Global Site
Breadcrumb navigation
NEC technology greatly simplifies the process of registering new objects with AI-based image recognition
~ Reduce working-hours required to create training data with "weakly supervised learning" that utilizes ambiguous information ~Tokyo, May 30, 2022 - NEC Corporation (NEC; TSE: 6701), in a joint effort with RIKEN, Japan’s largest and most comprehensive research organization for basic and applied science, has developed a technology that greatly simplifies the work of preparing training data, which is required when registering new objects with AI-based image recognition (Note 1). This development is expected to facilitate the application of AI across a wide range of fields.
In recent years, AI-based image recognition technology has been applied to a variety of businesses, including construction and manufacturing. As it has been increasingly adopted, the cost of preparing training data has become a major issue. For example, if one tries to adapt an image recognition system deployed in one construction site or factory to another, it is often necessary to continually register new objects. In such cases, the preparation of training data has been a major burden on users.
NEC and RIKEN have now developed a breakthrough algorithm that utilizes ambiguously labeled data, which can be collected with significantly lower costs than clean, fully annotated data. This new technology is based on a paradigm called "weakly supervised learning." The latter also uses ambiguous data, but the conventional algorithms have suffered from instability of training, which results in low accuracy. The newly developed algorithm successfully stabilizes weakly supervised learning and trains accurate models (Note 2). By using this technology, the time required to create training data has been confirmed to be reduced by 75% when building an image recognition engine that detects 80 types of objects (Note 3).
Generally, when an object is newly registered with an image recognition engine, the time required for labeling can be greatly reduced if a model can be trained with only data labeled on the new detection object. Such training data may have ambiguous "weak labels." "Weakly supervised learning" can leverage such ambiguously labeled training data, while AI algorithms usually assume training data to be clean and precisely labeled. For example, consider a scenario with an AI engine that detects cars, trucks and buses, then newly register the object "motorcycle" with the engine. If we collect training data with only motorcycles labeled, the regions without any labels can be seen as labeled "not a motorcycle," which ambiguously indicates that there is a car, a truck, a bus, or nothing. The weakly supervised learning makes it possible to learn only from such ambiguous data, which leads to a great reduction in the working-hours required to create data.
Fully supervised learning
Label all objects
Weakly supervised learning
Label only new objects
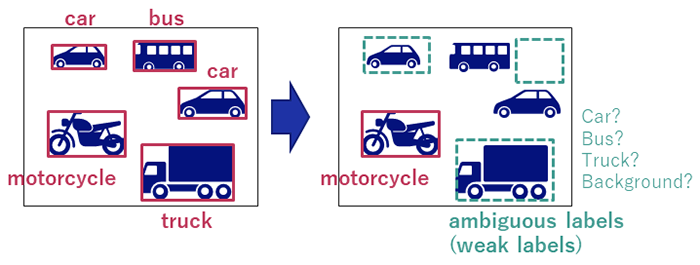
The position and type of all
objects are known
Unlabeled areas are only known
to be "not motorcycles"
Mechanism for adding a motorcycle as a new detection target
When trained with fully labeled data, the model should be optimized so that the output values are close to the correct labels. In contrast, weakly supervised learning optimizes the model while sequentially estimating the correctness of model predictions based on weak labels. However, with this method, optimization becomes unstable due to label ambiguity, and it has not been possible to train a highly accurate model.
Fully supervised learning | Weakly supervised learning | ||
Conventional methods | Newly developed method | ||
Learning data creation |
Large amounts of work Difficult to achieve |
Less work | Less work |
Learning stability | レ | × | レ |
Comparison to conventional technologies
Our new technology solves these issues by regularizing training in order to eliminate instability. Existing methods also apply ad hoc regularization to stabilize training, but there is no guarantee of "training a model that correctly classifies objects." However, NEC and RIKEN have now developed an algorithm that simultaneously stabilizes weakly supervised learning and trains accurate models.
This makes it possible to train highly accurate models, even from weakly labeled data.
This technology was announced at the International Conference on Machine Learning (ICML) 2021, which is well-known in the fields of machine learning and artificial intelligence. (Note 4)
***
- (Note 1)This technology was developed by the Riken AIP-NEC Collaboration Center (April 1, 2017 to March 31, 2022), which was established as a joint research institution between Riken and NEC.
- (Note 2)NEC research
- (Note 3)Estimated assuming that a new detection target will be added to the model based on the public dataset MS COCO for object detection. Specifically, one of the 80 classes of MS COCO was regarded as a new object, and the reduction rate of the number of labels was calculated.
- (Note 4)Shuhei M. Yoshida, Takashi Takenouchi, Masashi Sugiyama. Lower-Bounded Proper Losses for Weakly Supervised Classification.
http://proceedings.mlr.press/v139/yoshida21a.html
About NEC Corporation
NEC Corporation has established itself as a leader in the integration of IT and network technologies while promoting the brand statement of “Orchestrating a brighter world.” NEC enables businesses and communities to adapt to rapid changes taking place in both society and the market as it provides for the social values of safety, security, fairness and efficiency to promote a more sustainable world where everyone has the chance to reach their full potential. For more information, visit NEC at https://www.nec.com.

LinkedIn: https://www.linkedin.com/company/nec/
YouTube: https://www.youtube.com/user/NECglobalOfficial
Facebook: https://www.facebook.com/nec.global/
Twitter: https://twitter.com/NEC_corp
NEC is a registered trademark of NEC Corporation. All Rights Reserved. Other product or service marks mentioned herein are the trademarks of their respective owners. © NEC Corporation.