Global Site
Breadcrumb navigation
Achieved real-time wireless communication of IoT - adaptive network control technology
Featured TechnologiesFebruary 21, 2019
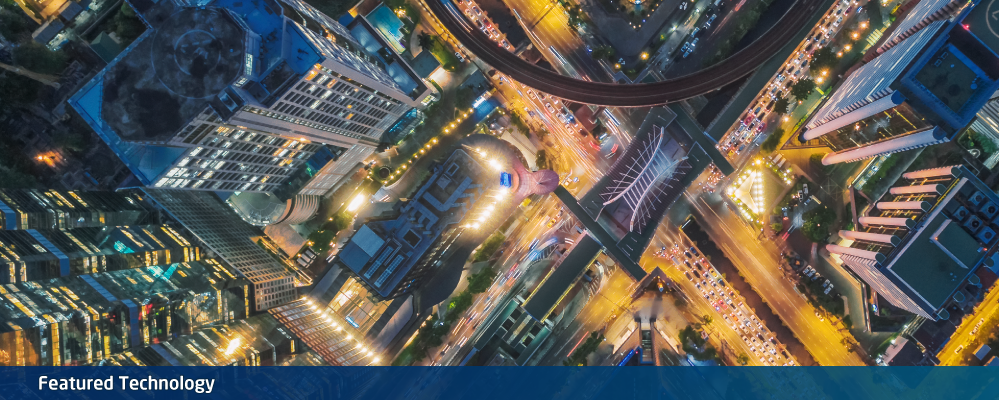
An adaptive network control technology that constructs a stable communication environment by determining the priority of the types and situations of IoT devices to be connected via wireless network and then allocating wireless bandwidths. We spoke to the developers about the details and possibilities of the technology.
Valuing communication delay deadlines and social security
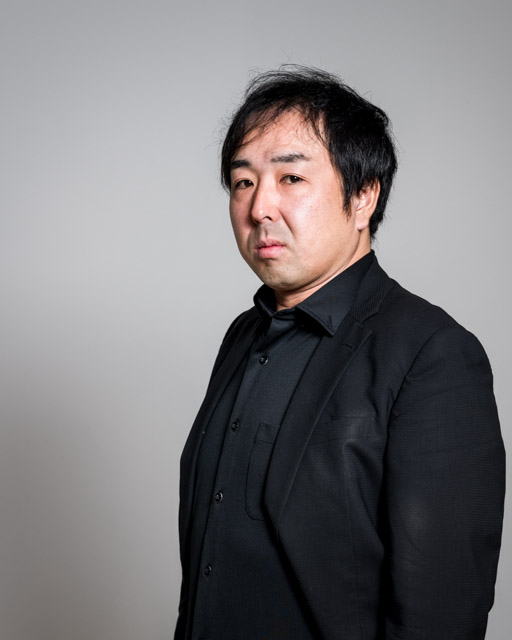
Principal Researcher
System Platform Research Laboratories
― What kind of technology is adaptive network control technology?
This technology determines the level of priority by grasping the types and situations of terminals to be connected to the same wireless network and then performs real-time distribution and control of the network’s wireless bandwidth.
Wireless communication uses shared radio waves, but the available bandwidth (wireless bandwidth) for wireless communication is limited. Therefore, if there are many terminals connected wirelessly, wireless bandwidth congestion will occur. With conventional technologies, the situation was controlled by allocating transmissions in the order of access following policies for fair allocation of wireless bandwidths. So, if people using the Internet feel their connection is slower than usual, this kind of queuing is the reason behind it.
However, this must be changed in a society where IoT and autonomous driving will be widely adopted. Take autonomous vehicles for example. At intersections, a large number of people with smart devices as well as vehicles that communicates with the network gather. If there is a great delay in communication between even a single vehicle and the wireless base station, there is a risk that the vehicle may collide with another vehicle.
In scenes such as this, the maximum communication speed, which had conventionally been sought after in terms of quality, is not relevant. What is more relevant is to be able to stably allocate proper communication speeds to all devices even if the maximum communication speed was slow in order to avoid accidents. So the idea is to meet the communication delay deadline and not ensure maximum speed. We have developed an adaptive network control technology that is capable of meeting the communication delay deadline by appropriately allocating transmissions to bandwidths based on the level of priority per terminal.
Actually, the idea itself had already existed from long ago. However, at this point, “prediction” was a problem that arose. To meet the deadline, at times, it is required to perform allocations to other high priority devices by lowering the priority of devices that are predicted not to have communication delays. Therefore, if the prediction of these delays could not be obtained precisely, there was a possibility that the all-around performance would become lower than that of conventional methods.
The main appeal of this technology is that it was able to achieve highly precise “predictive” capabilities. Other than estimating communication terminals through machine learning, it has been successful in building stable wireless communication through prediction and control by performing real-time analyses of the communication situation. The technology has earned a high reputation at international conferences and its research paper has received the Best Paper Award at the IEEE ComSoc International Communications Quality and Reliability Workshop (CQR) 2017. Also, it is scheduled to receive the Best Paper Award from the Technical Committee on Network Systems of The Institute of Electronics, Information and Communication Engineers (IEICE).
Assuming applications in mobility and surveillance cameras
― What kind of applications are you thinking about?
First, we are thinking about applying the technology in the mobility area for devices such as vehicles. According to the international standard for vehicle communication, a communication delay within 100 milliseconds and a success rate of at least 95% is set as the standard for safe operation (*1). However, during a simulation performed in February 2018, we had cleared the success rate of 95% for communication within 100 milliseconds (*2). This success rate is equivalent to approximately 5 times (*3) the success rate attained through conventional methods and is an innovative value even in global standards. Recently, we developed a prototype and managed to achieve successful verification results through tests on the actual device.
In relation to autonomous driving, we are also considering to apply the technology to transport robots (AGV) in factories and logistics. A crucial issue within the industry is how to raise the transportation efficiency of AGVs in facilities where several hundred machines are simultaneously in motion. In the sense that the network is controlled to enable the machines to efficiently move around without colliding in real-time, it is basically the same as autonomous driving, so we believe that the technology can be applied immediately.
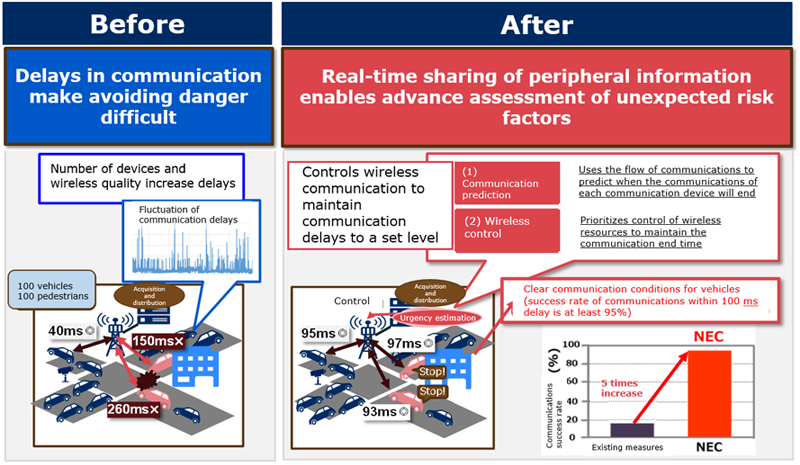
Furthermore, we are looking into applying the technology for surveillance cameras. Traffic in surveillance cameras fluctuates in data amount as time passes. In recent years, compression technology such as H.264 has evolved and since feeding differences frame-by-frame has become the mainstream method, the amount of traffic varies greatly depending on whether or not there are people present.
Observing this point and applying it to machine learning will enable us to understand the characteristic of traffic when there is a person present or not, or if there are several people present. Therefore, it is important to determine whether or not an incident is occurring rather than paying attention to the actual image. We believe that this technology would be capable of effectively using the network bandwidth by determining the priority of cameras at large facilities that perform security and surveillance with several cameras.
- *1An organization that examines the requirements and specifications of mobile networks:
3GPP (3rd Generation Partnership Project) is an organization that standardizes mobile networks such as the 3rd generation (3G), 4G, and 5G. A similar value concerning network requirements for vehicles was reported by the 5G Infrastructure Public Private Partnership, a European 5G related research promotion organization. - *2Communication success rate:
The percentage of cases in which data sent from the terminal per unit of time was received within 100 milliseconds. - *3Conventional ratio:
Proportional Fairness technique. A widely known technique for wireless resource allocation of the wireless base station. (The communication success rate of the communication delay within 100 milliseconds is 18%)
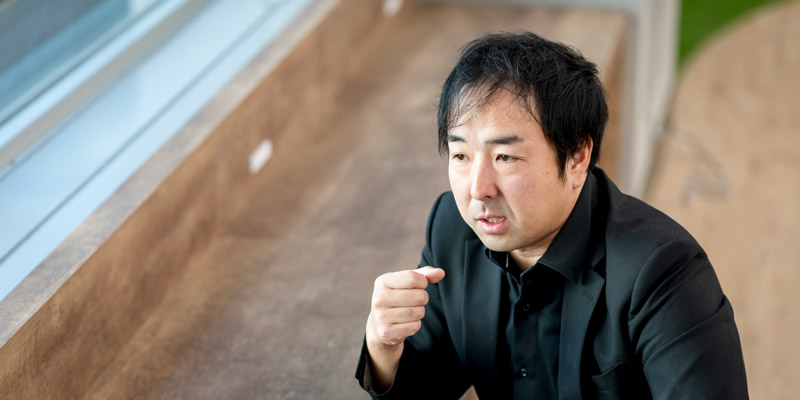
Anticipating smart city network construction
― Looking further ahead, what kind of development are you planning?
We are working on developing an autonomous learning system using reinforcement learning anticipating 5G. One theme of this technology was to enable it to optimally tune parameters by sufficiently adapting to changing environments. We look to continue improving its precision.
Also, though we’ve talked about various applications, recently we’ve come to believe that this technology will end up leading to a “smart city.” The use of the technology at intersections and factories are all important applications and what can be achieved when these technologies are combined is a network that supports our daily lives. We believe it is important to further evolve this technology in order to create a network for the entire city that supports the lives of all its residents.
To begin with, the IoT usually develops into a single solution by combining several services. We believe that advancing research while crossing over technical fields and business areas would lead creating new social values. Paying attention to only one technology will not lead to change.
In that sense, NEC’s comprehensive power is a potent trait. I believe this technology could not have been created relying solely on AI technologies and knowledge on communication. Concretely speaking, in the network communication field, time-series prediction used in machine learning doesn’t function well. In communication, since there are frequent occurrences of unevenness and loss in data, it is not possible to use all time-series data as they are. We were able to create this technology by integrating the know-how of our network researchers.
NEC boasts a wide business area including AI and communications as well as a wide range of customers. Another one of our strengths is our various research teams composed of experts in each field that are capable of collaborating with each other. Making use of the NEC’s comprehensive power, we aim to continue providing new solutions with the help of our customers.
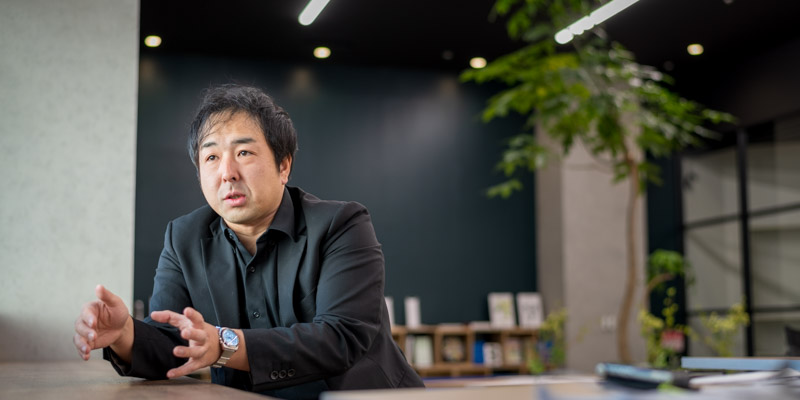
- ※The information posted on this page is the information at the time of publication.