Global Site
Breadcrumb navigation
From the Frontline of the Research on Machine Learning in the US
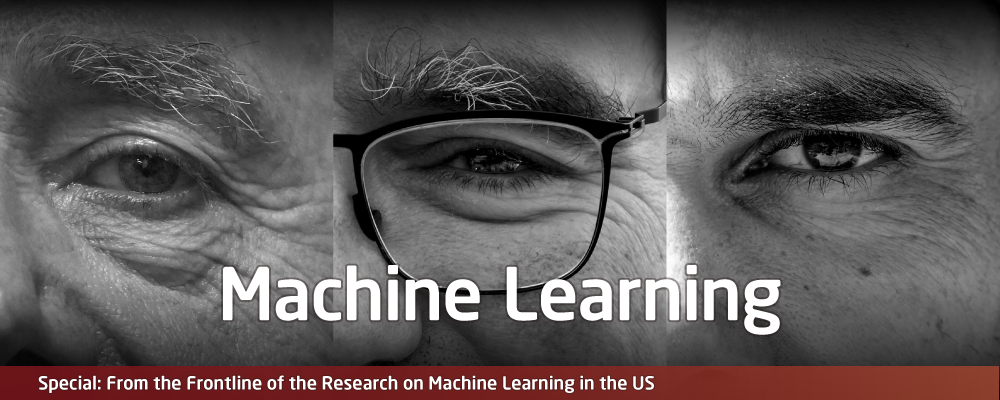
Vol.1
Hans Peter
Graf
-Groundbreaker in Machine Learning researches-
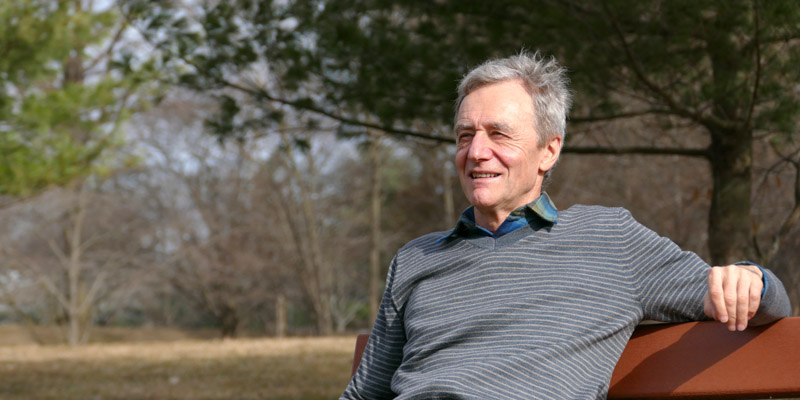
Department Head, Machine Learning
NEC Laboratories America
Hans Peter Graf
-Groundbreaker in Machine Learning researches-
NEC's AI technology has earned the industry's number one / only one reputation in various fields related to information visualization and analysis, including the world's top level image recognition technology. The driving force behind these achievements is the Machine Learning department of NEC Laboratories America (NECLA), which is located in the town of Princeton, NJ, a quiet environment surrounded by trees and close to the campus of Princeton University. It has been responsible for bridging fundamental AI research and business units of NEC.
In addition to playing a central role in the development of Torch, which is one of the main current machine learning platforms, this department constantly produces the world's top class AI researchers, working at the industry’s front lines.
In this interview, the department head Dr. Hans Peter Graf, who leads top-class researchers while pursuing advanced researches by himself, and is responsible for commercialization of research results and technology transfer to each business unit, discusses the significance and contribution of NECLA in AI research, and the future prospects.
Dr. Hans Peter Graf
Hans Peter Graf is head of the machine learning research department at NEC Laboratories America in Princeton. The department develops machine learning algorithms, as well as a range of applications in semantic text analysis, cognitive video interpretation and bio-medical analysis. His responsibilities include technology transfer to business units and the commercialization of research results. Systems for the inspection of manufactured parts, a system for trade surveillance at the Tokyo Stock Exchange and e-Pathologist, a system assisting pathologists with cancer diagnostics are some of the technologies from his department that were commercialized successfully.
Before joining NEC Laboratories, Hans Peter was in Bell Laboratories and AT&T Laboratories where he developed neural net models and machine learning applications. Massively parallel neural net processors of his design were key parts in high-speed address readers and check processing systems.
Hans Peter received a Diploma and a PhD, both in physics, from the Swiss Federal Institute of Technology in Zurich, Switzerland. He is author or coauthor of over 100 reviewed articles and some 50 issued patents. He is a Fellow of the IEEE and a member of the American Physical Society.
From Neural Network to Deep Learning
First of all, I would like to briefly review the past trends of AI research, including my own interest in AI. For me, "Making a computer which can think like the human brain" has always been a dream since a long time ago. And, about 30 years ago, when I was at Bell Laboratories, I had an opportunity to design a neural network chip that mimics the characteristics of neurons.
At that time, I thought, "If imitating the connections between neurons in the brain, we might be able to develop a new type of powerful processor." Various research teams have tackled this task over the past 30 years, building neural networks that focused not only on the firing frequency of neurons but also on the timing of spikes (action potentials). They have tried to build non-von-Neumann-type computers that can handle storing data and operation concurrently. But they only found that the efficiency of a normal processor always surpasses that of new architectures.
So I decided to switch from hardware to a software approach, focusing on the “supervised learning” method called SVM (support vector machine). This kind of machine learning turned out to be a very effective way to train AI and it was suitable for a wide range of applications.
Around 2005, the idea of neural networks was revived, but this time in software, utilizing a cascade of multiple layers of nonlinear processing units. The result led to deep leaning and since then, we have been conducting research focusing on how to develop and apply this AI technology.
Importance of Research Community and Corporate Support
Complex and difficult problems like AI cannot be solved by only one researcher. The existence of a research community and stimulating environment is important. Research institutes like Bell Laboratories and NECLA have strong communities with a commitment to conducting the highest level of research. While it attracts excellent researchers, it also gives them a big challenge because researchers always compete to develop the best ideas. These environments inspire researchers and are the driving force behind research.
The other thing I emphasize in the field of AI research is the attitude of always working on issues with the application in mind. Pursuing only theory does not make much progress in research. AI researchers have been dealing with algorithms for the past 30 years. However, there is no special power in algorithms themselves. Well, what is important is to use the algorithm for practical applications. It requires to think not only about the research side, but to let the research be driven by the application field.
It is in this respect that a research institute directly connected to industry like NECLA is best suited for AI research. In fact, academic AI researchers are often frustrated that their tasks are too abstract. Of course, such researches are also important, but the opportunity to have an impact on the real world is rare. It is important to have both research and application perspectives.
On the other hand, it takes a lot of time for AI research to produce actual results. NEC has committed to machine learning and supported researchers even before I joined NECLA. For example, in the area of digital pathology diagnosis, NEC successfully launched "e-Pathologist", a pathology image analysis system and a well known application of AI, earlier than anyone else because it has been working on it since15 years ago. Such long-term corporate support is one of NECLA's strengths.
NECLA Puts Emphasis on Solution Development Based on AI
Nowadays, you can find many corporate-based AI research departments out there. But in the case of Facebook, for example, the developed technology is basically used for its own services. In other words, there is only one output channel. Therefore, there is only one channel for communication with users, which is easy to deal with, but it limits the diversity of research.
However, in the case of NECLA, customers exist outside of NEC and in many different markets. Therefore, applications of AI range from image recognition for medical treatment to inspection of products and parts at production sites and to monitoring of transactions in stock markets. This is another reason why NECLA is attractive for researchers.
On the other hand, Facebook and Google use their own services to collect so-called big data and use it for deep learning. In terms of the size of the data collection, no other companies can beat them. Instead of following the same path, we are exploring unknown territory by adding new functionality, such as the recognition of actions.
Basic research takes time, but a sense of speed is important for solution development. So we have tried to quickly create and provide applications and systems that meet customer needs by incorporating open source codes. It is important how to organize the pipeline from research to business unit to customer that technology transfer happens quickly and smoothly. And I think the value of NECLA is the fact that we are experts in transferring break-through technology quickly to customer environments.
In the world of AI research, your rival can be both enemy and friend
One of my roles as department head is to recruit talented researchers. In AI development, project management as is common in more structured developments does not make sense. The reason is that it is difficult to accurately estimate the time until the actual results are obtained. Therefore, it is important to prepare an environment where you can put trusted researchers together and let them focus on tasks.
Of course, it takes a lot of money and time to secure good people. Moreover, just because there is no apparent result this year, it is not appropriate to fire them. That's why corporate commitment is essential. In that sense, NECLA is an ideal environment for research, and we are always looking for motivated researchers who want to pursue their own ideas. Conversely, if you need guidance and advice all the time, NECLA is not for you. We need people who have the drive to explore various possibilities by themselves.
On top of that, it is necessary to identify what kind of research actually opens the way to new markets. For this purpose, it is important to keep close relationships with external communities. Attending conferences or writing papers can be time consuming and even seem like a waste of your precious time. However, exchange of ideas with critical experts validates your ideas, and writing papers forces you to organize your thoughts clearly. Of course, as soon as you publish your paper, your rival researchers move quickly to beat you with their own ideas, but actually, that is the best way to drive you to create even better ideas.
And now, your software is exposed to similar challenges in the open source community. But, a researcher aiming for the top should know that he / she can improve himself / herself by using feedback even from “enemies”.
In addition, the difficulty of AI in business is the fact that it is not a product or solution itself but one of the features that support various solutions. What's more, every customer has different underlying data as well as different needs, so it is necessary to start with collecting data and customizing the solutions to meet the customers’ needs. These tasks are very time consuming. And I think many other IT companies are struggling with this aspect of AI solution development as well. Among them, NEC is one of the few companies that has continued to be successful in applying AI technology, and that is why NECLA attracts excellent researchers in the field of machine learning.
NECLA's contribution to the real world
Among the achievements of NECLA, the most impressive was that in 2010, e-Pathologist was selected as one of Nikkan Kogyo Shimbun's 53rd Ten Most Innovative New Product Awards. I think e-Pathologist was a system that was well ahead of the times, dealing with a very important area of cancer diagnosis. Also, in 2018, the Japan Exchange Group adopted our trade surveillance system using machine learning.
Efforts are not always rewarded, but when they lead to good results, researchers are very much satisfied with joy. In terms of commercialization of AI, I think we have achieved considerable results.
Furthermore, in terms of contributions to the open source community, Torch, a machine learning platform, has been surprisingly successful. Originally it was developed for internal use within our department, but after being open sourced, many external researchers added various extensions, and we felt like NECLA hired those people for free.
The scripting language used by Torch, called Lua, is efficient and excellent, but is not well known among the students. So Facebook has developed PyTorch that uses Python, a script language that is more popular, and PyTorch has become the most popular machine learning platform along with Google's TensorFlow. This was also a proud contribution, resulting from our continuing efforts.
Seeking the next big thing after deep learning
Regarding the future prospects, it is important to further accelerate the flow of the commercialization pipeline mentioned earlier. Also, now it is safe to say that almost all areas where deep learning can be applied are covered, and conversely its limits have become obvious. In other words, the time has come to think about new architectures. The human brain is processing much more complex information than current AI. It will be necessary to develop more advanced technology which is closer to the thinking mechanisms of our brain, and to develop techniques that can understand and reason from context. Deep learning is just pattern matching, resulting from iterative learning processes. It requires too many resources and is inefficient.
Early deep learning was simply a series of layers of neural networks, but research has recently moved to more structured models. Some researchers focus on graph structures that perform abstractions by analyzing how various entities relate, while others work on associative memories. In the latter case, access to memory is not based on explicit addressing but on the basis of similarity. Its realization requires substantial improvement in everything from data representation to storage structure to re-calling mechanisms.
In recent years, AI researchers have come into the spotlight, as the commercial value of the use of AI has increased, especially in the field of advertising. But that does not necessarily lead to a good direction for research. Research is essentially a steady process that requires constantly exploring new ideas, and the current trend may be leading too many researchers in just one direction. But at the same time, the great success of AI recently is attracting a different kind of smart people, which is an interesting development. These are two aspects of the recent trends, and as a researcher it is important to find your own research that will lead to the next big things.
Lastly, for young people, I can recommend research in the AI field to all researchers. Currently, computer scientists, engineers and technologists play main roles in AI research. However, as the application of AI has expanded, we see its influence and impact not only on businesses and manufacturing processes but also on macroeconomics and social phenomena. Issues such as privacy and data bias in job matching, for example, have also come into focus and research in these fields is not limited to computer scientists. Therefore, you may find AI related research fields interesting even if you are not a science oriented person.
In any case, I recommend young researchers to explore the world of AI, which has great potential in many areas. There is no other field that is so interesting and challenging.
(interview and text / Kazutoshi Otani)
Related Link
Vol.1
Hans Peter
Graf
-Groundbreaker in Machine Learning researches-