systems that have human-like intelligence, with abilities such as causal inference.
General machine learning algorithms are primarily based on finding and utilizing the correlations between data. However, the correlations do not always imply causal. The crucial difference between correlation and causation is whether the cause has an actual effect on the result. To cite a common example, there is a correlation between ice cream sales and electricity usage, but no causation. The correlation is that ice cream sales and electricity usage both increase in summer. However, a reduction in ice cream sales alone does not cause a reduction in electricity usage. Similarly, a reduction in electricity usage alone does not affect ice cream sales, so there is no causal relationship.
The most effective way to determine whether two things have a causal relationship is through a random controlled trial (RCT). However, RCTs are often not feasibly practical due to their high cost and frequent ethical issues. Consequently, causal discovery and inference technology that discovers the causal relationships among things through observational data is a research field that is attracting more and more attention.
Judea Pearl, a Turing Award winner who is known as the father of the Bayesian network, successfully developed a causal inference framework that uses causal graphs to generate and extract causal relationships. Recently, the process of discovering causal relationships among data has been drawing widespread interest from academia to industry. Research themes have begun to shift away from basic theoretical research, toward applied research aimed at practical real-world use.
As Judea Pearl once said, causal analysis is a technique for mining the causes and effects (i.e. the "why") behind the data. Future developments of this technology will bring new advances and innovations in artificial intelligence and lead to the creation of new trends. In fact, new business opportunities that utilize causal analysis technology are already being created. For example, causal analysis technology is being considered for use in services that provide insights for market research, support the development of diagnostic and therapeutic strategies for health care professionals, and provide decision-making automation systems for retailers.
NEC's causal analysis for real-world problems
― Now that research in causal analysis technology is being conducted around the world, what are the unique characteristics of NEC's technology?
With conventional verification-type causal analysis technology, data analysts need to have specialized knowledge and experience in the target industry, and they are required to define the causal relationships by themselves. The framework of this technology is based on the verification of the causal relationships defined by the data analysts. Of course, since the work of formulating the causal hypotheses is performed by people, the analysis becomes very complicated if there are many variables.
However, real-world situations rarely involve a small number of variables. Scenarios encountered in market research, such as user behavior analysis, often involve dozens or more variables. It is not unusual for more than 100 variables to be involved. In other words, handling such data with verification-type causal analysis technology requires extremely complex work and enormous amounts of time.
NEC's causal analysis technology incorporates unique innovations to address these challenges. Nowadays, our technology is gaining widespread recognition from customers particularly in the field of market research, and the number of users is expanding. Here, I would like to briefly discuss four innovations that we have achieved.
Causal discovery technique that handles numerous variables
One of the innovations achieved by our technology is causal discovery technique that can handle large numbers of variables. With this method, the data analysts are not required to have specialized experience and knowledge of the target subject. The causal structure and relationships among variables can be found automatically from the observation data. By significantly improving the calculation speed with our unique high-speed search algorithm, it is now possible to handle data analysis with more than 100 variables. Results of data analysis experiments demonstrate that this method can reduce the analysis time by a factor of 50 or more, compared to conventional methods.
Causal analysis for mixed data of various types
In application scenarios such as customer questionnaire analysis and product sales promotion, various types of data are included, such as continuous data, discrete data, ordinal data, and categorical data. With conventional algorithms, it is common to consolidate such mixed data into a specific data type before processing. However, this method causes a substantial degradation in the accuracy of some data. To address this issue, we have developed our own causal inference method that demonstrates high accuracy even for mixed data.
Interactive embedding of expert knowledge
Nonetheless, errors and bias will inevitably occur in the causal structures and relationships that are automatically obtained from the observation data. However, it should be possible for experts to rely on their own experience and knowledge to correct any erroneous causal relationships. By achieving interactive embedding of expert knowledge, we have developed a method that significantly improves the accuracy of the causal structures and relationships. Previously verified causal relationships can be integrated easily, and the automatic model update functions enable the accuracy to be improved continuously. As mentioned earlier, the data analysts do not need to have specialized knowledge or experience. Accuracy can be improved by incorporating verified causal relationships and by enabling experts in the field to make simple corrections.
Integrated causal analysis platform
We have also taken great care to make it easier for users to use NEC's causal analysis technology. For example, we selected a SaaS software platform from the early stages of research and integrated the functions required for causal analysis, such as key factor analysis, causal graph complexity control, intervention effect simulation, and causal relationship visualization. This lowers the barriers for users to experience and use the technology, while also making it easy to get feedback from users.
Progress in demonstration and utilization in fields such as marketing
― What kind of use cases do you envision for actual applications?
Customers in a wide range of fields, such as marketing, retail, manufacturing, finance, insurance, medical, and nursing care, are currently showing a strong interest in this technology. In the field of market research, we have already collaborated with many customers and have been working on Proof of Concept(PoC) in actual business operations.
Use cases in marketing
For example, in marketing, the technology can determine the key factors that influence why users purchase a product. It can also help to evaluate and improve the sales activities of manufacturers, as well as to monitor the performance of sales activities.
The technology can also be applied in the analysis of customer satisfaction. For example, causal analysis technology can be utilized with the user survey data for a brand of shampoo to determine the factors that affect user satisfaction. By identifying specific points of improvement that can increase user satisfaction, the user experience can be enhanced continuously.
The technology can also be applied in brand analysis. For example, in the analysis of user survey data for a certain automobile manufacturer, a model was constructed for the causal relationships among a variety of factors and the brand. Among the various perceptions that affect the brand image, including emotional value, functional value, and social value, it was discovered that emotional value has the greatest influence. The use of these analysis to clarify the direction for strengthening the brand image has been one of our greatest achievements.
Use cases in the retail field
― Could you talk more about the demonstrations that are in progress, particularly in the market research and retail fields?
Sure. Retailers have the opportunity to leverage causal analysis technology in many processes, including marketing, pricing, and product displays. Furthermore, insights into sales data make it possible to develop data-driven marketing strategies based on customer data. One such application is attribution analysis, which evaluates the degree to which ads contribute to conversions. In one case, for example, we have constructed a model for the causal relationships among the customer purchasing behavior and the customer contact points inside the store (such as posters, leaflets, and sales staff) and outside the store (such as Internet, TV, and outdoor advertisements) based on the retail and marketing data of luxury milk in various stores, and are performing analysis to determine the attribution of each advertising contact point and the influence path.
In another case, when a model was constructed for the causal relationship between the price of individual furniture items and store sales based on the annual sales data of a global furniture sales company, specific furniture items were discovered that had a great influence on the overall store sales. The company now aims to increase overall store sales by improving the pricing strategies for key items discovered through such a process.
Use cases in business decision-making
― In what other fields is the technology being used?
The technology is also helping to support decision-making in management. Data is an important factor when making big business decisions. Causal analysis technology can provide a scientific basis of support for decision-making by identifying factors that influence judgment based on various data, as well as by quantifying the degree of influence.
In one case, for example, an analysis of a certain detergent product revealed that the cleaning ability was the most important indicator of purchase behavior. As a result, the company's new product development process has become more focused on continuously improving the cleaning ability, and their advertising also more strongly highlights the cleaning ability of their products. In another case involving a telecommunications carrier, the main factors affecting customer satisfaction were estimated based on customer behavior data and questionnaire data, and they were used to support the execution of strategies aimed at improving customer satisfaction. Extending this solution to larger-scale customer groups makes it possible to anticipate the improvement effects of various strategies on customer satisfaction, and to accurately identify the appropriate target customer segments.
Aiming to create a semi-open platform for causal analysis
― What are your future goals?
Up to now, we have collaborated with several major corporations, mainly in the marketing field, to perform proof of concept in a variety of business scenarios. The application effects and potential of causal analysis have earned high praise, and the future prospects for the technology are promising.
Going forward, we plan to provide a software development kit (SDK) for causal analysis in order to maintain the performance advantages of the core algorithms of causal inference, as well as to accelerate the spread of causal analysis technology into other fields. We are also considering establishing an open architecture for causal analysis.
In doing so, we aim to support mixed data structures that incorporate a greater diversity of data, including time-series data. We would like to improve decision-making optimization systems based on causal relationships, and realize the construction of technology that can support the cycle of analyzing data, deriving causal insights, and performing causal decision-making.
We also seek to develop and provide an SDK that includes the core functions of causal inference, with the goal of increasing its utilization and adoption in many fields such as manufacturing and medical care. We are aiming to smoothly incorporate NEC's causal analysis technology into the business processes for a wider variety of customers.
Furthermore, we would like to expand NEC's proprietary causal analysis platform to a semi-open platform. By making the platform compatible with open-source and third-party causal inference algorithms, we hope to develop an ecosystem for the research and utilization of causal analysis technology and accelerate its development and spread.