Global Site
Breadcrumb navigation
Atsushi Sato(PhD in Physics/Research Fellow/NEC Biometrics Research Laboratories)
September 28, 2018
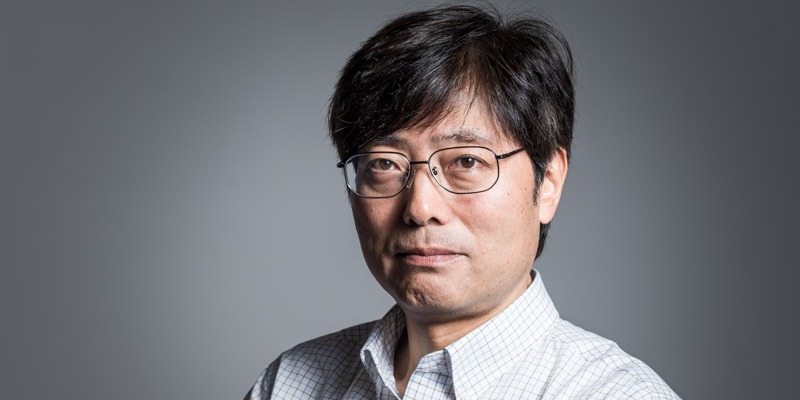
Developing GLVQ: NEC's proprietary technology that pioneered machine learning
I have previously worked on research specializing in pattern recognition, image processing, and machine learning, but when I started out at NEC, my research subject was the development of character recognition techniques for mail sorting systems. The recognition unit of the systems back then was hardware, and we needed to optimize the standard pattern designs and revise the learning approach in order to improve performance. I majored in physics in university, so the field of character recognition was outside of my specialty. I recall having lots of things I didn't understand at the time and that research was not an easy quest at first. However, I kept my motivation high while working on the development of the Generalized Learning Vector Quantization (GLVQ), which successfully improved our recognition performance significantly. The representative of the then Ministry of Posts and Telecommunications, to whom we delivered this system, even endorsed NEC's system as being the best. Mail sorting systems based on the GLVQ have been introduced in 50 countries as of today thanks to the other members developing overseas algorithms, and are contributing to streamlined postal distribution internationally. The GLVQ has also become the foundation for NEC's illustrious face recognition technology.
We are now advancing this learning approach and image processing technology in our current research of capturing people's movements from camera images. NEC is operating public safety business worldwide. Progress in this technological research can lead to further assurance of safety and security due to earlier detection of dangerous behavior from network security cameras at airports and stations. I also expect our developments to be applicable to useful solutions to our daily lives, such as streamlining venue operation by controlling human traffic, in addition to the aforementioned monitoring purposes.
Machine learning with small data
The world is seeing a boom in artificial intelligence, bolstered by the progress in deep learning and machine learning technologies. The speed of technological evolution is dramatic, making it difficult to make any predictions for 10 years ahead. However, we can say for sure that in the future not so far away, artificial intelligence will take over most of human's intellectual tasks and bring about a more comfortable and efficient society.
Still, we have the problem of real-world data collection as our bottleneck for improving the performance of artificial intelligence. To solve real-life problems, we need immense amounts of data for learning, but collecting necessary data from the real world for solving problems is extremely difficult. To this end, we are currently collaborating with RIKEN AIP (Advanced Integrated Intelligence Platform Project) to tackle the challenging question of how we can improve performance with limited data. How to complement missing information with completely different information will be key to this problem. We hope to further deepen this technological research and create image recognition and pattern recognition technologies that can be fully applicable to practical use in society.
The most crucial quality for a researcher is a zeal to explore the essence of problems
Regretfully, Japan is said to be far behind the U.S. and China regarding the research into machine learning. Therefore, cultivating excellent researchers is one of the greatest issues we are currently facing. To play a part in this challenge, I also teach students at the University of Tokyo as a visiting professor.
What I would like to expect from next-generation researchers is the willingness to explore the essence of the problem. In the study of physics, researchers are challenged to approach the essence of the problem. The question is: How to approach the essence of the issues and work on drawing out formulations that lead to solutions. I hope that new researchers are aggressive in taking on such challenges. The bottom line is the motivation to solve the problem. I treasure the motivation to create something new and different from other people the most. I expect such strong motivations from young and coming researchers.
- ※The information posted on this page is the information at the time of publication.