Global Site
Breadcrumb navigation
Manmohan Chandraker(PhD in Computer Science/Department Head, Media and Analytics/NEC Laboratories America)
September 28, 2018
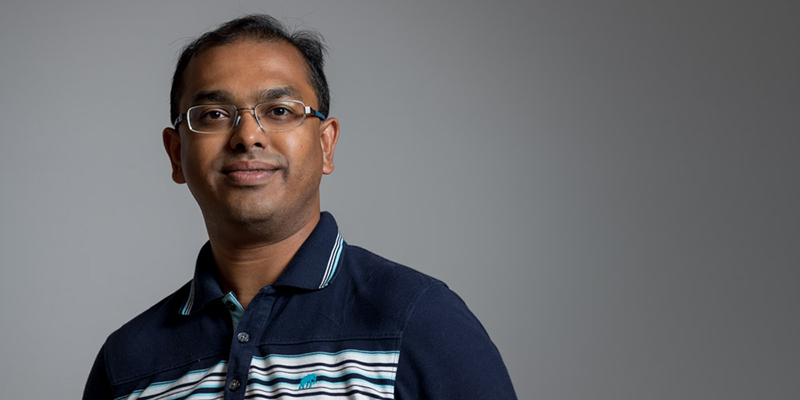
Research at Forefront: 3D Scene understanding and Visual Recognition.
Our current research topics in computer vision span two different areas, one is 3D scene understanding, and the other is visual recognition. Applications for 3D scene understanding include self-driving and augmented reality, while visual recognition can be applied to vast areas such as surveillance, retail and healthcare.
In 3D scene understanding, we take images as input and discover the 3D structure of the scene, along with interactions between its constituent elements. To recover 3D information from 2D images, we exploit cues from the geometry of image projection, knowledge of how light interacts with the scene and semantic priors that encode how the structure of the world is governed.
For visual recognition we are interested in determining how pixels can lead to more abstract information, using techniques from machine learning. Examples of information that we want to extract are recognizing who is in a picture, determining where the object of interest is, what activities are going on in the scene, trying to predict what future actions might take place in a scene, or how a scene might evolve.
Self-driving can lead to social impact such as reducing congestion, accidents and pollution to benefit us and future generations. But as social responsibility, if self-driving may cause to increase commuting time and distance, then for example, it may increase pollution as unintended consequence.
Novel Deep Learning Technologies for State-of-the-Art in Computer Vision.
NEC Labs has achieved strong advances in Domain Adaptation, to adapt a model trained in one domain to another one where no labels are available. The advent of deep learning has led to strong performances in tasks like facial recognition, we must ensure that our recognition software does not have implicit biases and performs equally well for Asians, Africans or any other ethnic group, to prevent misuse for profiling or unintended surveillance consequences. If we are deploying software that is known to have a bias towards a particular ethnic or gender category, then is our duty, it is a social responsibility to remove this bias. We have developed the first face recognition method which achieves performance on ethnicities with no labeled data at levels similar to fully supervised methods.
In the case of self-driving, NEC Labs is solving the next generation of challenges like occlusion reasoning and blind spot reasoning, or diverse future prediction, besides solving simpler tasks such as distance measurement, collision avoidance or lane keeping. With our occlusion reasoning and 3D scene understanding technologies, we can probabilistically guess what might be the semantic category in the hidden regions invisible to the driver and prevents accidents. Our future prediction can handle complex interactions between traffic participants and deal with the ambiguity of predicting multiple possible dangers given the same past history.
The key here is how do we inject insights from computer vision into the deep learning methods that we are developing. Another aspect that we are working on is interpretability. That is, we want to develop deep learning for computer vision that is not just a black box. Rather, it should allow us to make an informed decision about what is the possible reason for the black box to predict a certain outcome. Other challenges that we believe important are privacy, security or reliability of deep learning or computer vision methods.
Impact on the Research Community.
We are very active in research and professional associations. We publish regularly at the top-tier computer vision and machine learning venues such as CVPR, ICCV or NIPS. We organize workshops and tutorials on current topics at these venues to convey our message to the wider world, as well as to get insights. We also have a very strong internship program, many collaborations with universities and work with professors at various schools and their research groups on problems that are of mutual interest.
Advice for Young Researchers in Computer Vision
Computer vision right now is a very active field with new developments almost every day. I encourage young researchers to dive deeper into the core domain areas that are required to solve particular problems. We will make real impact when we figure out ways to combine our progress in black box deep learning methods, with our domain knowledge from tasks like 3D reconstruction or object recognition.
Another competence required is the ability to communicate and spread your ideas. The problems that we are solving now are big. It requires us to combine the efforts of many people and many organizations. And it's important that we are writing code that is reliable and reproducible and can be easily used by other people to achieve even bigger aims,
Next Innovations in the fields of Robotics and Augmented Reality
I believe the next level of problems in computer vision will be related to the newest type of interfaces that produce novel ways to interact with the physical world. Augmented reality will be huge since it is a new way for us to link up the physical and digital worlds. Another important area is Robotics, which is another such interface with several open challenges. Technically, we rely on large-scale labeled data now, but to solve problems when it is hard to get labels, we are also developing new methods in domain adaptation, self-supervision and learning by demonstration.
The Future of Computer Vision
Over the past five to ten years, a large amount of data has become available that allows us to solve much bigger problems than before. These strengths will continue in the future, the scale of the data will increase, computation speed and performance will keep getting better. This means that after ten years, the type of insight gained from computer vision might be fundamentally different or strengthened. Perhaps we can move beyond recognition to solving precognition problems. I can imagine a world twenty years from now when problems such as autonomous driving have been largely solved. As a result, the entire road infrastructure and the housing market might evolve and air pollution may be reduced. And these benefits might be possible because computer vision has been able to make progress.
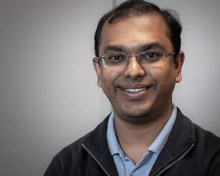
Manmohan Chandraker
Manmohan Chandraker received a B.Tech. in Electrical Engineering at the Indian Institute of Technology, Bombay and a PhD in Computer Science at the University of California, San Diego. Following a postdoctoral scholarship at the University of California, Berkeley, he joined NEC Labs America in Cupertino, where he conducts research in computer vision. His principal research interests are sparse and dense 3D reconstruction, including structure-from-motion, 3D scene understanding and dense modeling under complex illumination or material behavior, with applications to autonomous driving, robotics or human-computer interfaces. His work on provably optimal algorithms for structure and motion estimation received the Marr Prize Honorable Mention for Best Paper at ICCV 2007, the 2009 CSE Dissertation Award for Best Thesis at UC San Diego and was a nominee for the 2010 ACM Dissertation Award. His work on shape recovery from motion cues for complex material and illumination received the Best Paper Award at CVPR 2014.
Relevant Laboratories