Global Site
Breadcrumb navigation
Rare Event Discovery Technology: Revealing all possible patterns and their probabilities by AI and simulation integration
Featured TechnologiesMarch 30, 2019
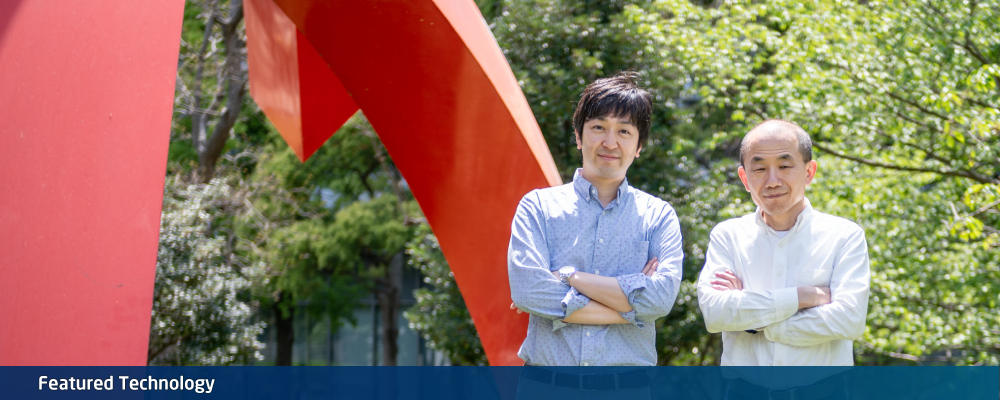
The rare event discovery technology integrates AI and simulation to reveal all possible patterns and their probabilities. We asked the two researchers in detail about the technology that was developed by NEC and AIST in collaboration.
Technology that drives AI even with zero training data
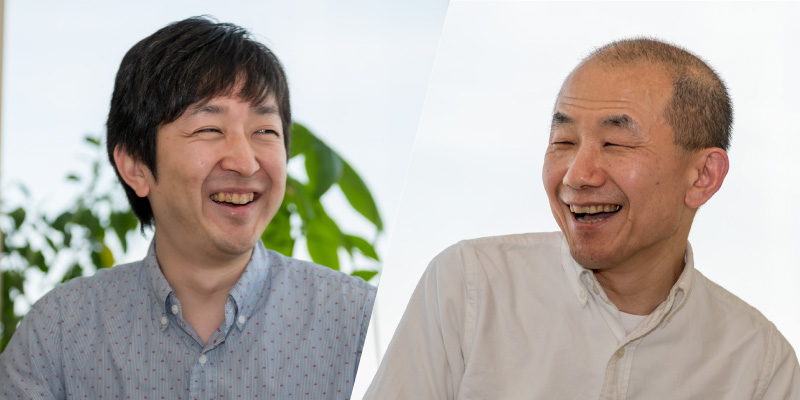
Assistant Manager,
NEC Data Science Research Laboratories
Project Manager,
NEC-AIST AI Cooperative Research Laboratory
Professor,
the Institute of Scientific and Industrial Research,
Osaka University
Leader,
NEC-AIST AI Cooperative Research Laboratory
― What is the research direction aimed for in NEC-AIST AI Cooperative Research Laboratory?
Washio: This research laboratory is the first industry-university joint research institute established by AIST (National Institute of Advanced Industrial Science and Technology). Projects are launched based on three advanced and practical research themes related to artificial intelligence (AI). We have been actively conducting research since 2016. The research achievement in one of the projects is the rare event discovery technology.
Kisamori: That’s right. The rare event discovery technology comes from the basic idea of searching and exploring all sorts of patterns and their possibilities by integrating simulation into AI. For example, we developed this technology assuming a use case in manufacturing process management as well as in machine design.
Washio: I think there isn’t any other research in the world that is based on such a concept. It is sure that there are many studies that take AI technologies into simulation to make simulation faster or more advanced. However, our technology does the opposite, which is using AI by integrating simulation into it. This makes it possible for AI to operate in environments where training data isn’t enough to apply conventional AI technologies.
One of the hot topics in AI research field is machine learning with a small amount of data. Typically machine learning technology rely on a massive amount of data to learn relationships and patterns, but the sufficient amount of data may not always be available in real business. For example, accidents or malfunctions in plants are usually rare, so collecting sufficient amount of data is difficult. Global B2C IT enterprises use mass information collected from individuals worldwide―however, I think that Japanese firms should not rely on “big data” in order to survive and thrive, instead they should focus on the technology that enables machine learning with small amount of data in B2B. Furthermore, AI that works even when there is absolutely no or zero data will be within our reach. The rare event discovery technology creates the base of the development of a practically useful AI where there is no data or where data is difficult to collect.
Successful revealing not only rare event patterns but also their probabilities
― Please explain the rare event discovery technology.
Kisamori: Briefly saying, the technology effectively explores all patterns by using a simulator. It can find patterns with their probabilities in short time without overlooking even if they are difficult for humans to find due to their rarity.
In fact, we already have a press release of a successful case with optical equipment design in May 2018 as our first research achievement. When designing optical instruments such as a telescope, you combine multiple different kinds of lenses to create the optimal performance balance―however, in very rare cases, you could suffer stray light, which degrades the performance of the instrument. This is a problem that occurs at a probability of around one in a hundred million. Conventionally, experienced specialists spent about a week for verification. Now with this technology, we can complete the verification in as short a time as a day, and find all of the conditions where the problem occurs.
This time, we enhanced the technology so that we can also derive the probability of any given occurrence. When you map out all rare events, the presented patterns can include those that are unlikely to occur in real life. This update enables the AI to learn the probability of each pattern while taking into account such circumstances.
The association of the highs and lows of risks with probability allows the AI to search and learn by focusing on high-risk, high-probability events, which realizes high efficiency. The search also covers areas with lower probabilities at the same time, which reduces the possibility of overlooking rare events. I believe that we struck a good balance between concentration and distribution in pattern search in terms of suitability for practical use.
This enhancement also showed successful results in experiments using a production process simulator provided by a steel manufacturer. When we showed the results to the experts who know the process characteristics very well, they saw the benefit of not only covering all expected patterns, but also discovering high-risk patterns that were difficult to find in a short time through conventional means.
Previously we used the technology to find out all possible risks in optical design. This time, we assumed application at a plant and confirmed the effectiveness of the technology under circumstances where evaluating probability is critical. As we are aiming at a technology that can be universally applied, it is significant for us to achieve this success.
Washio: It is very meaningful that we were not only able to find out rare cases, but also demonstrate that the probability of each pattern can be calculated. Because this lets us calculate risks. Knowing how much risk it takes for something to happen―this will be a vital indicator for us, humans, when making our final management decisions.
Furthermore, both in the cases of the optical instrument and plant, we started experiments of this technology in a “zero data” state, where no real data exists. I would like to emphasize that we have produced successful results based solely on the simulator provided by a company.
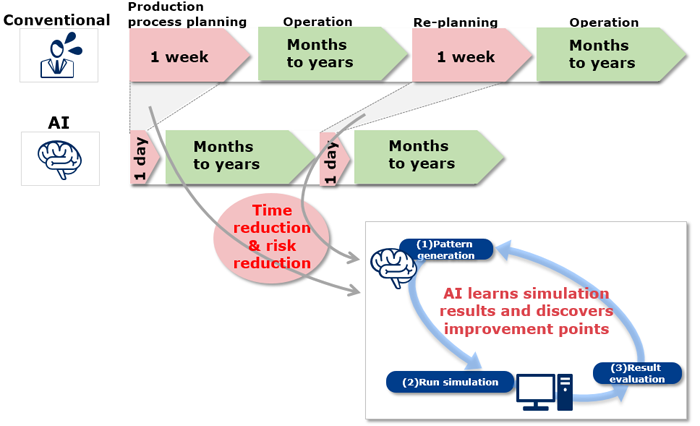
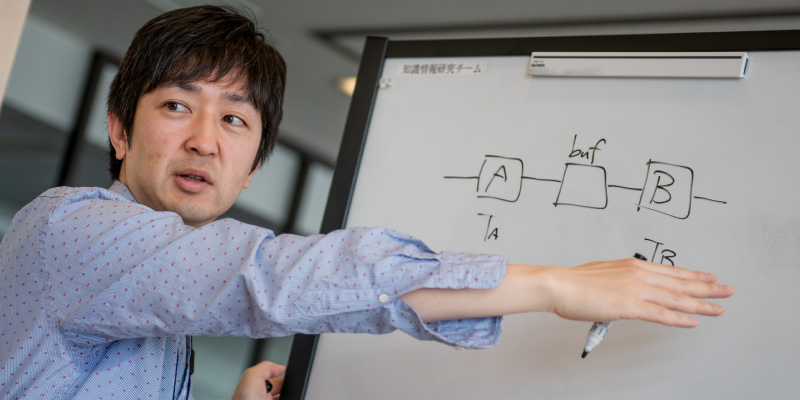
Aiming for a platform that supports any simulator
― What applications do you suppose for this technology?
Kisamori: First, we suppose simulation-based design and planning in manufacturing. For example, in the steel industry, the intermediate products accumulated between processes can overflow if the prior process is faster and the following process cannot catch up to it. Eventually, production will come to a stop. Particularly in steel manufacturing, this situation is critical because intermediate products kept in storage are heavy and large―carried by trucks and cranes.
This is one of the simplest examples―if you use this technology, you can speed up high-dimensional simulations that involve more complex production processes and operations. The AI will go over tens and hundreds of thousands of patterns and present highly probable risks beyond human expectation in short time.
Washio: Today, industries are seeing a transition from mass production of fewer items to smaller lots of numerous items, making the management of manufacturing processes and plans quite complicated. It is no longer a world where we can keep the plant moving at a certain production process, but rather, fluid production is in demand for each day. We need to constantly think about new developments. While past experiences may not be enough to drive production, the application of this technology can create social value.
Companies are increasingly being evaluated by their social responsibilities nowadays. Any problem in manufacturing can have significant liabilities for the company. Companies need to exert extra efforts to catch problems in all processes and resolve them one by one. We believe that the need for this technology is increasing in order to further ensure the reliability of design and production plan.
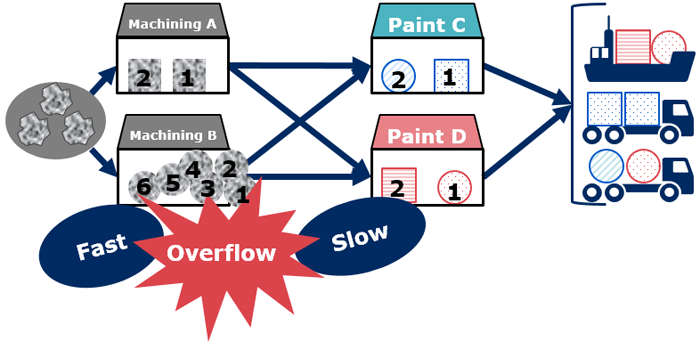
Kisamori: What we are ultimately aiming at is making this technology into a platform. Currently, we are focusing on manufacturing, but our final goal is to make a platform that can be utilized in any industry, for any application, and with any simulator by replacing simulators.
Washio: Yes. This technology should be applicable to process and design optimization as long as we can model the target. We hope to finalize this technology as a platform widely usable by many customers.
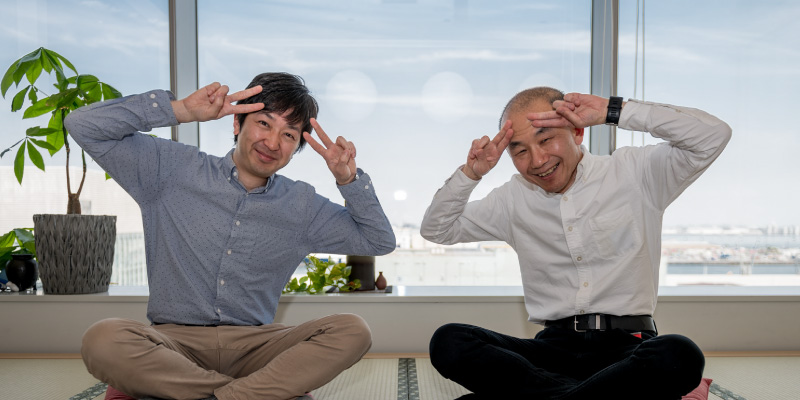
- ※The information posted on this page is the information at the time of publication.