Global Site
Breadcrumb navigation
Retrieval based time-series data analysis that enables instant detection of similarities with past data
Featured TechnologiesDecember 12, 2018
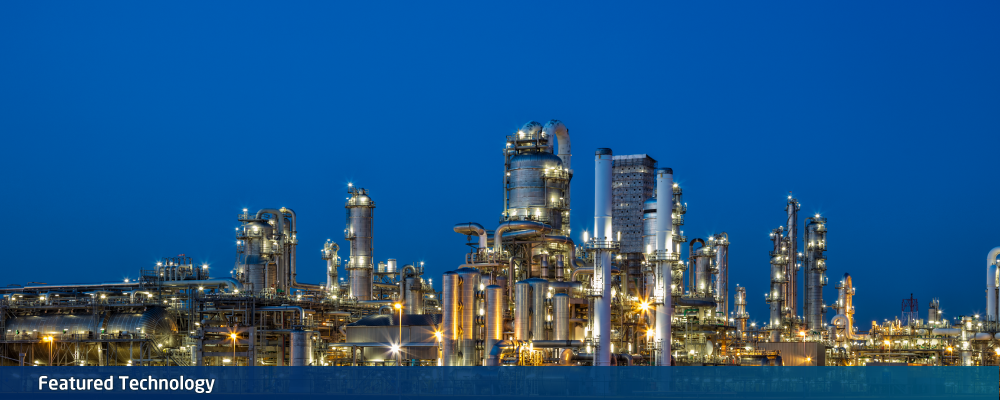
NEC Laboratories America is developing Retrieval based time-series data analysis technology. We asked the Director of the Secure System Research Laboratories, which is currently collaborating with NEC Laboratories America on applied research, about the details.
Searching for features in a large-scale system across time
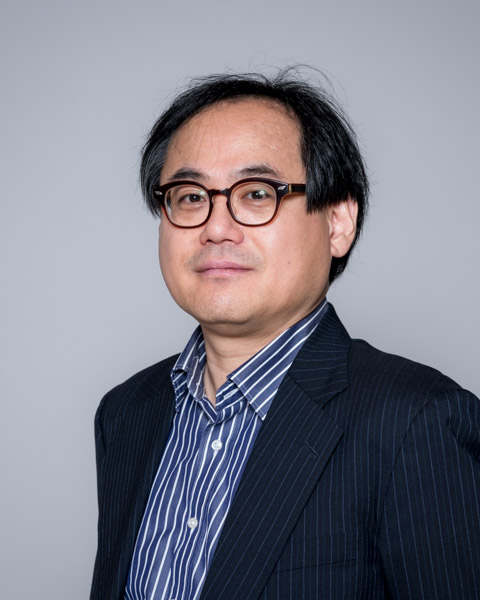
Senior Manager
Secure System Research Laboratories
― What kind of technology is the Retrieval based time-series data analysis?
It is a technology that enables instant matching of and finding similarities between present and past time-series data such as sensor values. With this technology, people will be able to quickly and accurately acknowledge that the current system situation is similar to something that has occurred in the past.
For example, megaplants, data centers, and social infrastructure facilities have thousands of sensors collecting time-series data to monitor the systems. If a change in sensor values can be instantly checked for similarity with a past condition in the system, then we can more accurately understand the state of the system. However, even if we try to simplify the matching of a few tens of seconds of recent time-series data with the enormous amount of amassed past data, the comparison of sensor values from several thousand sensors over a year needs a comparative computation of trillions of times―an immense volume of computation. So that is where deep learning comes in. Time-series data is learned via deep learning, and features are extracted from the data to be used for comparison. This way, the comparative computation can be compressed to one hundred-thousandth, which achieves high-speed searching. Also, the other significant characteristic of this technology is the ability to perform higher precision data matching as more data is accumulated over time.
The essence of this technology is to be able to extract features in a compact form by combining the features of temporal changes in sensor values and the features of the relationships among sensors. More specifically, the key point of this technology is that it can efficiently learn accumulated time-series data through deep learning and generate a feature extraction engine according to that system. This way of learning was presented at the 24th SIGKDD Conference on Knowledge Discovery and Data Mining, an international conference on data mining held in London in August 2018.
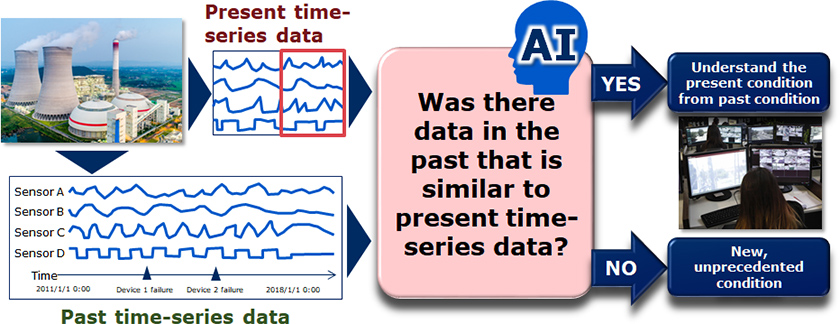
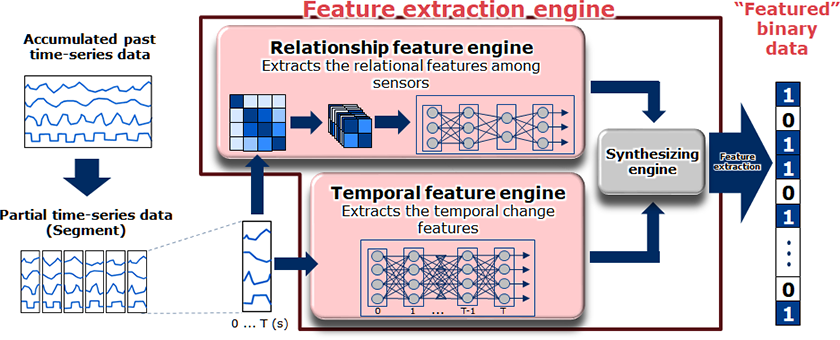
Failure diagnosis and problem prediction in addition to anomaly detection
― To what can this technology be applied?
For example, at megaplants this technology can be applied to anomaly detection. Specifically, we make the features of time-series data, obtained from thousands of sensors in the plant under normal circumstances, into a database. This will enable real-time detection of anomalies when any feature that has not been observed in past normal circumstances appears.
Moreover, anomaly detection is not the only thing that this technology makes possible. If current data can be matched with accumulated past abnormality data, failure can be diagnosed based on the current problem's similarities with past cases. Previous relevant information can be instantly provided.
What's more, this technology can automatically extract features that routinely occur before an anomaly and use that information to predict future failures.
These systems perform essentially the same tasks as skilled staff. The retrieval based time-series data analysis technology performs the kind of evaluation that we, humans, have been making based on years of experience and our subconscious understanding of the systems, looking at time-series data, with higher precision by means of deep learning.
Accuracy improves as it operates―introducible right away even when data is initially limited
― How is this technology different from conventional anomaly detection systems?
Yes, NEC already has the system invariant analysis technology when it is just about anomaly detection. The system invariant analysis technology generates a model formula that represents the invariable relationship in normal-state time-series data and detects an unusual state as an anomaly when data deviating from the normal model appears. By thoroughly covering the entire system using a mass of mathematical formulae, this allows early detection of anomalies that may otherwise go unnoticed by humans or that have never been encountered in the past. These are characteristics which makes the invariant analysis technology unique and powerful.
In contrast, as mentioned earlier, the retrieval based time-series data analysis is a mechanical realization of anomaly detection based on years of experiences and subconscious understanding of seasoned staff. The combined application of the invariant analysis and model-free time-series data analysis enables the monitoring of systems from two approaches: a mathematical approach and an approach from an expert-like perspective. Furthermore, system monitoring can be made even more powerful by the additional coverage of failure diagnosis and malfunction prediction.
The retrieval based time-series data analysis also has the advantage of low-cost, quick, and smooth introduction thanks to the no-hassle of creating a normal model. A system monitoring can be started with a small accumulation of data. Generally-speaking, more than 90% of the collectible data indicates a normal state. This system enables more accurate detection of anomalies as more and more normal-state data is accumulated. That is why the technology should be able to develop a high-precision system in a few months even when data is limited in the initial introduction phase.
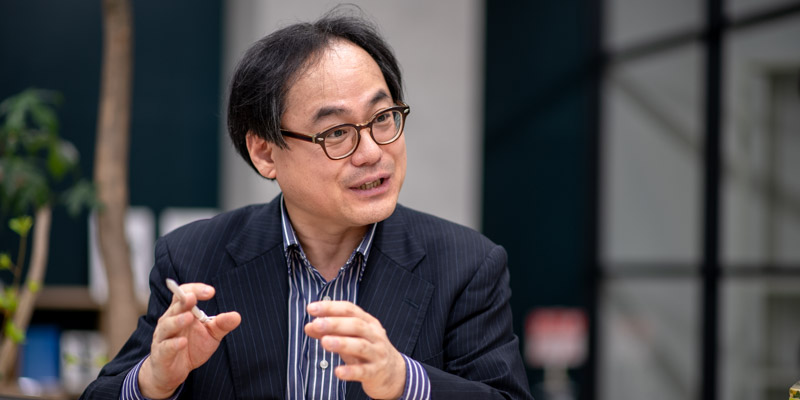
Meteorological forecasting and other possibilities beyond plant monitoring
― What other possibilities are there, other than plant applications?
As explained before, the whole point of this technology is to generate a comparable features from features of temporal data changes and the features of relationships among sensors. We believe that this technology holds a wide range of possibilities for applications beyond megaplants, data centers, and social infrastructure facilities.
While I spoke about this technology with an emphasis on the detection of anomalies from the normal state, this technology can actually derive multiple normal states, such as normal state 1, normal state 2, normal state 3, and on. The detection is not binary―normal or not normal―in this technology; in fact, it can detect and classify a diverse range of states, which opens up possibilities for needs for comparison with past conditions over time.
The model-free time-series data analysis also handles non-numerical data. As long as the data is in time-series, this approach can deal with graph data and text data, which leaves room for further potential in a wide range of fields. Meteorological forecasting by comparing meteorological data is one example. There should be more possible applications, and we seek to actively promote co-creation with different customers and partners.
- ※The information posted on this page is the information at the time of publication.